Gather-Excite: Exploiting feature context in ConvNets
neural information processing systems(2018)
摘要
The powerful image representations learned by deep convolutional neural networks (ConvNets) have propelled this family of models to a state of dominance in image classification. But by constructing features in a strictly bottom-up manner with local operators, ConvNets may be unable to efficiently exploit contextual information that resides in the relationships between features. The focus of this work is to propose a simple, lightweight solution to the issue of limited context propagation in ConvNets. Our approach, which we formulate as a gather-scatter operator pair, propagates context across a group of neurons by aggregating responses over their extent and redistributing the aggregates back through the group. The simplicity of our approach brings several benefits: the operators add few parameters, minimal computational overhead and, importantly, can be directly integrated into existing architectures to improve performance without careful hyperparameter tuning. We present evidence that integration of gather-scatter operators into a ConvNet produces qualitatively different intermediate feature representations. Moreover, we show with experiments on the CIFAR-10, CIFAR-100 and ImageNet datasets that improving context diffusion can be just as important as increasing the depth of a network, at a fraction of the cost. In fact, we find that by supplementing a ResNet-50 model with gather-scatter operators, it is able to outperform its 101-layer counterpart on ImageNet with no additional learnable parameters.
更多查看译文
AI 理解论文
溯源树
样例
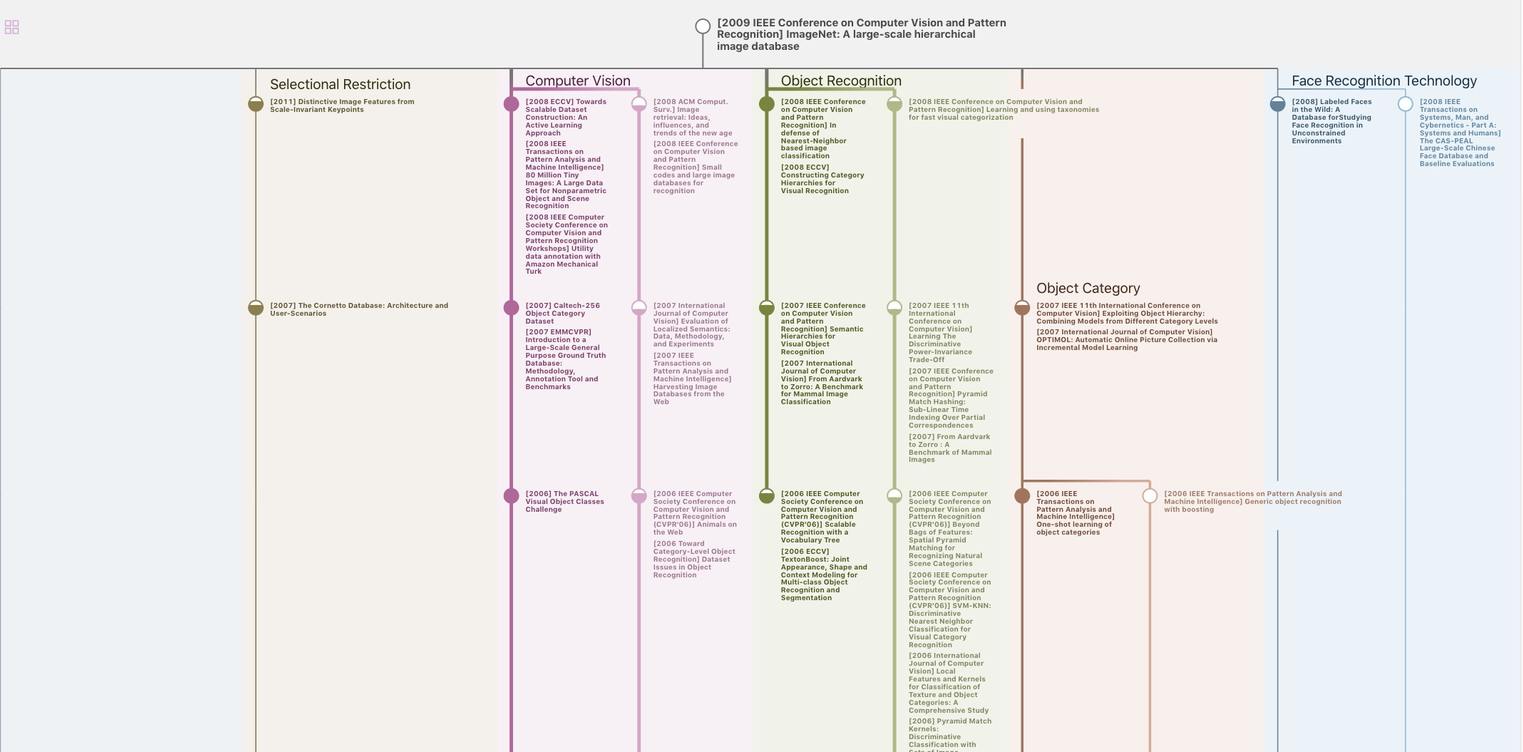
生成溯源树,研究论文发展脉络
Chat Paper
正在生成论文摘要