An Inequality Constrained Ensemble Kalman Filter For Parameter Estimation Application
2018 IEEE AEROSPACE CONFERENCE(2018)
摘要
This paper proposes a Selective Constrained Ensemble Kalman Filter (SCenKF) for nonlinear parameter estimation application. The ensemble based Kalman filter has two advantages. Firstly, its stability performance is not affected by large initial state estimation error. Secondly, the nonlinear model does not require to be linearized. The inequality constraints are represented in terms of error function and complementary error function. In addition, the characteristics of particle filter and genetic algorithm are integrated into SCenKF to prevent matrix singular problem in Kalman gain computation and enable the memory mechanism on the historical best performance ensemble member. To study the performance of the proposed SCenKF, a case study for photovoltaic (PV) model parameter estimation is used. In this study, the proposed SCenKF is compared with the particle swarm optimization (PSO) and improved comprehensive photovoltaic parameter identification (ICPPI) method. The result shows that the proposed SCenKF achieves better estimation accuracy as compared to the other two methods. In addition, the computational cost of the SCenKF falls between PSO and ICPPI.
更多查看译文
关键词
Selective Constrained Ensemble Kalman Filter,nonlinear model,particle filter,photovoltaic model parameter estimation,comprehensive photovoltaic parameter identification method,nonlinear parameter estimation,state estimation error
AI 理解论文
溯源树
样例
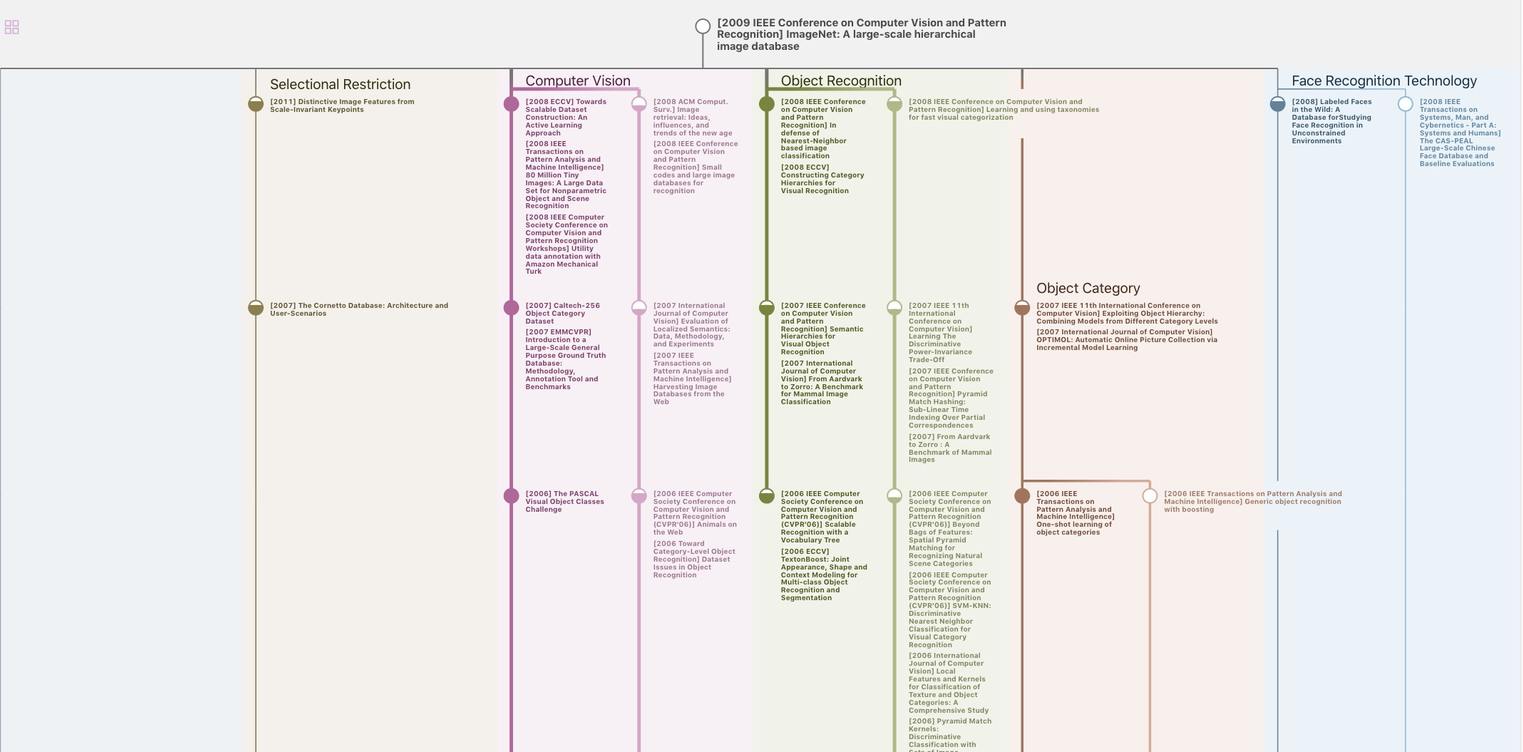
生成溯源树,研究论文发展脉络
Chat Paper
正在生成论文摘要