Label propagation algorithm based on nonnegative sparse representation
Dalian Ligong Daxue Xuebao/Journal of Dalian University of Technology(2012)
Abstract
Graph-based semi-supervised learning strategy plays an important role in the semi-supervised learning area. This paper presents a novel label propagation algorithm based on nonnegative sparse representation (NSR) for bioinformatics and biometrics. Firstly, we construct a sparse probability graph (SPG) whose nonnegative weight coefficients are derived by nonnegative sparse representation algorithm. The weights of SPG naturally reveal the clustering relationship of labeled and unlabeled samples; meanwhile automatically select appropriate adjacency structure as compared to traditional semi-supervised learning algorithm. Then the labels of unlabeled samples are propagated until algorithm converges. Extensive experimental results on biometrics, UCI machine learning and TDT2 text datasets demonstrate that label propagation algorithm based on NSR outperforms the standard label propagation algorithm.
MoreTranslated text
Key words
Clustering relationship,Label propagation,Nonnegative sparse representation,Semi-supervised learning,Sparse probability graph
AI Read Science
Must-Reading Tree
Example
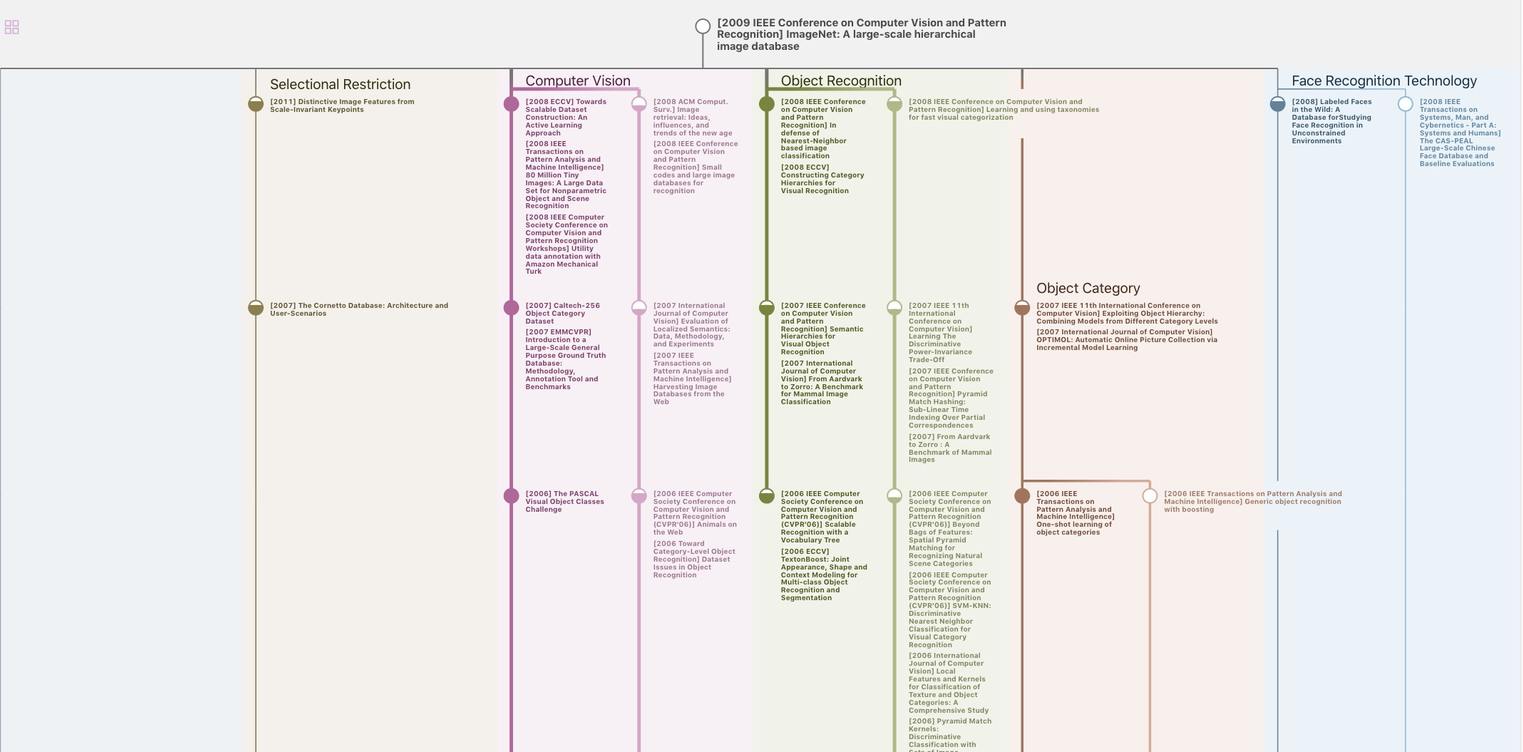
Generate MRT to find the research sequence of this paper
Chat Paper
Summary is being generated by the instructions you defined