Improvement of low-rank representation denoising model based on nonconvex penalty function
Proceedings of the 4th International Conference on Communication and Information Processing(2018)
摘要
Because nuclear norm tends to underestimate the nonzero singular values in low-rank representation model (LRR), a parameterized nonconvex penalty function is used to estimate non-zero singular value, which can recover the low-rank matrix more accurately. For image denoising, using the inherent non-local self-similarity of the image, the improved model can more effectively detect and remove noise and improve quality of the recovered image. In order to solve the proposed model, an alternative minimisation method has been adopted. Experimental results indicate that the proposed algorithm achieves a competitive denoising performance, especially for high-intensity noise, in comparison with state-of-the-art algorithms in terms of subjective and objective qualities.
更多查看译文
关键词
image denoising, low-rank representation, non-local self-similarity, nonconvex penalty function
AI 理解论文
溯源树
样例
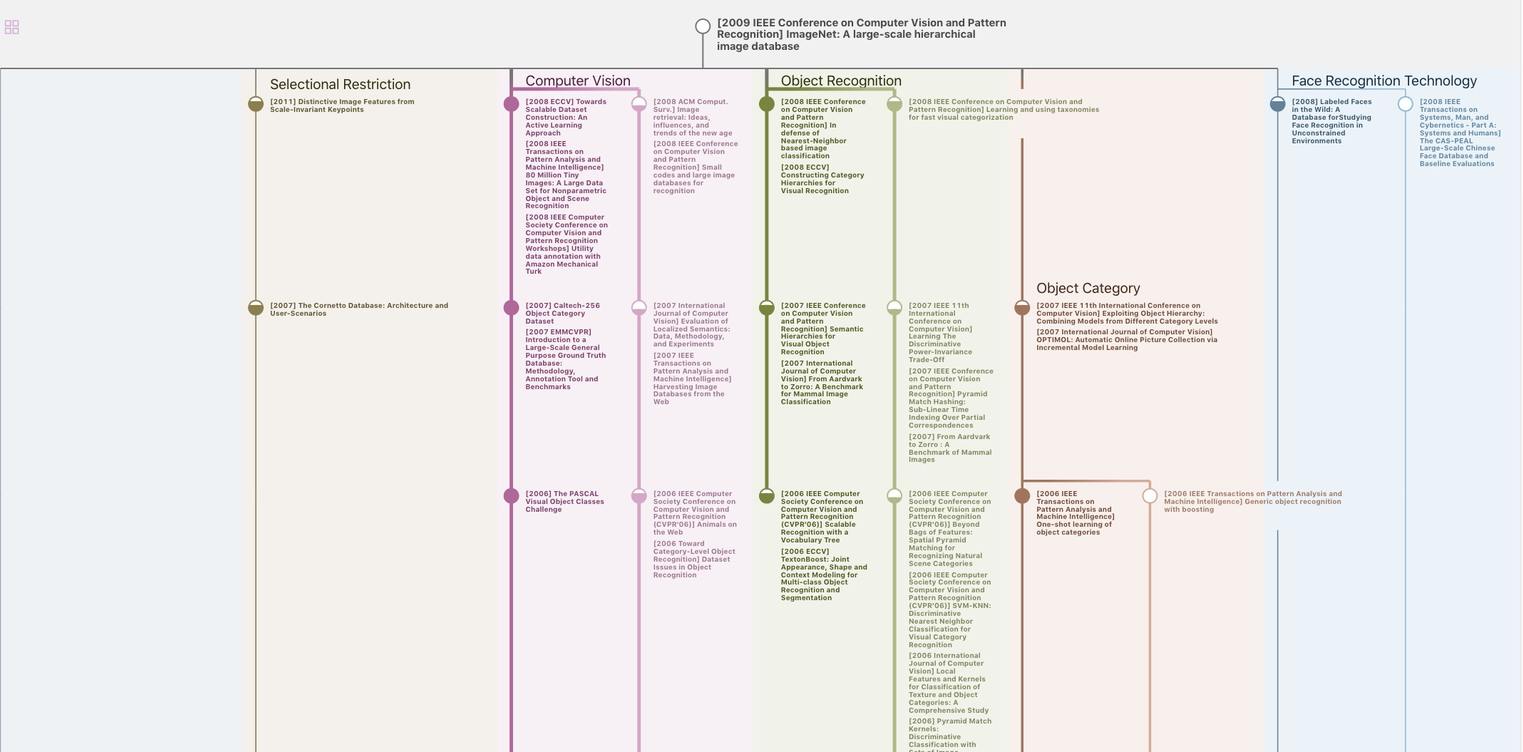
生成溯源树,研究论文发展脉络
Chat Paper
正在生成论文摘要