A Performance Evaluation Of Several Deep Neural Networks For Reverberant Speech Separation
2018 CONFERENCE RECORD OF 52ND ASILOMAR CONFERENCE ON SIGNALS, SYSTEMS, AND COMPUTERS(2018)
摘要
In this paper, we compare different deep neural networks (DNN) in extracting speech signals from competing speakers in room environments, including the conventional fully-connected multilayer perception (MLP) network, convolutional neural network (CNN), recurrent neural network (RNN), and the recently proposed capsule network (CapsNet). Each DNN takes input of both spectral features and converted spatial features that are robust to position mismatch, and outputs the separation mask for target source estimation. In addition, a psychacoustically-motivated objective function is integrated in each DNN, which explores perceptual importance of each IT unit in the training process. Objective evaluations are performed on the separated sounds using the converged models, in terms of PESQ, SDK as well as STOI. Overall, all the implemented DNNs have greatly improved the quality and speech intelligibility of the embedded target source as compared to the original recordings. In particular, bidirectional RNN, either along the temporal direction or along the frequency bins, outperforms the other DNN structures with consistent improvement.
更多查看译文
关键词
reverberant speech separation,speech signals,multilayer perception network,recurrent neural network,spectral features,separation mask,target source estimation,speech intelligibility,DNN structures,deep neural networks,capsule network,room environments,convolutional neural network,psychacoustically-motivated objective function,embedded target source
AI 理解论文
溯源树
样例
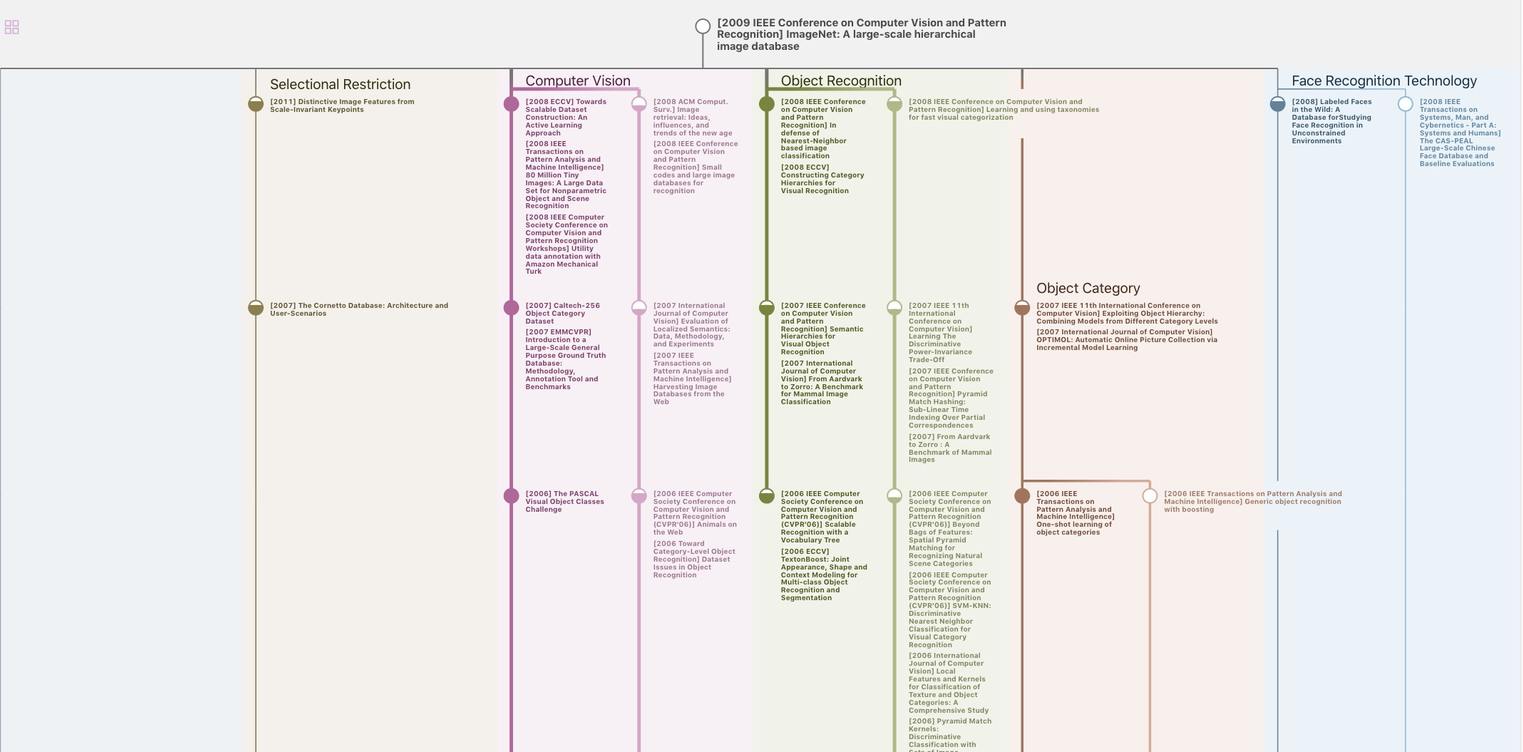
生成溯源树,研究论文发展脉络
Chat Paper
正在生成论文摘要