Multimodal analysis of structural and functional MRI for schizophrenia diagnosis
Proceedings of SPIE(2018)
摘要
Schizophrenia (SZ) is one of the important brain diseases. Multimodal magnetic resonance (MR) images provide the important imaging biomarkers to detect the pathological changes in both brain function and anatomy for SZ diagnosis. In this paper, we propose a multi-modal image classification algorithm based on sparse coding and random forest to combine the structural and functional MR brain image analysis for SZ diagnosis. First, the structural and functional MR images are processed to extract the anatomical features and functional connectivity measures for representation. Second, for each modality, sparse coding is used for initial feature selection and the selected features are used as input for random forest (RF) models to calculate a proximity matrix for each modality. Third, the features from the two modalities are combined by linear combination of two proximity matrices into one matrix and the classical multidimensional scaling (MDS) is applied to the proximity matrix for dimensionality reduction. Finally, the reduced matrices are served as inputs for the RF models for multi-modal classification. Our proposed algorithm is tested on the structural and functional MRIs for classification of SZ and healthy controls. Both sparse coding and RF have capability of estimating the potential relationship among various features to reach an ideal group discriminating performance. Experimental results show the effectiveness of the proposed multimodal classification method for SZ diagnosis.
更多查看译文
关键词
Random forest,sparse coding,multi-modality,classification,manifold learning,schizophrenia
AI 理解论文
溯源树
样例
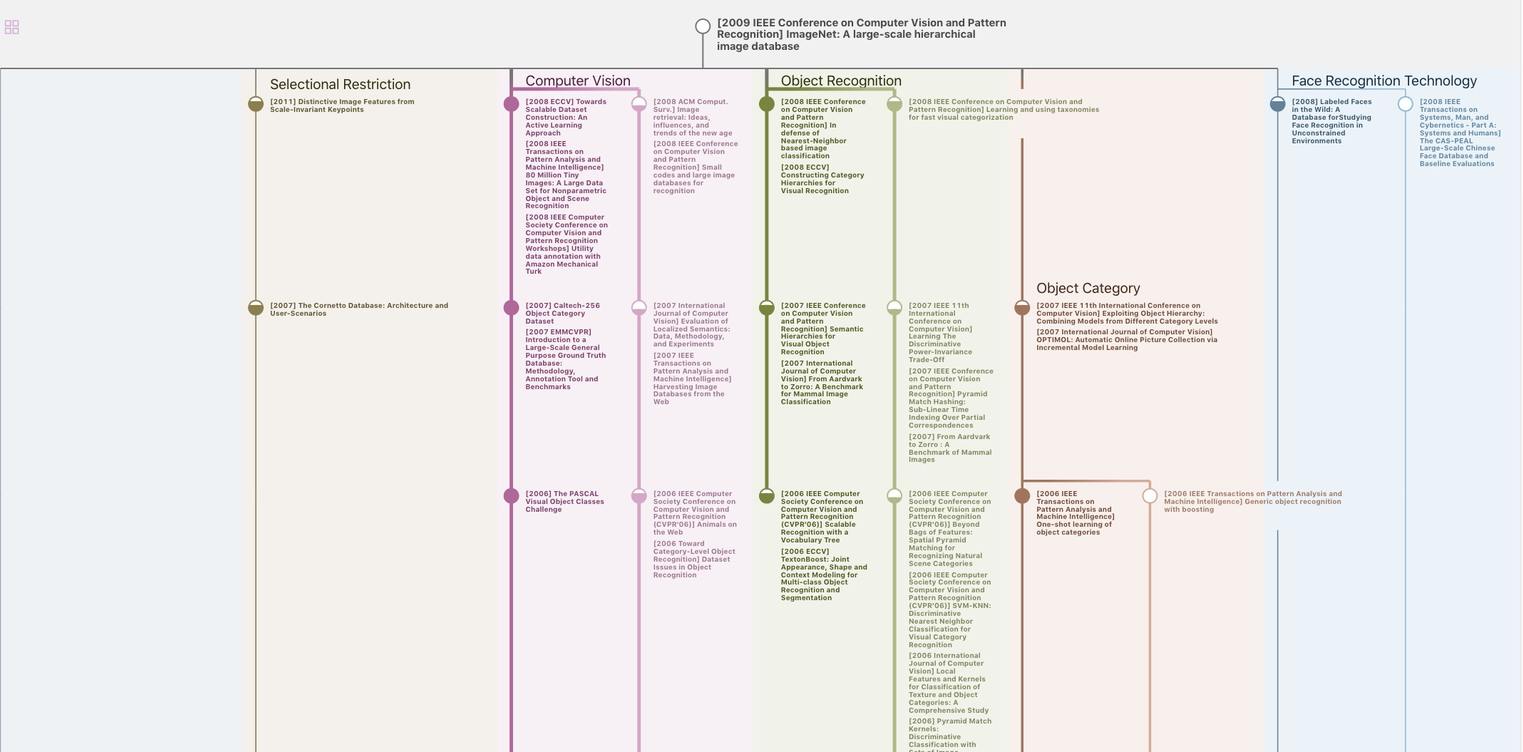
生成溯源树,研究论文发展脉络
Chat Paper
正在生成论文摘要