Fault diagnosis of wind turbine based on Long Short-term memory networks
Renewable Energy(2019)
摘要
Time-series data is widely adopted in condition monitoring and fault diagnosis of wind turbines as well as other energy systems, where long-term dependency is essential to form the classifiable features. To address the issues that the traditional approaches either rely on expert knowledge and handcrafted features or do not fully model long-term dependencies hidden in time-domain signals, this work presents a novel fault diagnosis framework based on an end-to-end Long Short-term Memory (LSTM) model, to learn features directly from multivariate time-series data and capture long-term dependencies through recurrent behaviour and gates mechanism of LSTM. Experimental results on two wind turbine datasets show that our method is able to do fault classification effectively from raw time-series signals collected by single or multiple sensors and outperforms state-of-art approaches. Furthermore, the robustness of the proposed framework is validated through the experiments on small dataset with limited data.
更多查看译文
关键词
Wind turbine,Fault diagnosis,Long short-term memory (LSTM)
AI 理解论文
溯源树
样例
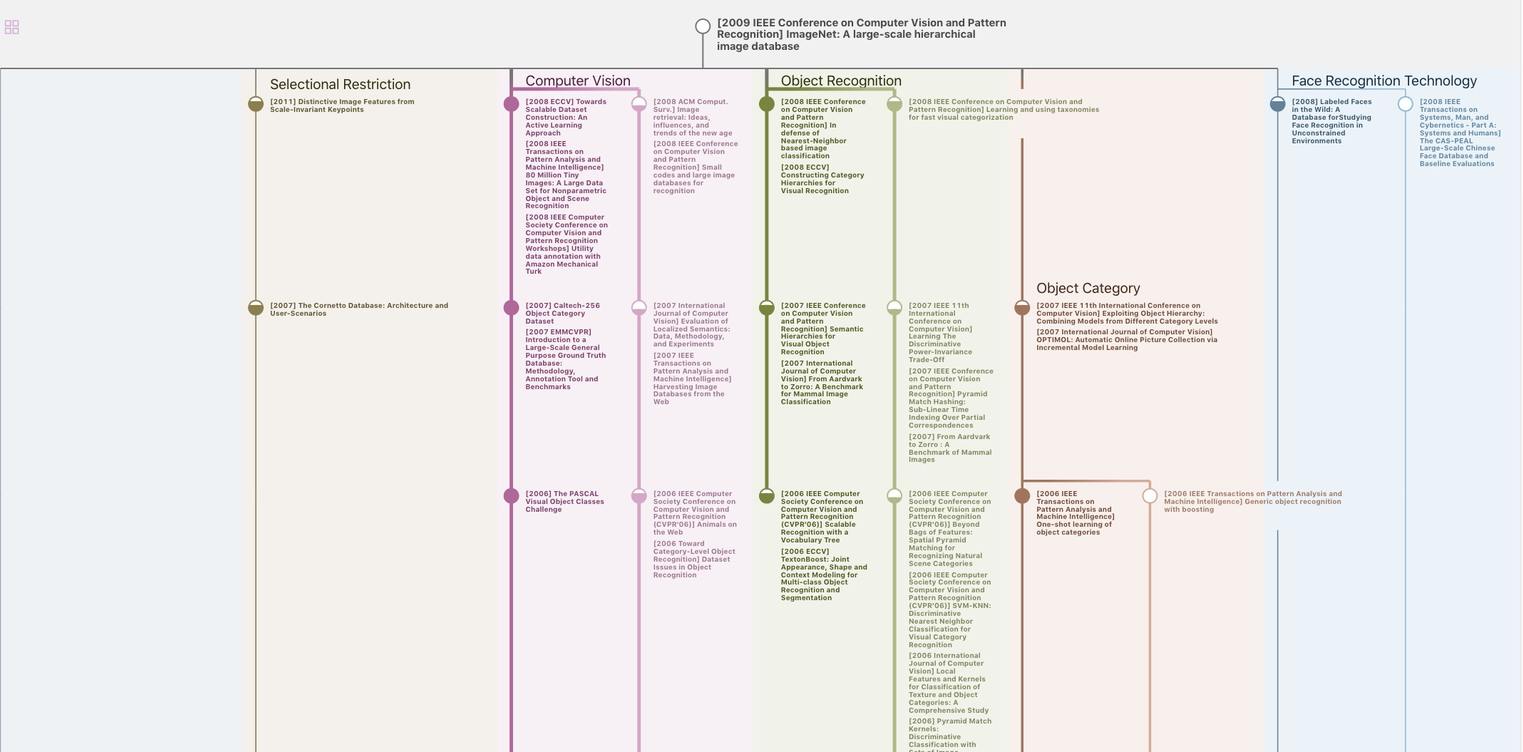
生成溯源树,研究论文发展脉络
Chat Paper
正在生成论文摘要