Machine Learning for Early HARQ Feedback Prediction in 5G
2018 IEEE GLOBECOM WORKSHOPS (GC WKSHPS)(2018)
摘要
We put forward Machine Learning methods to predict the decodability of a received message before the end of the actual transmission in an Early Hybrid Automatic Repeat reQuest (E-HARQ) feedback scheme. Here we focus on a single retransmission setting for ultra-reliable and low-latency communication (URLLC) and demonstrate how more elaborate classification approaches can significantly improve the effective bit error rate towards the URLLC requirement of 10
-5
with only small latency overhead. We stress the importance of a careful determination of the classifier's working point and discuss appropriate ways of discriminating between different classifiers. We demonstrate the feasibility of our procedure for different signal-to-noise ratios as well as subcode lengths and discuss practical implications of our findings.
更多查看译文
关键词
Communication systems,ultra-reliable and low-latency communication,physical layer,Hybrid Automatic Repeat reQuest,Machine Learning,imbalanced classification
AI 理解论文
溯源树
样例
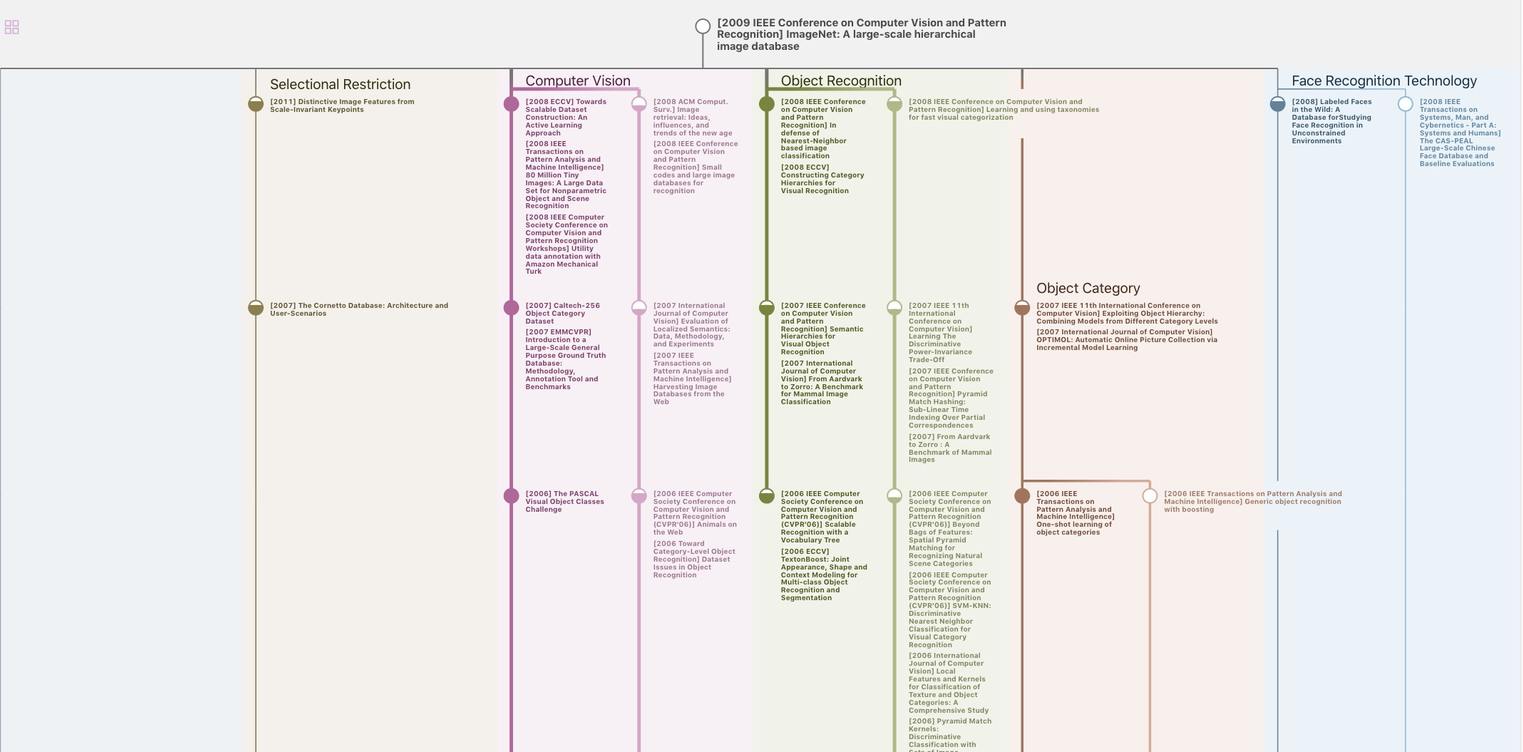
生成溯源树,研究论文发展脉络
Chat Paper
正在生成论文摘要