PointNet Evaluation for On-Road Object Detection Using a Multi-resolution Conditioning.
CIARP(2018)
Abstract
On-road object detection is one of the main topics in the development of autonomous vehicles. Factors related to the diversity of classes, pose changes, occlusions, and low resolution make object detection challenging. Most of the object detection techniques which have been based on RGB images, have limitations because of the influence of environmental lighting conditions. Consequently, other sources of information have become interesting for undertaking this task. This paper proposes an on-road object detection method, which uses 3D information acquired by a LiDAR HD sensor. We evaluate a neural network architecture based on PointNet for multi-resolution 3D objects. To carry this out, a multi-resolution conditioning stage is proposed in order to optimize the performance of the PointNet architecture applied over LiDAR data. Both the training and evaluation processes are performed by using the KITTI dataset. Our approach uses low computational cost algorithms, which are based on occupancy grid maps for on-road object segmentation. The experiments show that the proposed method achieves better results than PointNet evaluated on a single resolution.
MoreTranslated text
Key words
Pedestrian detection, LiDAR, Deep learning, Resolution conditioning
AI Read Science
Must-Reading Tree
Example
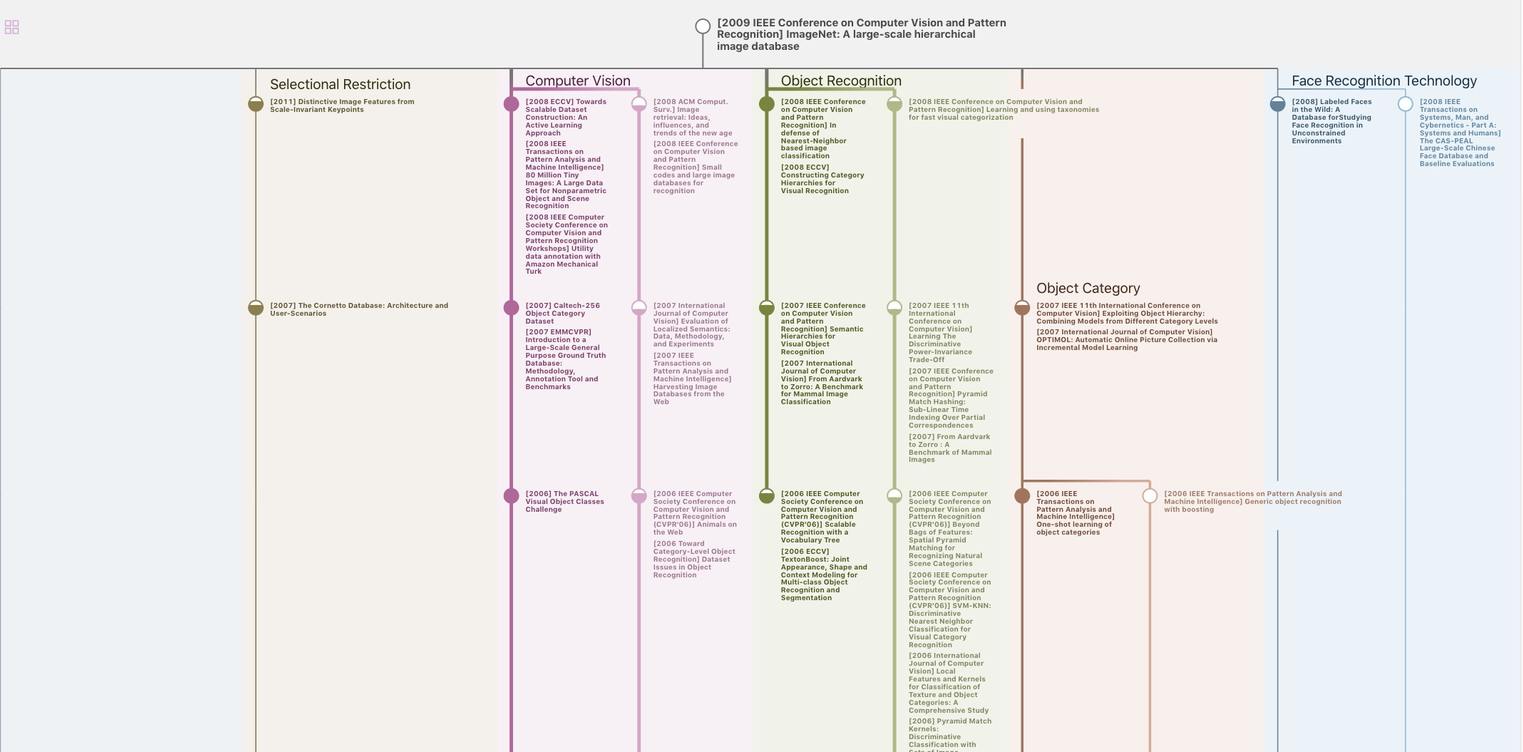
Generate MRT to find the research sequence of this paper
Chat Paper
Summary is being generated by the instructions you defined