Cytoarchitecture and Layer Estimation in High-Resolution Neuroanatomical Images
bioRxiv(2018)
摘要
A robust method for quantifying the cellular architecture (cytoarchitecture) of the brain is a requisite for differentiating brain areas, identifying neurological diseases, and modeling architectural differences across species. Current methods for characterizing cytoarchitecture and, in particular, identifying laminar (layer) divisions in tissue samples, require the expertise of trained neuroanatomists to manually annotate the various regions within each image. However, as neuroanatomical datasets grow in volume, manual annotations become inefficient, impractical, and risk the potential of biasing results. In this paper, we propose an automated framework for cellular density estimation and detection of laminar divisions within retinal and neocortical datasets. This method is based upon the use of sparse recovery methods to simultaneously denoise cellular densities and detect transitions in the density which mark the beginning and end of layers. Retinal and neocortical images are used to demonstrate the efficacy of the methods. These results demonstrate the feasibility of using automation to reveal the cytoarchitecture of neurological samples in high-resolution images.
更多查看译文
关键词
Cytoarchitecture estimation,sparse approximation,inhomogeneous Poisson processes,Total Variation (TV)-minimization,group-sparse regularization,group-sparse regularization
AI 理解论文
溯源树
样例
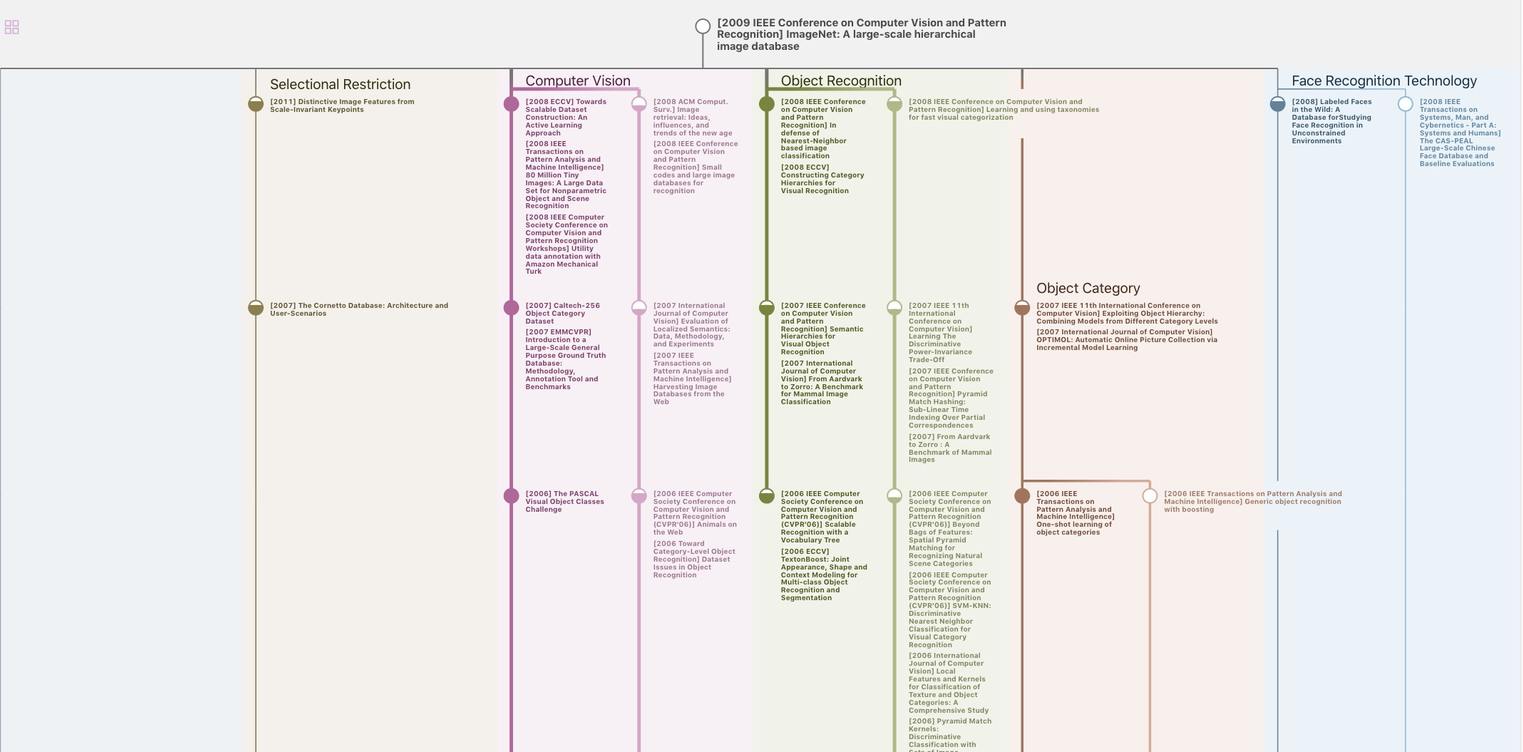
生成溯源树,研究论文发展脉络
Chat Paper
正在生成论文摘要