Robust high dimensional factor models with applications to statistical machine learning.
Statistical science : a review journal of the Institute of Mathematical Statistics(2021)
摘要
Factor models are a class of powerful statistical models that have been widely used to deal with dependent measurements that arise frequently from various applications from genomics and neuroscience to economics and finance. As data are collected at an ever-growing scale, statistical machine learning faces some new challenges: high dimensionality, strong dependence among observed variables, heavy-tailed variables and heterogeneity. High-dimensional robust factor analysis serves as a powerful toolkit to conquer these challenges. This paper gives a selective overview on recent advance on high-dimensional factor models and their applications to statistics including Factor-Adjusted Robust Model selection (FarmSelect) and Factor-Adjusted Robust Multiple testing (FarmTest). We show that classical methods, especially principal component analysis (PCA), can be tailored to many new problems and provide powerful tools for statistical estimation and inference. We highlight PCA and its connections to matrix perturbation theory, robust statistics, random projection, false discovery rate, etc., and illustrate through several applications how insights from these fields yield solutions to modern challenges. We also present far-reaching connections between factor models and popular statistical learning problems, including network analysis and low-rank matrix recovery.
更多查看译文
关键词
Factor model,FarmSelect,FarmTest,PCA,covariance estimation,perturbation bounds,random sketch,robustness
AI 理解论文
溯源树
样例
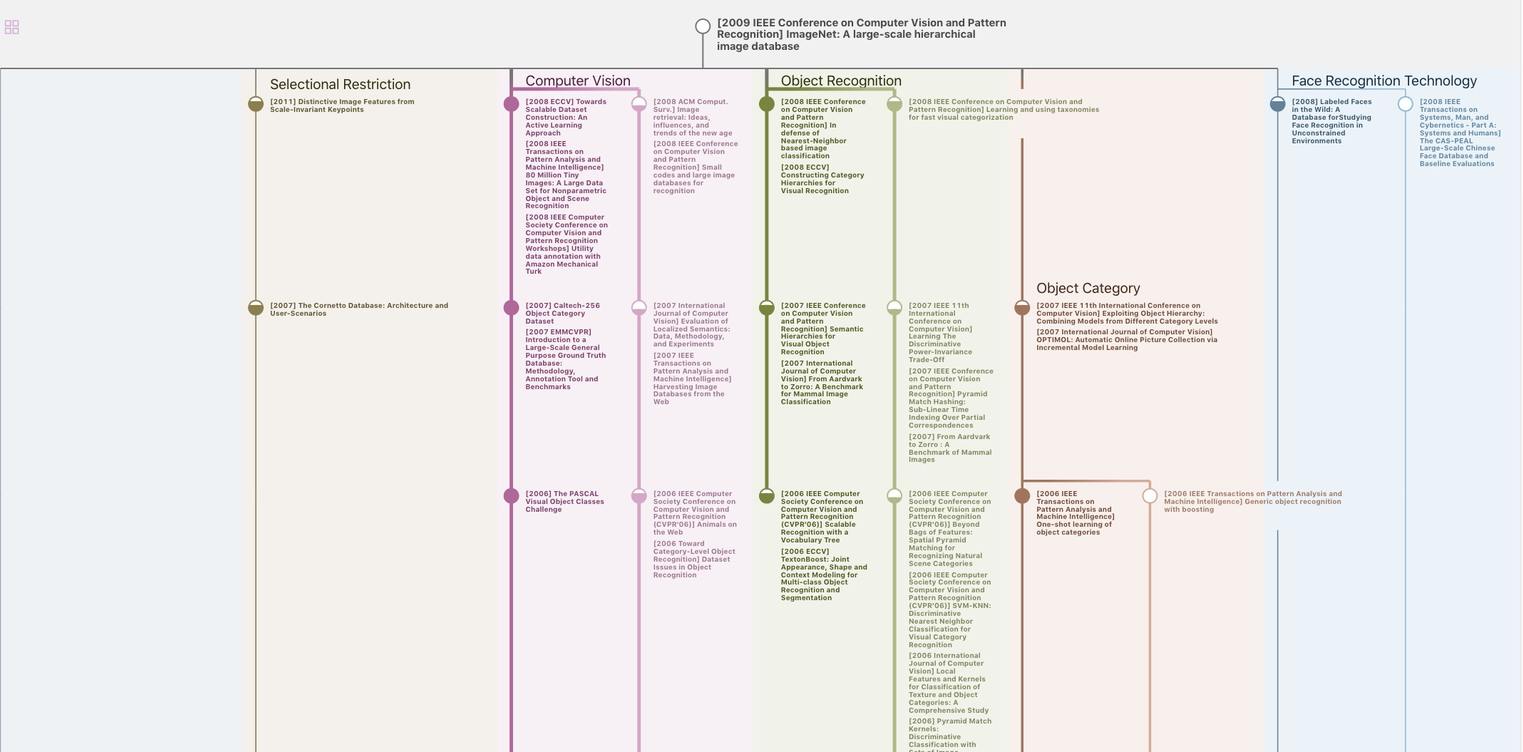
生成溯源树,研究论文发展脉络
Chat Paper
正在生成论文摘要