Polar Decoding on Sparse Graphs with Deep Learning
2018 CONFERENCE RECORD OF 52ND ASILOMAR CONFERENCE ON SIGNALS, SYSTEMS, AND COMPUTERS(2018)
摘要
In this paper, we present a sparse neural network decoder (SNND) of polar codes based on belief propagation (BP) and deep learning. At first, the conventional factor graph of polar BP decoding is converted to the bipartite Tanner graph similar to low-density parity-check (LDPC) codes. Then the Tanner graph is unfolded and translated into the graphical representation of deep neural network (DNN). The complex sum-product algorithm (SPA) is modified to min-sum (MS) approximation with low complexity. We dramatically reduce the number of weight by using single weight to parameterize the networks. Optimized by the training techniques of deep learning, proposed SNND achieves comparative decoding performance of SPA and obtains about $0.5$ dB gain over MS decoding on ($128,64$) and ($256,128$) codes. Moreover, $60 \%$ complexity reduction is achieved and the decoding latency is significantly lower than the conventional polar BP.
更多查看译文
关键词
Polar codes,belief propagation,deep learning,neural networks,sparse graphs
AI 理解论文
溯源树
样例
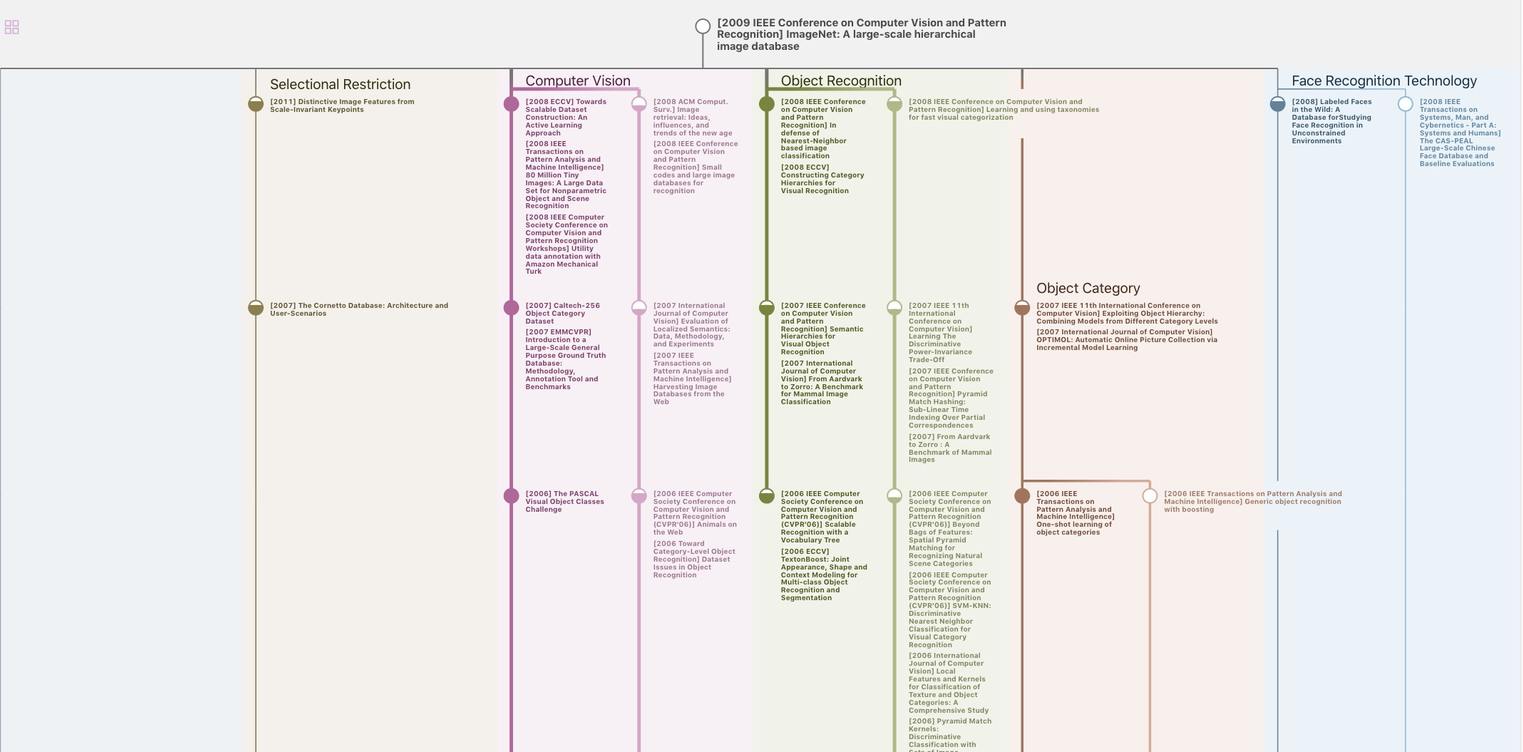
生成溯源树,研究论文发展脉络
Chat Paper
正在生成论文摘要