Matching estimators for causal effects of multiple treatments
STATISTICAL METHODS IN MEDICAL RESEARCH(2020)
摘要
Matching estimators for average treatment effects are widely used in the binary treatment setting, in which missing potential outcomes are imputed as the average of observed outcomes of all matches for each unit. With more than two treatment groups, however, estimation using matching requires additional techniques. In this paper, we propose a nearest-neighbors matching estimator for use with multiple, nominal treatments, and use simulations to show that this method is precise and has coverage levels that are close to nominal. In addition, we implement the proposed inference methods to examine the effects of different medication regimens on long-term pain for patients experiencing motor vehicle collision.
更多查看译文
关键词
Causal inference,generalized propensity score,multiple testing,nominal exposure,observational data
AI 理解论文
溯源树
样例
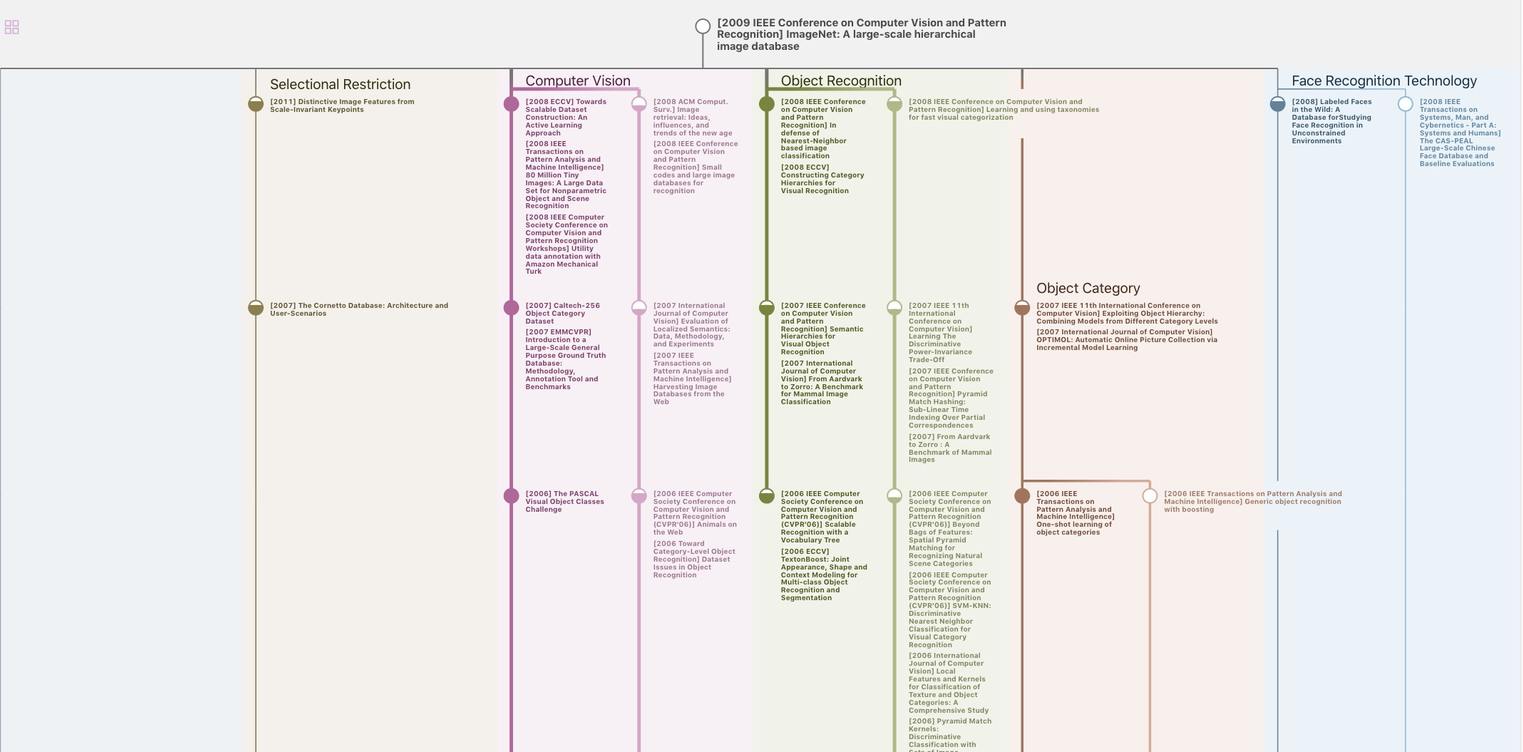
生成溯源树,研究论文发展脉络
Chat Paper
正在生成论文摘要