Mri-Based Synthetic Ct For Radiation Treatment Of Prostate Cancer
INTERNATIONAL JOURNAL OF RADIATION ONCOLOGY BIOLOGY PHYSICS(2018)
Abstract
The purpose of this work is to develop a learning-based method to generate synthetic CT from routine anatomical MRI for MRI-only radiation treatment of prostate cancer. We propose to integrate auto-context model and patch-based anatomical signature into machine learning framework to effectively capture the relationship between the CT and MR images for CT synthesis. The proposed prediction of CT images consists of two major stages: the training stage and the prediction stage. During the training stage, patch-based anatomical features are extracted from the aligned MRI-CT training images, and the most informative features are identified to train a random forest based on auto-context model. During the prediction stage, we extract the selected features from the new MRI and feed them into the well-trained forests for the iterative CT prediction. Our predicted CT images generated from MRI were registered to original (planning) CT images for generating a synthetic CT-based treatment plan. Mean absolute error (MAE) and normalized cross-correlation (NCC) were used to quantify the differences between the synthetic and original CT images. Clinically relevant dose volume histogram (DVH) metrics were extracted from original CT-based and synthetic CT-based treatment plans for quantitative dosimetric evaluation. Gamma analysis was performed for the comparison of absorbed dose distributions between the both plans of each patient. This CT prediction method was tested using 22 prostate patients with 34 treatment plans (mean prescription dose: 48.79±15.20 Gy), each of whom has planning CT and MRI images. The mean MAE and NCC between MRI-based synthetic CT and planning CT were 29.86±10.04HU and 0.98±0.03 for all patients’ data. Mean dose differences between PTVs on synthetic and planning CT images across all the plans were -0.23±0.36%, -0.12±0.14%, -0.13±0.13%, -0.14±0.14%, -0.13±0.14%, and -0.15±0.24% for Dmin, D10, D50, D95, Dmean and Dmax, respectively. Mean dose differences between bladder, rectum, femur head and penile bulb on both CT images ranged from -0.25% to 0.20% for all DVH metrics. No significant differences were observed in the DVH metrics of the PTV and OARs between synthetic and planning CT-based plans (p > 0.05). The average pass rate of gamma analysis was over 99% with 3%/3 mm (dose difference/distance to agreement) acceptance criteria in every plan. We have investigated a novel learning-based approach to generate CT images from routine MR images for MRI-based prostate cancer radiotherapy. We have demonstrated that the reported method is capable of reliably generating synthetic CT images from the MR images and providing dose calculation of comparable accuracy to the standard CT for prostate cancer treatment planning. The image similarity and dosimetric agreement between synthetic and planning CT warrants further development of a MRI-only workflow for radiotherapy of prostate cancer.
MoreTranslated text
Key words
synthetic ct,prostate,radiation treatment,mri-based
AI Read Science
Must-Reading Tree
Example
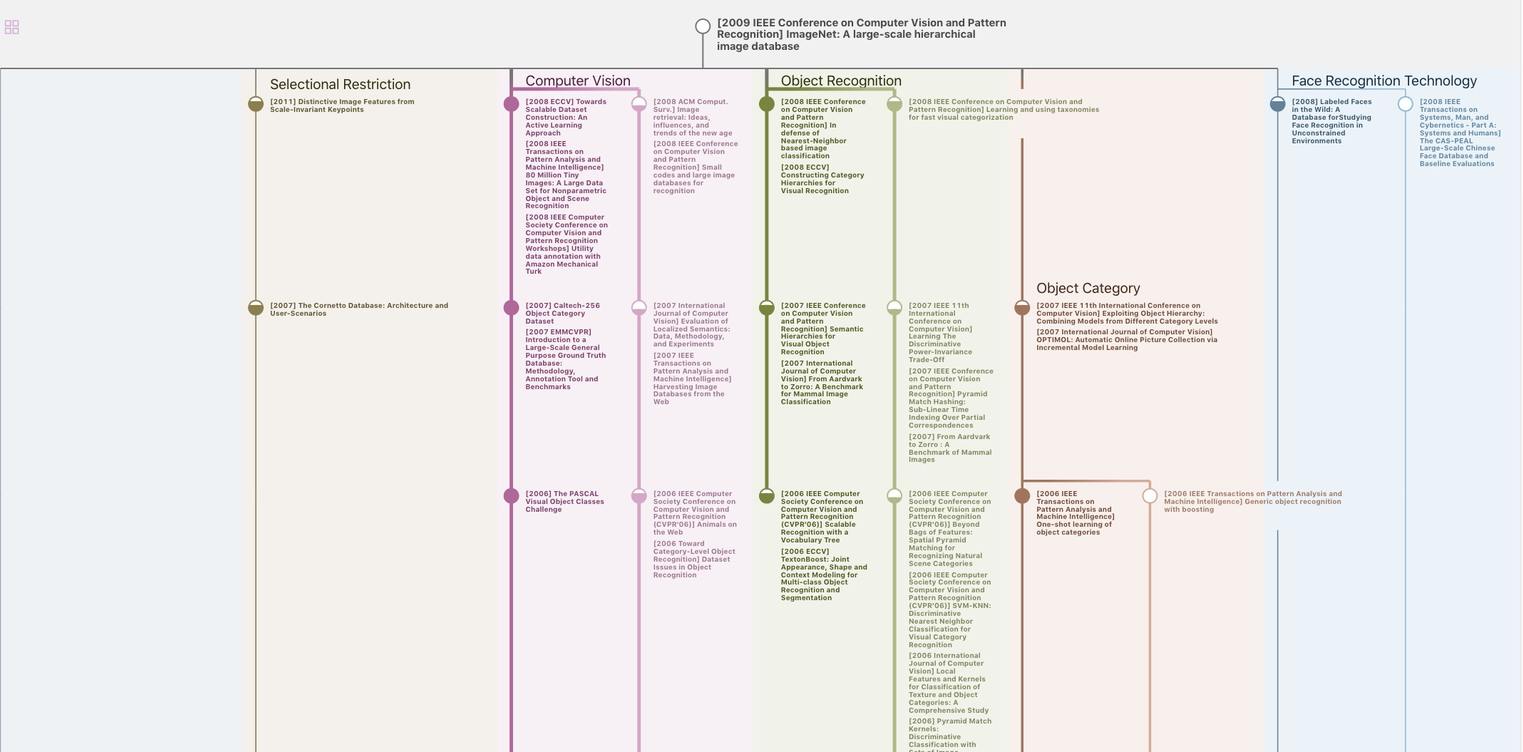
Generate MRT to find the research sequence of this paper
Chat Paper
Summary is being generated by the instructions you defined