Efficient Nonlinear Fault Diagnosis Based on Kernel Sample Equivalent Replacement
IEEE Transactions on Industrial Informatics(2019)
摘要
Contribution plots and reconstruction-based contribution (RBC) are efficient linear diagnosis tools in multivariate statistical process monitoring. Unfortunately, they cannot be directly applied to nonlinear fault diagnosis with kernel-based methods due to kernel function covers up the information of the original process variables. Although existing kernel gradient-based approaches have solved this problem to a certain extent, they are still far from suitable for practical applications because they require extremely huge amounts of computation. Their calculations cannot be obtained in a tolerable time unless expensive hardware costs are involved. This paper will thoroughly address this issue by revealing a hidden but important equivalent relationship between the variance-covariance matrix of a centralized process variables matrix and the centralized kernel matrix. Based on this relationship, the nonlinear detection index can be transformed into an explicit quadratic form of variables sample, such that contribution plots and RBC can be directly applied to kernel-based fault diagnosis with a very limited amount of computation, just as their usages in the linear cases. Simulation results obtained from two industrial examples demonstrate the effectiveness of the new method.
更多查看译文
关键词
Kernel,Fault diagnosis,Indexes,Principal component analysis,Fault detection,Covariance matrices,Monitoring
AI 理解论文
溯源树
样例
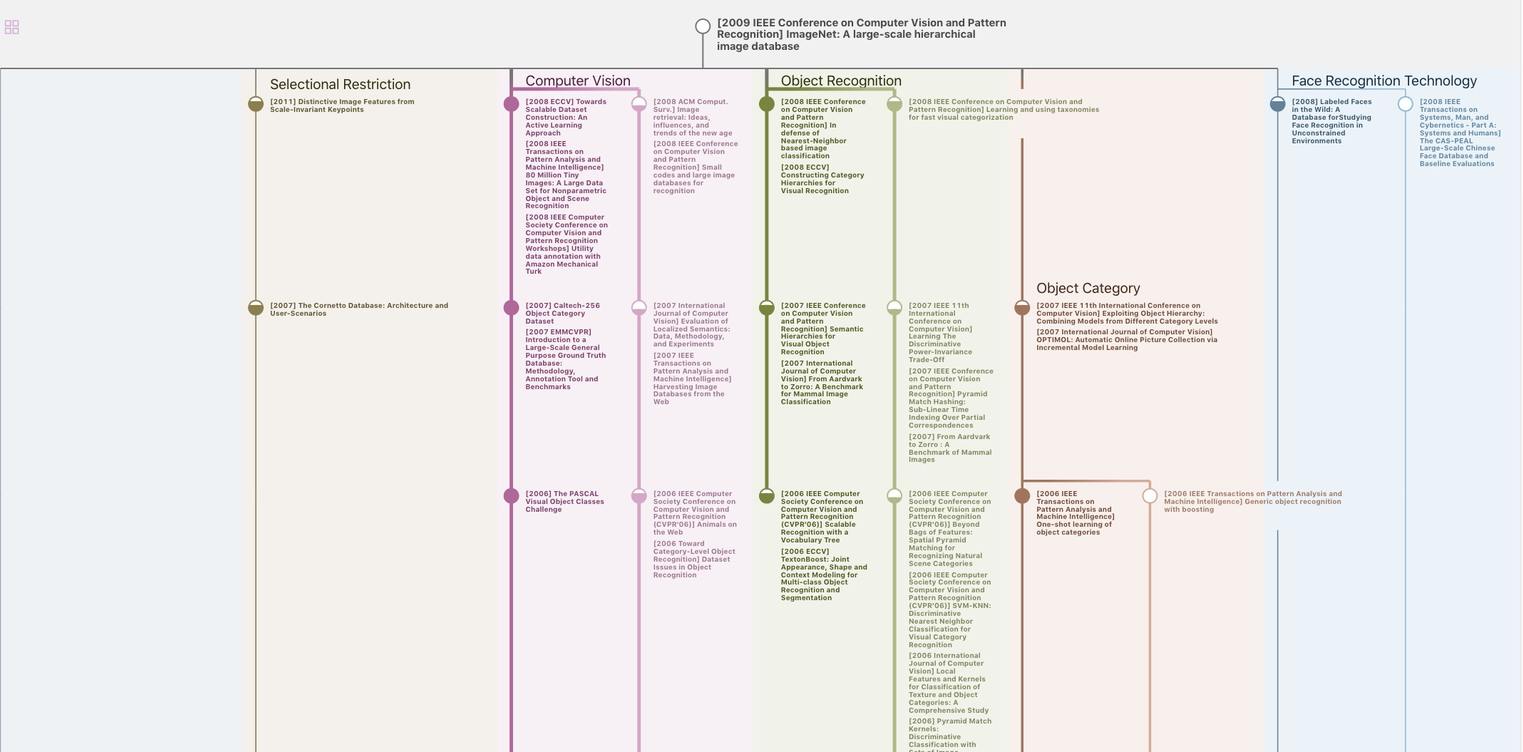
生成溯源树,研究论文发展脉络
Chat Paper
正在生成论文摘要