Global complexity analysis of inexact successive quadratic approximation methods for regularized optimization under mild assumptions
Journal of Global Optimization(2020)
摘要
Successive quadratic approximations (SQA) are numerically efficient for minimizing the sum of a smooth function and a convex function. The iteration complexity of inexact SQA methods has been analyzed recently. In this paper, we present an algorithmic framework of inexact SQA methods with four types of line searches, and analyze its global complexity under milder assumptions. First, we show its well-definedness and some decreasing properties. Second, under the quadratic growth condition and a uniform positive lower bound condition on stepsizes, we show that the function value sequence and the iterate sequence are linearly convergent. Moreover, we obtain a o (1/ k ) complexity without the quadratic growth condition, improving existing 𝒪(1/k) complexity results. At last, we show that a local gradient-Lipschitz-continuity condition could guarantee a uniform positive lower bound for the stepsizes.
更多查看译文
关键词
Inexactness, Line search, Successive quadratic approximation, Quadratic growth condition, Linear convergence
AI 理解论文
溯源树
样例
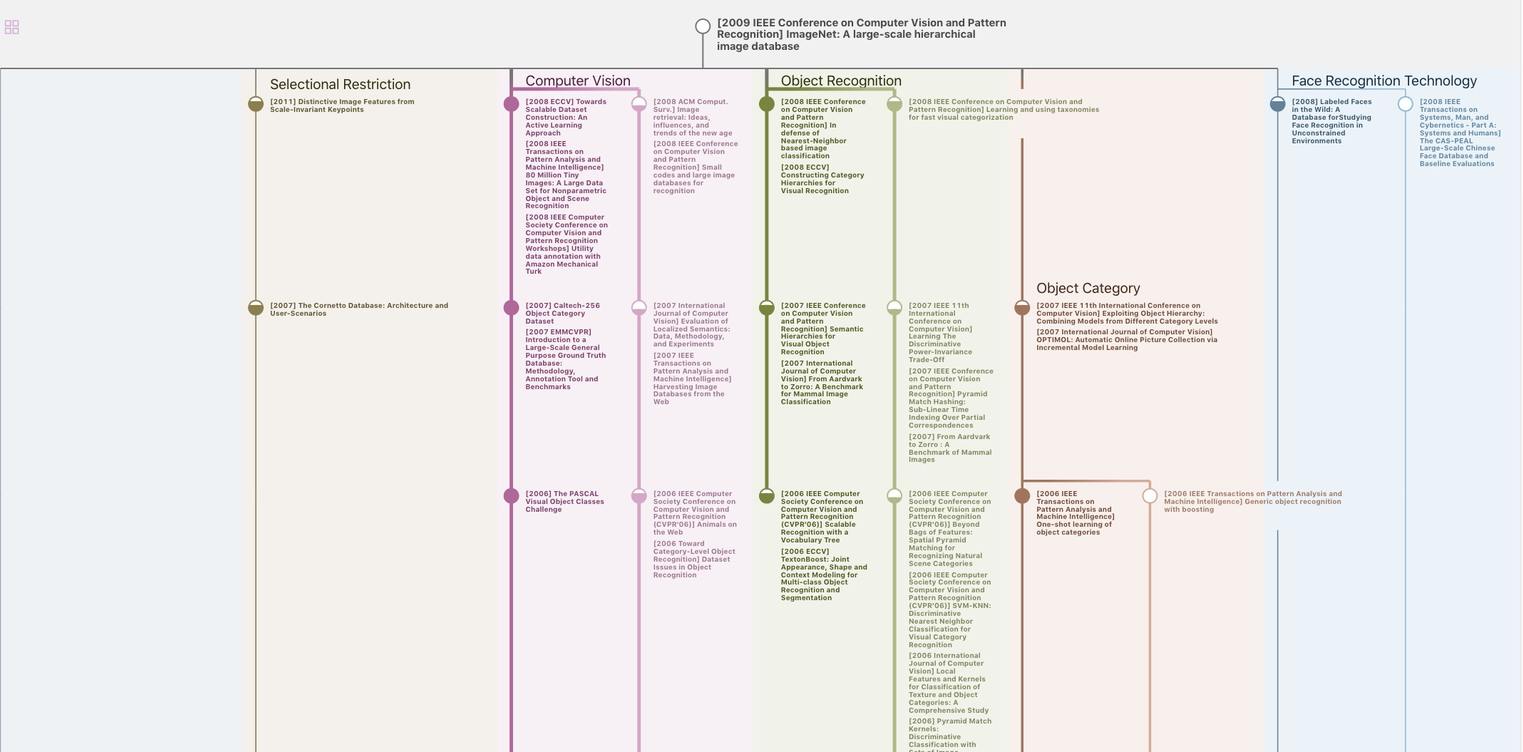
生成溯源树,研究论文发展脉络
Chat Paper
正在生成论文摘要