Application of data mining in fault diagnosis of induction motor
2016 IEEE First International Conference on Control, Measurement and Instrumentation (CMI)(2016)
Abstract
Data driven approaches are gaining popularity in the field of condition monitoring due to their knowledge based fault identification capability for wide range of motor operation. Particularly the method, based on mining the data can encompass the wide behavioral operation of induction motor drive system in industries. Therefore, appropriate low cost instrumentation embedding an efficient algorithm becomes the industrial demand for fault diagnosis of induction motor drive. A hardware friendly algorithm for multi-class fault diagnosis by applying data mining technique is proposed in this paper. Most frequently associated faults like bearing fault, stator inter-turn fault, broken rotor bar fault are investigated for a drive fed induction motor. Discrete wavelet transform-Inverse discrete wavelet transform (DWT-IDWT) algorithm is used to obtain the unique characteristics from each synthesized sub-band and these filtered signals are exploited for feature extraction. A feature selection technique based on Genetic Algorithm (GA) is utilized to identify the potential features for reducing the dimensionality of the feature space. The use of smallest length filter of 2 coefficients (db1) for DWT-IDWT algorithm and 6 relevant features has made the proposed algorithm computationally efficient. The classification accuracy for the investigated multiple faults are found to be quite appreciable. Further, a comparative study is also done using different classifiers: k-NN, MLP and RBF.
MoreTranslated text
Key words
induction motor drive,bearing fault,stator inter-turn fault,DWT,GA
AI Read Science
Must-Reading Tree
Example
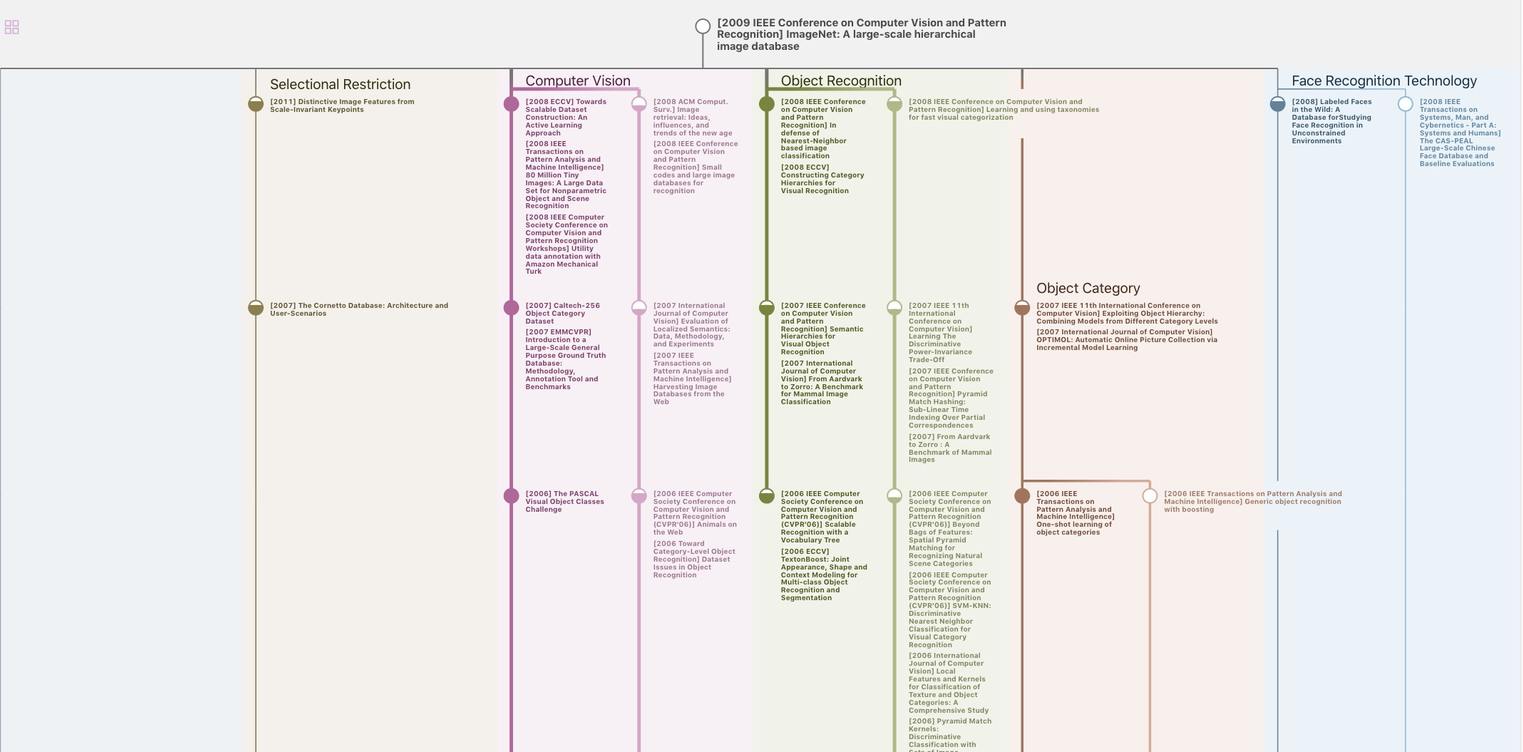
Generate MRT to find the research sequence of this paper
Chat Paper
Summary is being generated by the instructions you defined