Magnetic Domain Wall Neuron With Lateral Inhibition
JOURNAL OF APPLIED PHYSICS(2018)
Abstract
The development of an efficient neuromorphic computing system requires the use of nanodevices that intrinsically emulate the biological behavior of neurons and synapses. While numerous artificial synapses have been shown to store weights in a manner analogous to biological synapses, the challenge of developing an artificial neuron is impeded by the necessity to include leaking, integrating, firing, and lateral inhibition features. In particular, previous proposals for artificial neurons have required the use of external circuits to perform lateral inhibition, thereby decreasing the efficiency of the resulting neuromorphic computing system. This work therefore proposes a leaky integrate-andfire neuron that intrinsically provides lateral inhibition, without requiring any additional circuitry. The proposed neuron is based on the previously proposed domain-wall magnetic tunnel junction devices, which have been proposed as artificial synapses and experimentally demonstrated for nonvolatile logic. Single-neuron micromagnetic simulations are provided that demonstrate the ability of this neuron to implement the required leaking, integrating, and firing. These simulations are then extended to pairs of adjacent neurons to demonstrate, for the first time, lateral inhibition between neighboring artificial neurons. Finally, this intrinsic lateral inhibition is applied to a ten-neuron crossbar structure and trained to identify handwritten digits and shown via direct large-scale micromagnetic simulation for 100 digits to correctly identify the proper signal for 94% of the digits. Published by AIP Publishing.
MoreTranslated text
Key words
magnetic domain wall neuron,domain wall,lateral inhibition
AI Read Science
Must-Reading Tree
Example
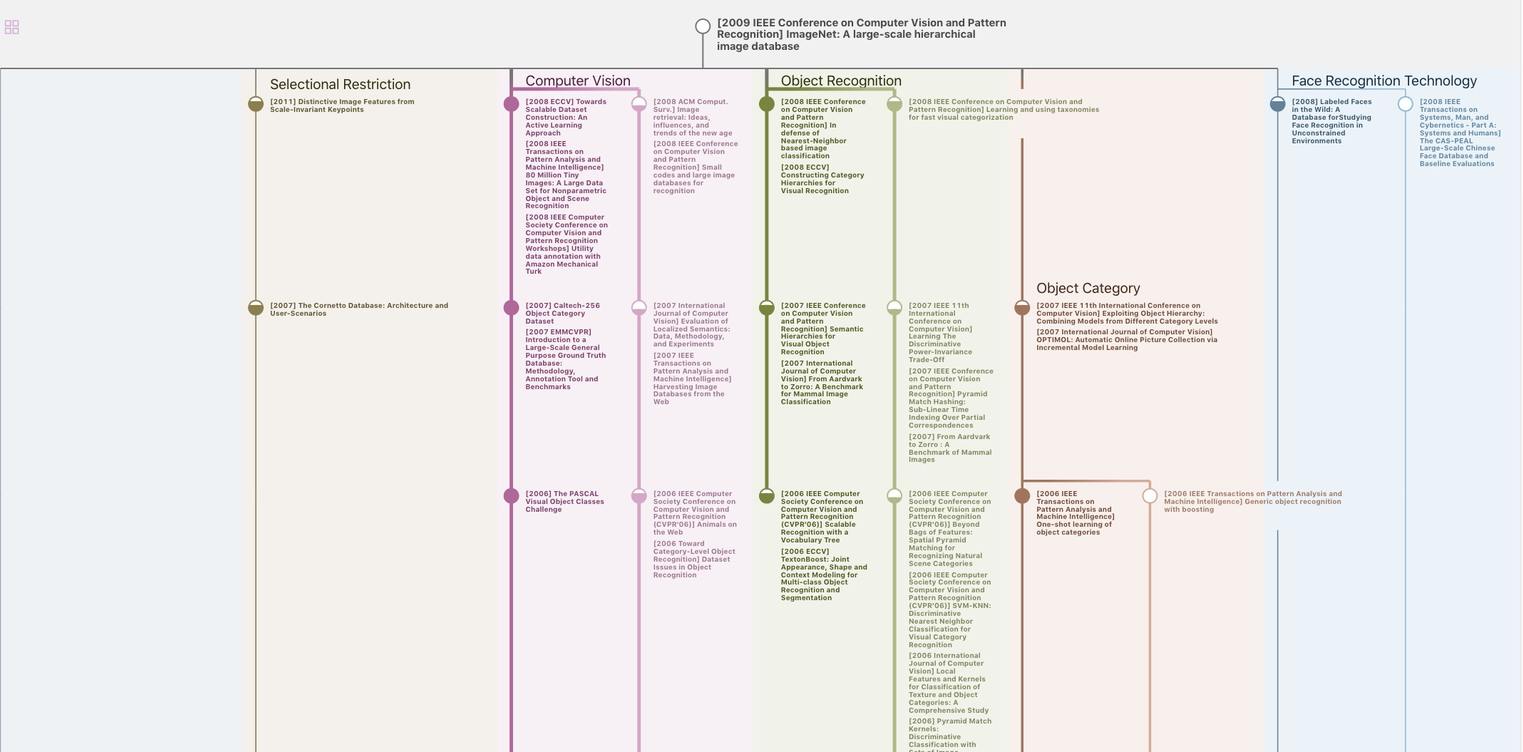
Generate MRT to find the research sequence of this paper
Chat Paper
Summary is being generated by the instructions you defined