Cancer Cell Specific Lethality By Degrading Specific Protein Target Network Identified Using A Chemical Screening Based Machine Learning Method
Cancer Research(2018)
Abstract
Cancer is a heterogeneous disease that can be understood as a combination of several pathways common to different cancer types or other non-cancer related diseases. Cancer drug resistance and variability in drug efficacy, a major problem in the field of cancer therapy, occurs due to heterogeneity in cancer cells specific pathways (networks) resulting in an ‘arms race9 due to progression of disease pathogenesis. Identifying cancer cell specific protein network to target from a long list of known targets for each cancer type is an essential solution that may also be useful for other cancers types with similar overlapping networks. Unfortunately, the traditional process of drug design does not consider identification and targeting a network of proteins in a cancer cell specific manner. To our knowledge, no method exists to simultaneously identify the targeted network and design non-toxic, potent synthetic libraries in a cancer cell specific manner. We have developed a method to use machine learning, modeling and experimental cellular data to identify the cell specific targeted network while simultaneously design and synthesize compound library to degrade the identified network of proteins for anticancer potency. We will present our approach for Castration Resistant Prostate Cancer (CRPC) using all known targets for prostate cancer. We have designed and synthesized non-toxic potent anticancer molecules for prostate cancer cells (LnCaP and C4-2 cells with most potent IC 50 ~ 0.72 nM on C4-2 and non-toxic in tissue-specific epithelial cells and in mice at high-dose). We selected compound designs for synthesis that were predicted to be active by training the machine learning algorithm on the set of active (IC 50 ≤100nM) and inactive compounds tested experimentally for prostate cancer cell types in an iterative manner. Our potent anticancer molecules degraded the androgen receptor (AR, known prostate cancer target) more than known potent AR inhibitor (Enzalutamide), even though it was not explicitly developed to target AR. Furthermore, we identified major “features” (proteins) based on the compound-proteome interaction profile that is common to all potent compounds from our entire library. We also identified compound-specific protein networks predicted to be inhibited by each potent anticancer compound. Finally, we experimentally validated degradation of our targeted network predictions prospectively, and identified a network of RORγ, AKR1C4, CYP17A1, SHBG and AR as differential targets for our most potent, non-toxic synthetic molecule. Our results suggest that potent non-toxic molecules prefer specific protein targeted network which may not rank the known “traditional” target (e.g. AR) as the top target to develop molecules for effective anticancer potency in a cell-specific manner. We conclude that our chemical screening based machine learning method can be used more generally to simultaneously identify protein-targeted networks (pathways), guide selection and synthesis of specific compounds for desired cell-specific activity (e.g. anticancer potency), as well as, suggest a rational way to approach drug combinations based on selected cell-specific networks that may be used to combat intra- and inter-cancer heterogeneity. Citation Format: Joydeb Majumder, Jonathan A. Fine, Travis C. Lantz, Cory J. Conder, Gaurav Chopra. Cancer cell specific lethality by degrading specific protein target network identified using a chemical screening based machine learning method [abstract]. In: Proceedings of the American Association for Cancer Research Annual Meeting 2018; 2018 Apr 14-18; Chicago, IL. Philadelphia (PA): AACR; Cancer Res 2018;78(13 Suppl):Abstract nr LB-076.
MoreTranslated text
Key words
specific protein target network,cancer cell,chemical screening,machine learning
AI Read Science
Must-Reading Tree
Example
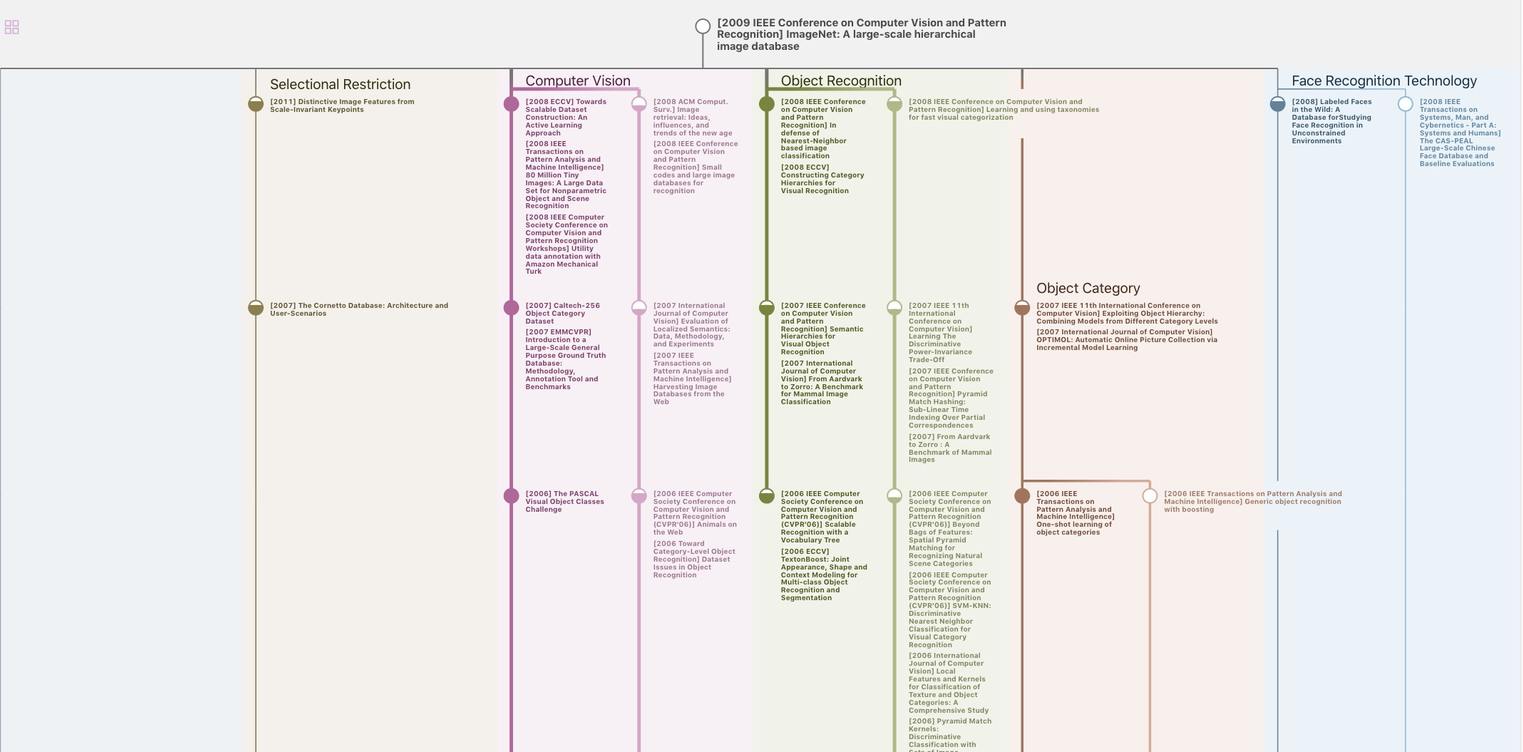
Generate MRT to find the research sequence of this paper
Chat Paper
Summary is being generated by the instructions you defined