Nonlinear predictability analysis of brain dynamics for automatic recognition of negative stress
Neural Computing and Applications(2018)
摘要
Negative stress, also named distress, is nowadays one of the most studied emotional states due to its high impact on advanced societies. Its automatic identification from physiological recordings can be extremely useful to prevent concomitant physical health problems as well as other mental disorders. However, this task is still an unresolved challenge. Recently, quadratic sample entropy (QSE) applied to the electroencephalogram signal has proven to be the most promising single metric to discern between emotional states of calm and negative stress. This index estimates repetitive patterns in a time series without considering time data order within each one, thus ignoring some relevant dynamics. Hence, in this work conditional entropy (CEn) and its correction (CCEn) are studied to complement and improve QSE ability in detection of distress. Both CEn and CCEn symbolize original time series to consider ordinal patterns and, thus, quantify accurately data predictability. Results obtained from 279 samples (146 associated with calm and 133 to distress) provide a similar discriminant power, about 65%, both for conditional entropies and QSE. More interestingly, these metrics also reveal the presence of complementary brain dynamics under a emotional state of distress. Thus, CCEn and QSE suggest a synchronization between opposite frontal and parietal brain regions from both hemispheres, such that when the level of negative stress increases, a more irregular activity is found in left frontal and right parietal areas. Simultaneously, more predictable activity is noticed in right frontal and left parietal regions. These findings agree with previous neurophysiological studies and allow to improve the identification of distress. Precisely, a very simple discriminant model based on CCEn and QSE is able to discern more than 80% of samples, thus overcoming slightly the results reported by previous works, where dozens or hundreds of variables have to be combined with advanced classifiers.
更多查看译文
关键词
Distress, EEG, Predictability, Regularity, Symbolization
AI 理解论文
溯源树
样例
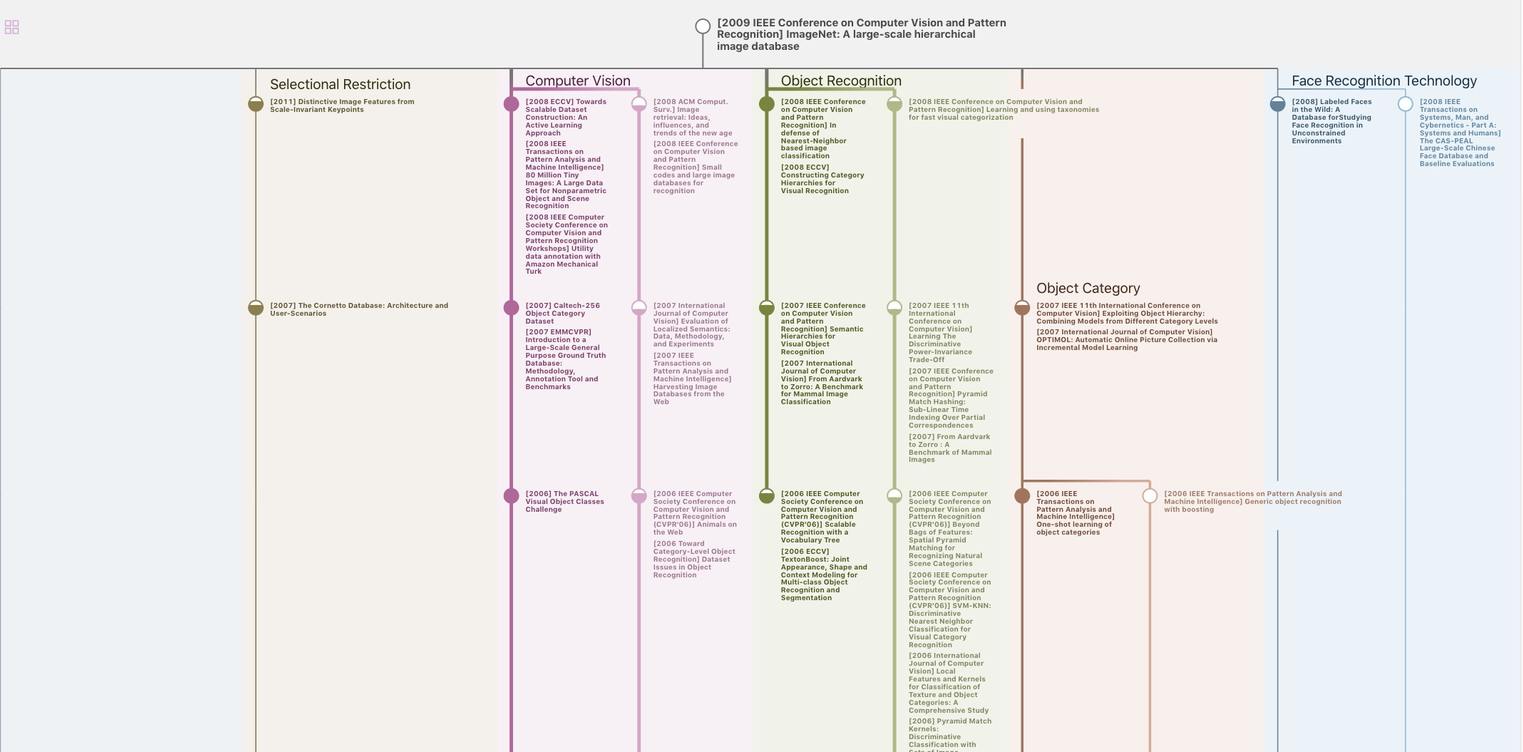
生成溯源树,研究论文发展脉络
Chat Paper
正在生成论文摘要