A statistical framework for cross-tissue transcriptome-wide association analysis
NATURE GENETICS(2019)
摘要
Transcriptome-wide association analysis is a powerful approach to studying the genetic architecture of complex traits. A key component of this approach is to build a model to impute gene expression levels from genotypes by using samples with matched genotypes and gene expression data in a given tissue. However, it is challenging to develop robust and accurate imputation models with a limited sample size for any single tissue. Here, we first introduce a multi-task learning method to jointly impute gene expression in 44 human tissues. Compared with single-tissue methods, our approach achieved an average of 39% improvement in imputation accuracy and generated effective imputation models for an average of 120% more genes. We describe a summary-statistic-based testing framework that combines multiple single-tissue associations into a powerful metric to quantify the overall gene–trait association. We applied our method, called UTMOST (unified test for molecular signatures), to multiple genome-wide-association results and demonstrate its advantages over single-tissue strategies.
更多查看译文
关键词
GWAS,gene-level association test,gene expression imputation,multi-tissue analysis,late-onset Alzheimer’,s disease
AI 理解论文
溯源树
样例
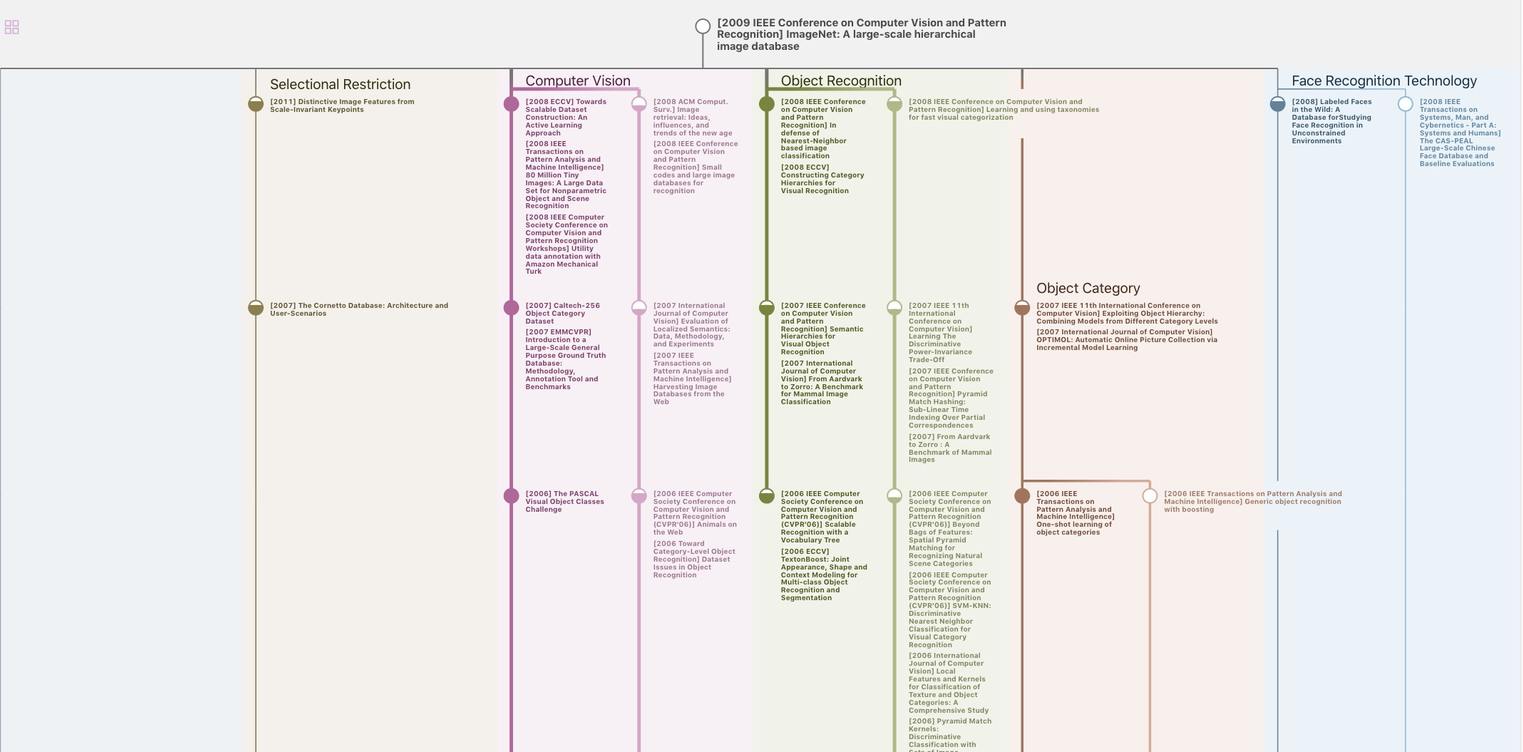
生成溯源树,研究论文发展脉络
Chat Paper
正在生成论文摘要