Countering The Forgetting Effect In Mixed-Model Manual Assembly
IFAC PAPERSONLINE(2018)
摘要
At present, manufacturing companies are increasingly feeling the need to respond rapidly on the changing customer demands. Mass customization was introduced in order to meet the market's needs. A direct result of the change in manufacturing strategy is an increase of the complexity for manual assembly operators. Due to a large variety of assembly tasks, the operator is cognitively challenged because he is not supposed to perform every task evenly frequently. This causes an unlearning phase in which the operator's cycle time increases. Some important learning forgetting models are discussed in this paper to get insights in the phenomenon. Furthermore, different counter strategies against the forgetting effect are listed and discussed. Opportunities and limitations of the strategies were pointed out. An approach for estimating the optimal conditions for retraining is described. A new learning forgetting estimation curve that focuses on mixed-model assembly lines must be introduced. The development of this curve must take into account the task similarity of the product mix and the impact on operator learning and forgetting. Using this estimation, optimal conditions for counter strategies can be proposed. The authors suggest that an experimental study should be used to evaluate the outcome of this approach. (C) 2018, IFAC (International Federation of Automatic Control) Hosting by Elsevier Ltd. All rights reserved.
更多查看译文
关键词
Forgetting effect, Manual assembly, Mixed-model, Retraining
AI 理解论文
溯源树
样例
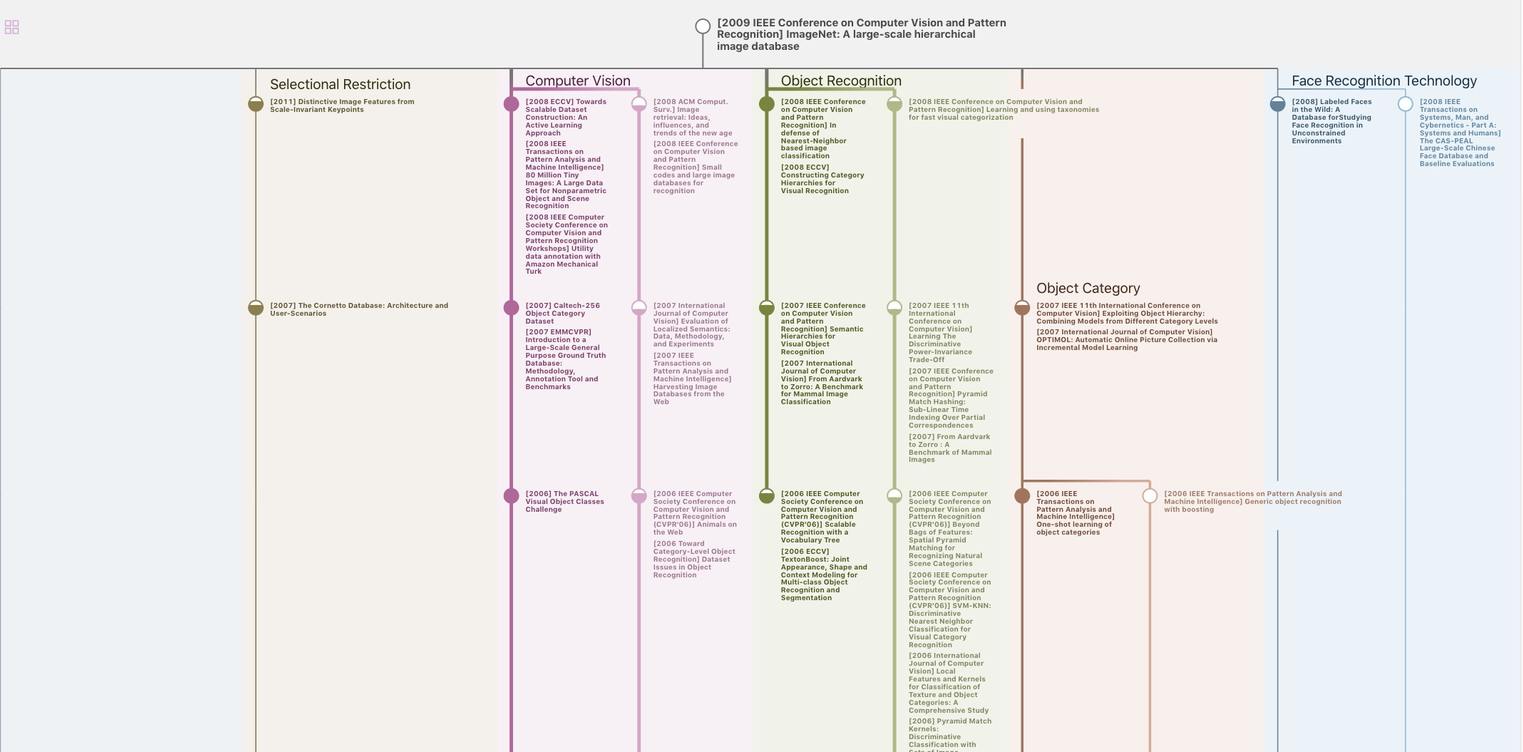
生成溯源树,研究论文发展脉络
Chat Paper
正在生成论文摘要