Predicting the direction of stock market prices using tree-based classifiers
The North American Journal of Economics and Finance(2019)
摘要
Predicting returns in the stock market is usually posed as a forecasting problem where prices are predicted. Intrinsic volatility in the stock market across the globe makes the task of prediction challenging. Consequently, forecasting and diffusion modeling undermines a diverse range of problems encountered in predicting trends in the stock market. Minimizing forecasting error would minimize investment risk. In the current work, we pose the problem as a direction-predicting exercise signifying gains and losses. We develop an experimental framework for the classification problem which predicts whether stock prices will increase or decrease with respect to the price prevailing n days earlier. Two algorithms, random forests, and gradient boosted decisio‘n trees (using XGBoost) facilitate this connection by using ensembles of decision trees. We test our approach and report the accuracies for a variety of companies as improvement over existing predictions. A novelty of the current work is about the selection of technical indicators and their use as features, with high accuracy for medium to long-run prediction of stock price direction.
更多查看译文
关键词
Stock price movement,Xgboost,Random forests,Machine classification
AI 理解论文
溯源树
样例
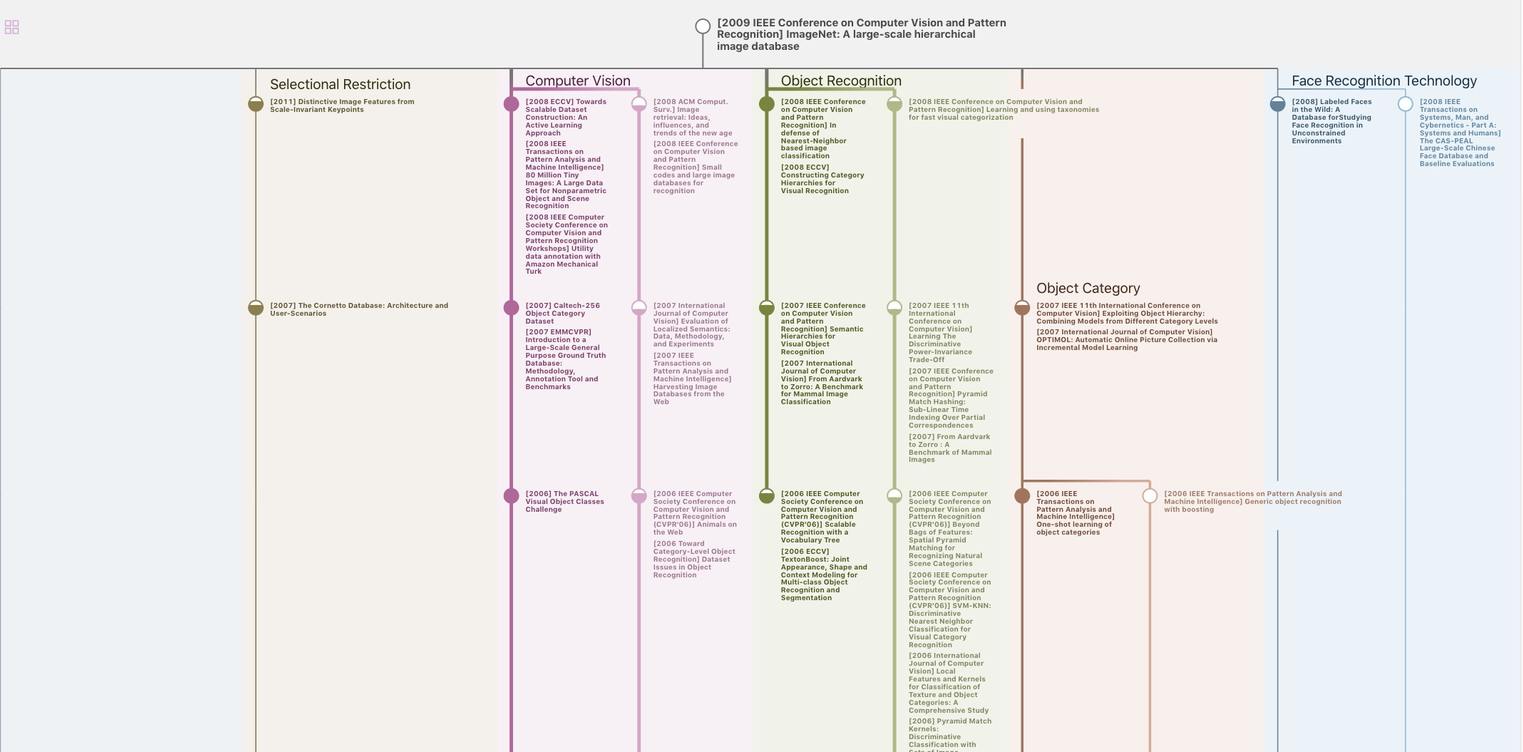
生成溯源树,研究论文发展脉络
Chat Paper
正在生成论文摘要