Non-intrusive Load Composition Estimation from Aggregate ZIP Load Models using Machine Learning
International Journal of Electrical Power & Energy Systems(2019)
摘要
Having continuous load structure and composition information of substations has a great relevance in power system analysis such as load modeling, load forecasting and demand-side management. In this paper, a parsimonious approach for load composition estimation using non-intrusive load disaggregation techniques for low voltage substations is presented with a concept of using ZIP load model characteristics of the aggregate active and reactive powers as predictor features. The disaggregation system uses machine learning algorithms such as Function Fitting Multi-Layer Perceptron Artificial Neural Network (MLP-ANN), Particle Swarm Optimization (PSO) and Genetic Algorithm (GA). During the study, a simulation dataset was generated using Monte Carlo simulation. Moreover, a comparative analysis with a benchmarked paper has been assessed and the proposed approach significantly outperforms.
更多查看译文
关键词
Artificial neural network,Load composition estimation,Load disaggregation,Non-intrusive load monitoring,Substation,ZIP modeling
AI 理解论文
溯源树
样例
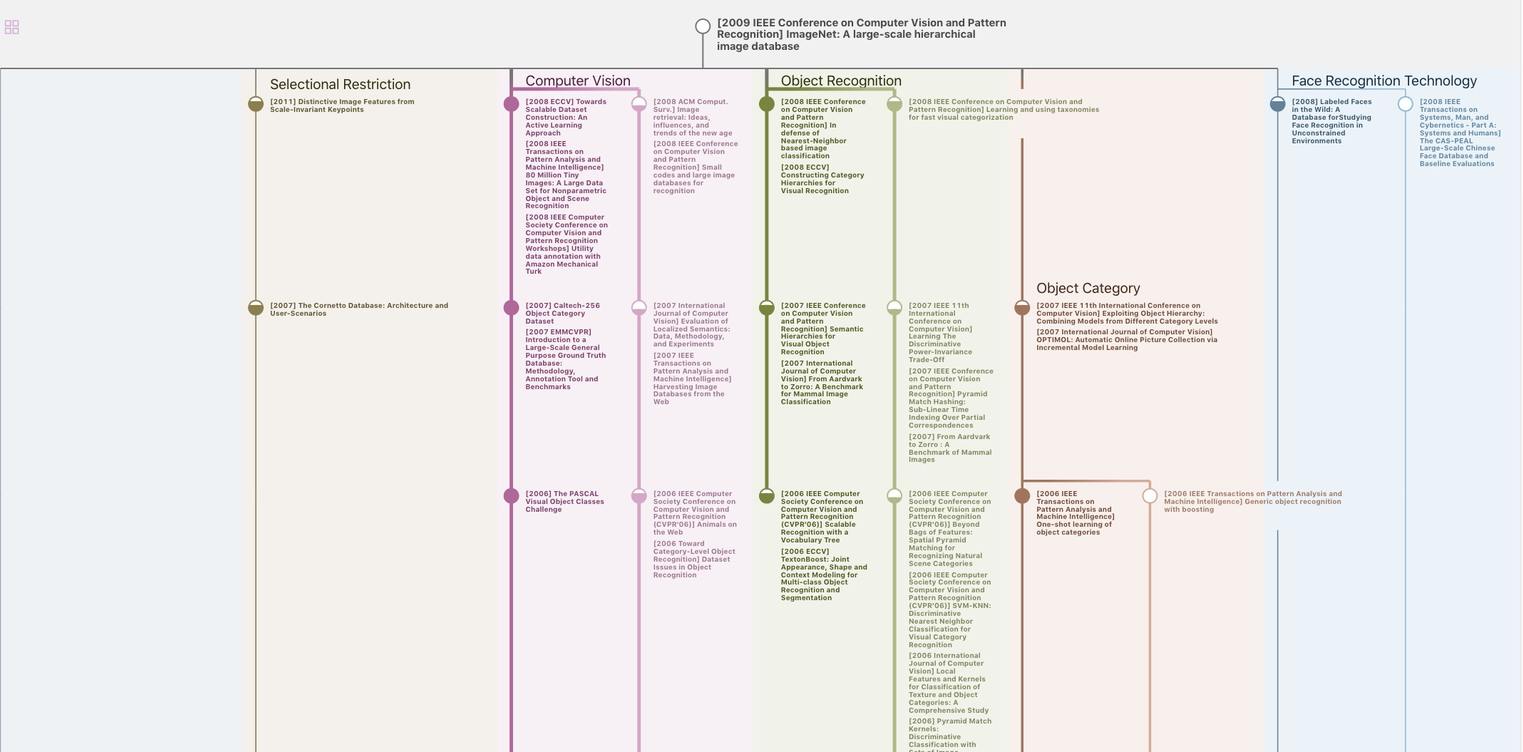
生成溯源树,研究论文发展脉络
Chat Paper
正在生成论文摘要