Single image thin cloud removal for remote sensing images based on conditional generative adversarial nets
Proceedings of SPIE(2018)
摘要
Remote sensing images play a critical role in modern Earth Observation missions, but the interpretations of remote sensing images are easily to be obstructed by the existence of cloud and haze. In this paper, a novel end-to-end generative Single-image thin Cloud and haze Removal Network, referred to as SCR-net, is proposed. The designed SCR-net is a generative model based on Conditional Generative Adversarial Nets (C-GANs), which uses a generator network G to learn the conditional distribution of the cloud-free data and a multi-scale discriminator D to distinguish between real and fake images. Through supervised training, the generator G can predict a cloud-free image using the input noise and a cloud-contaminated image of the same spot. An adversarial minimax objective is used to drive G to learn the distribution of real cloud-free data, and a distance objective in both feature space and image space ensures the quality of the generated image. Quantitative and qualitative experiments are conducted on the synthesized images and real images. Results reveal that the proposed method can effectively remove even and uneven thin cloud in remote sensing images of various scenes, along with good color consistency.
更多查看译文
关键词
Thin cloud removal,image enhancement,Conditional Generative Adversarial Nets,multi-scale feature
AI 理解论文
溯源树
样例
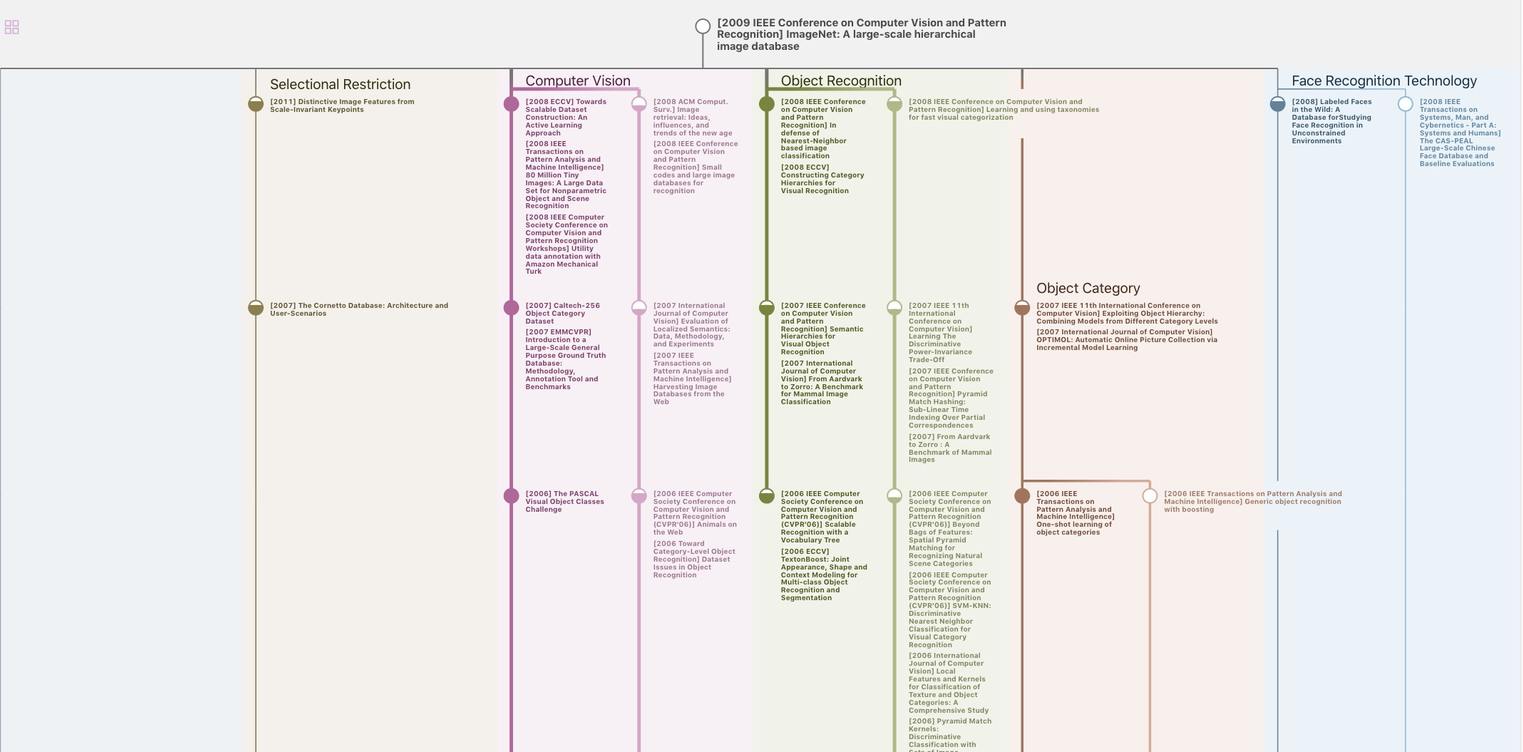
生成溯源树,研究论文发展脉络
Chat Paper
正在生成论文摘要