Leveraging Clinical Fields from a US EMR Database to Identify and Characterize Latent Autoimmune Diabetes in Adults (LADA) in Real World Practice Settings
VALUE IN HEALTH(2018)
Abstract
Absent ICD-9/ICD-10 diagnosis codes for certain diabetes mellitus (DM) subtypes, an analysis of EMR data was performed to identify and characterize a cohort of LADA patients. From the IQVIA US Ambulatory EMR database of 46 million patients from 315 practices, patients were identified as having LADA if there was any record between 1/1/2006-11/30/2017 that specified LADA subtype as part of the problems list (also appearing as “T1.5DM” or “Slowly Progressive Insulin Dependent DM”). Patient demographics and clinical profiles were summarized. A total of 2,332 LADA patients were identified: 49.6% men, mean 55.7±16.0 years of age at earliest LADA record (compared with their mean age at earliest non-LADA diabetes record, 53.6±15.8), and mean database observation period 4.9±3.9 years. Within the subset with available race information (n=1,607), 86.4% were Caucasian and 9.1% were Black. Traditional DM types appearing as ICD-9/ICD-10 diagnosis codes in their EMR history were: 55.5% Type2DM only and 39.0% both Type1DM/Type2DM. Additionally, family history of diabetes was recorded for 47.6%, with 5 patients having family history specific to LADA. Mean BMI (earliest recorded value on any LADA visit date) was 29.9±6.9 kg/m2. Mean HbA1c (peak value) among subset with labs was 9.3±2.4%. From the co-morbid conditions assessed (ICD-9/ICD-10 diagnosis definitions): 64.0% hyperlipidemia, 58.1% hypertension, 14.2% ASCVD, 10.9% CKD/ESRD, and 4.2% diabetic retinopathy. Prescriptions recorded after first LADA diagnosis included 63.0% insulin and 29.0% sulfonylureas (7.7% had no prescription records in EMR data for diabetes medications). Absent specific diagnostic codes, a LADA patient cohort was successfully identified in the EMR data having a profile consistent with known natural history for this diabetes subtype. Large real-world EMR databases can be useful for identifying patients from clinical subtypes that are not otherwise visible through traditional diagnostic coding and may broaden the range of research opportunities targeting many sub-populations.
MoreTranslated text
Key words
Diabetes,Continuous Glucose Monitoring,Type 2 Diabetes,Type 1 Diabetes
AI Read Science
Must-Reading Tree
Example
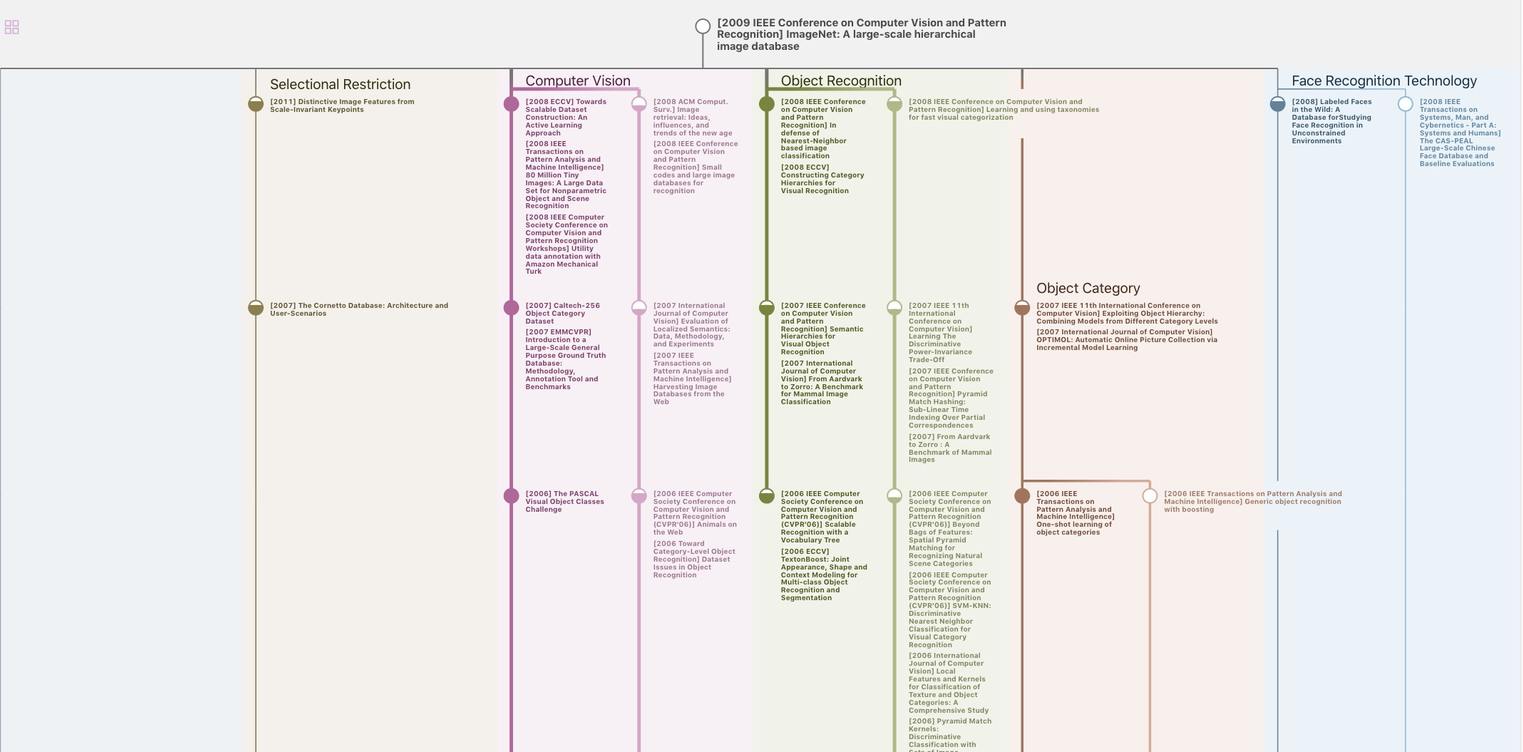
Generate MRT to find the research sequence of this paper
Chat Paper
Summary is being generated by the instructions you defined