Assessment Of Amplified Parkinsonian Speech Quality Using Deep Learning
2018 IEEE CANADIAN CONFERENCE ON ELECTRICAL & COMPUTER ENGINEERING (CCECE)(2018)
Abstract
In this paper, deep neural networks (DNNs) are applied to features extracted from Parkinsonian speech recordings to predict their perceived quality. This procedure was also used to benchmark the electroacoustic characteristics of speech amplifiers used by people afflicted with Parkinson Disease (PD). Speech recordings were obtained from 11 PD subjects and 10 normal controls, with and without the assistance of 7 different speech amplifiers, and their quality was assessed subjectively by normal hearing listeners. Mel-frequency and Gammatone frequency cepstral coefficients (MFCCs and GFCCs respectively) and their first order derivatives were extracted as features, and given as input to the DNN. Two optimizers were used to train the neural network, namely stochastic gradient descent (SGD) and Adam optimizers. The paper also shows the effect of feature reduction in enhancing the performance of the objective predictors. Experimental results showed that a trained DNN with reduced set of GFCC features outperforms other objective metrics in terms of correlation with the subjective measures.
MoreTranslated text
Key words
electroacoustic characteristics,Parkinson Disease,normal hearing listeners,mel-frequency,Gammatone frequency cepstral coefficients,neural network,feature reduction,GFCC features,amplified Parkinsonian speech quality,deep learning,deep neural networks,Parkinsonian speech recordings,speech amplifiers
AI Read Science
Must-Reading Tree
Example
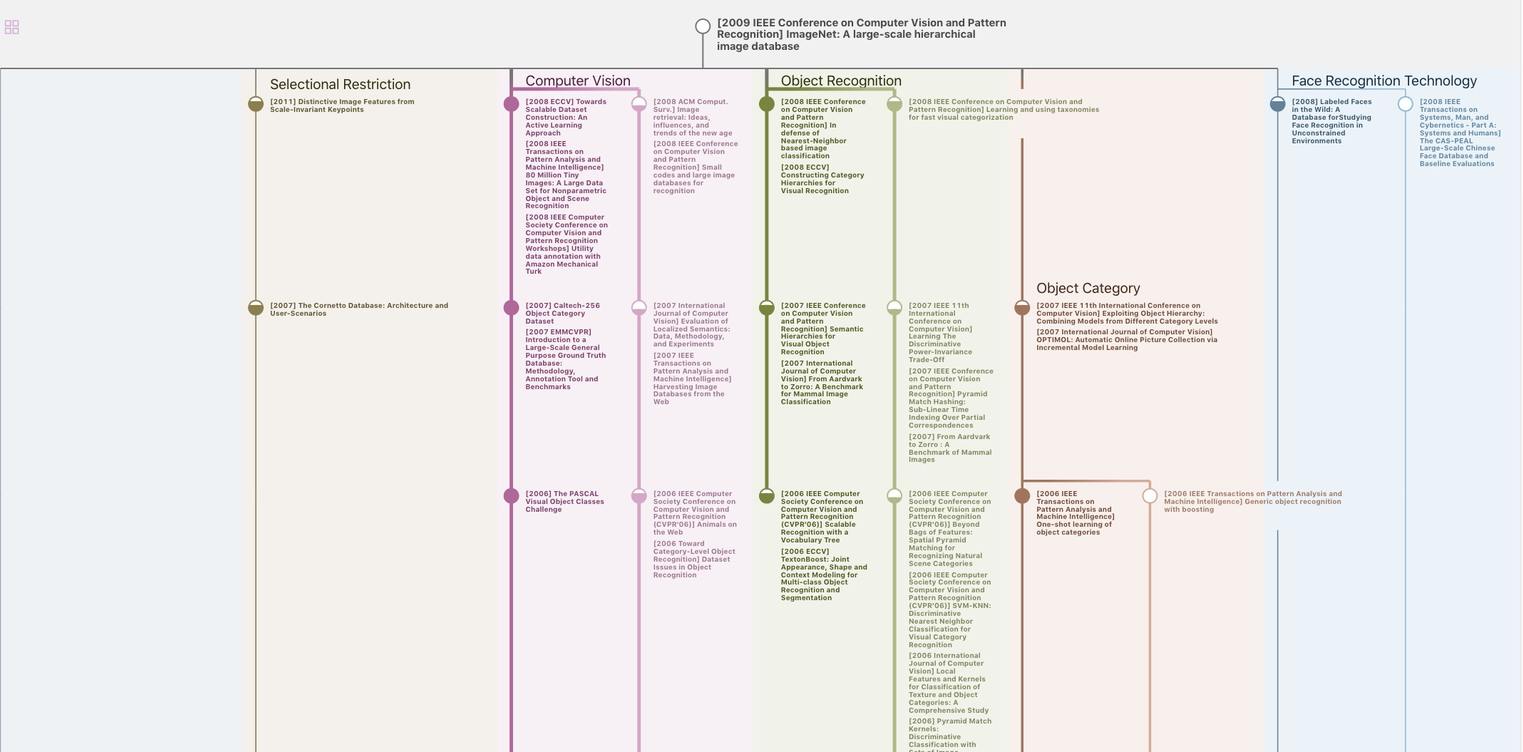
Generate MRT to find the research sequence of this paper
Chat Paper
Summary is being generated by the instructions you defined