Volumetric Instance-Aware Semantic Mapping And 3d Object Discovery
IEEE ROBOTICS AND AUTOMATION LETTERS(2019)
摘要
To autonomously navigate and plan interactions in real-world environments, robots require the ability to robustly perceive and map complex, unstructured surrounding scenes. Besides building an internal representation of the observed scene geometry, the key insight toward a truly functional understanding of the environment is the usage of higher level entities during mapping, such as individual object instances. This work presents an approach to incrementally build volumetric object-centric maps during online scanning with a localized RGB-D camera. First, a per-frame segmentation scheme combines an unsupervised geometric approach with instance-aware semantic predictions to detect both recognized scene elements as well as previously unseen objects. Next, a data association step tracks the predicted instances across the different frames. Finally, a map integration strategy fuses information about their 3D shape, location, and, if available, semantic class into a global volume. Evaluation on a publicly available dataset shows that the proposed approach for building instance-level semantic maps is competitive with state-of-theart methods, while additionally able to discover objects of unseen categories. The system is further evaluated within a real-world robotic mapping setup, for which qualitative results highlight the online nature of the method. Code is available at https://githuh.com/ ethz-asl/voxblox-plusplus.
更多查看译文
关键词
RGB-D perception, object detection, segmentation and categorization, mapping
AI 理解论文
溯源树
样例
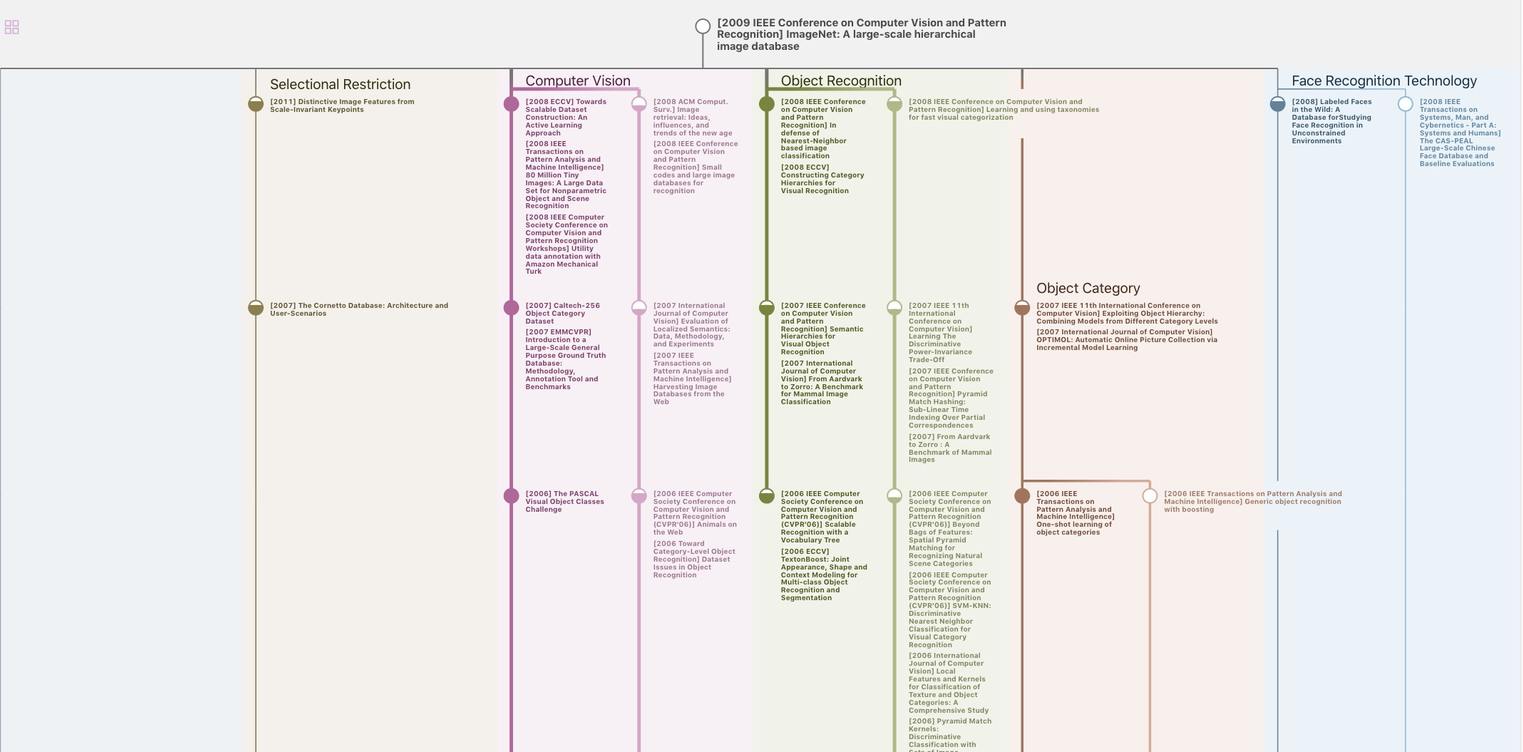
生成溯源树,研究论文发展脉络
Chat Paper
正在生成论文摘要