Bayesian inference for intratumour heterogeneity in mutations and copy number variation
JOURNAL OF THE ROYAL STATISTICAL SOCIETY SERIES C-APPLIED STATISTICS(2016)
摘要
Tissue samples from the same tumour are heterogeneous. They consist of different subclones that can be characterized by differences in DNA nucleotide sequences and copy numbers on multiple loci. Inference on tumour heterogeneity thus involves the identification of the subclonal copy number and single-nucleotide mutations at a selected set of loci. We carry out such inference on the basis of a Bayesian feature allocation model. We jointly model subclonal copy numbers and the corresponding allele sequences for the same loci, using three random matrices, L, Z and w, to represent subclonal copy numbers (L), the number of subclonal variant alleles (Z) and the cellular fractions (w) of subclones in one or more tumour samples respectively. The unknown number of subclones implies a random number of columns. More than one subclone indicates tumour heterogeneity. Using simulation studies and a real data analysis with next generation sequencing data, we demonstrate how posterior inference on the subclonal structure is enhanced with the joint modelling of both structure and sequencing variants on subclonal genomes. An R package is available from http://cran.r-project.org/web/packages/BayClone2/index.html.
更多查看译文
关键词
Categorical Indian buffet process,Feature allocation models,Markov chain Monte Carlo methods,Next generation sequencing,Random matrices,Subclone,Variant calling
AI 理解论文
溯源树
样例
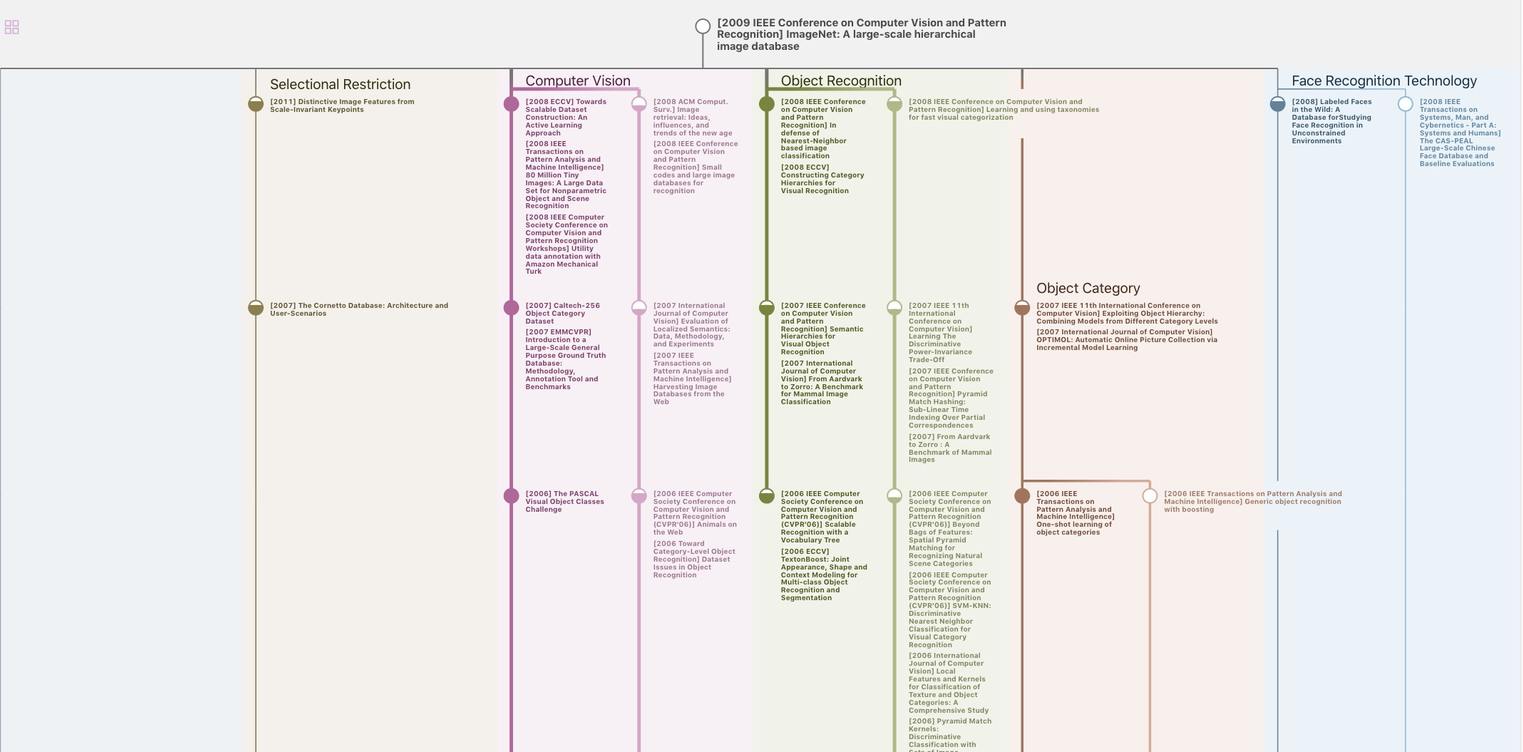
生成溯源树,研究论文发展脉络
Chat Paper
正在生成论文摘要