Upstream Fusion Of Multiple Sensing Modalities Using Machine Learning And Topological Analysis: An Initial Exploration
2018 IEEE AEROSPACE CONFERENCE(2018)
摘要
This paper presents a processing pipeline for fusing 'raw' and / or feature-level multi-sensor data - upstream fusion - and initial results from this pipeline using imagery, radar, and radio frequency (RF) signals data to determine which tracked object, among several, hosts an emitter of interest. Correctly making this determination requires fusing data across these modalities. Our approach performs better than standard fusion approaches that make detection / characterization decisions for each modality individually and then try to fuse those decisions - downstream (or post-decision) fusion. Our approach (1) fully exploits the inter-modality dependencies and phenomenologies inherent in different sensing modes, (2) automatically discovers compressive hierarchical representations that integrate structural and statistical characteristics to enhance target / event discriminability, and (3) completely obviates the need to specify features, manifolds, or model scope a priori. This approach comprises a unique synthesis of Deep Learning (DL), topological analysis over probability measure (TAPM), and hierarchical Bayesian non-parametric (HBNP) recognition models. Deep Generative Networks (DGNs - a deep generative statistical form of DL) create probability measures that provide a basis for calculating homologies (topological summaries over the probability measures). The statistics of the resulting persistence diagrams are inputs to HBNP methods that learn to discriminate between target types and distinguish emitting targets from non-emitting targets, for example. HBNP learning obviates batch-mode off-line learning. This approach overcomes the inadequacy of pre-defined features as a means for creating efficient, discriminating, low-dimensional representations from high-dimensional multi-modality sensor data collected under difficult, dynamic sensing conditions. The invariant properties in the resulting compact representations afford multiple compressive sensing benefits, including concise information sharing and enhanced performance. Machine learning makes adaptivity a central feature of our approach. Adaptivity is critical because it enables flexible processing that automatically accommodates a broad range of challenges that non-adaptive, standard fusion approaches would typically require manual intervention to begin to address. These include (a) interest in unknown or unanticipated targets, (b) desire to be rapidly able to fuse between different combinations of sensor modalities, and (c) potential need to transfer information between platforms that host different sensors. This paper presents results that demonstrate our approach enables accurate, real-time target detection, tracking, and recognition of known and unknown moving or stationary targets or events and their activities evolving over space and time.
更多查看译文
关键词
machine learning,standard fusion approaches,sensor modalities,real-time target detection,stationary targets,upstream fusion,multiple sensing modalities,topological analysis,initial exploration,processing pipeline,feature-level multisensor data,radio frequency,inter-modality dependencies,compressive hierarchical representations,integrate structural characteristics,statistical characteristics,target / event discriminability,probability measure,hierarchical Bayesian nonparametric recognition models,Deep Generative Networks,deep generative statistical form,topological summaries,HBNP methods,emitting targets,nonemitting targets,discriminating representations,low-dimensional representations,high-dimensional multimodality sensor data,difficult sensing conditions,dynamic sensing conditions,multiple compressive sensing benefits,enhanced performance,data fusion,sensing modes,batch-mode off-line learning
AI 理解论文
溯源树
样例
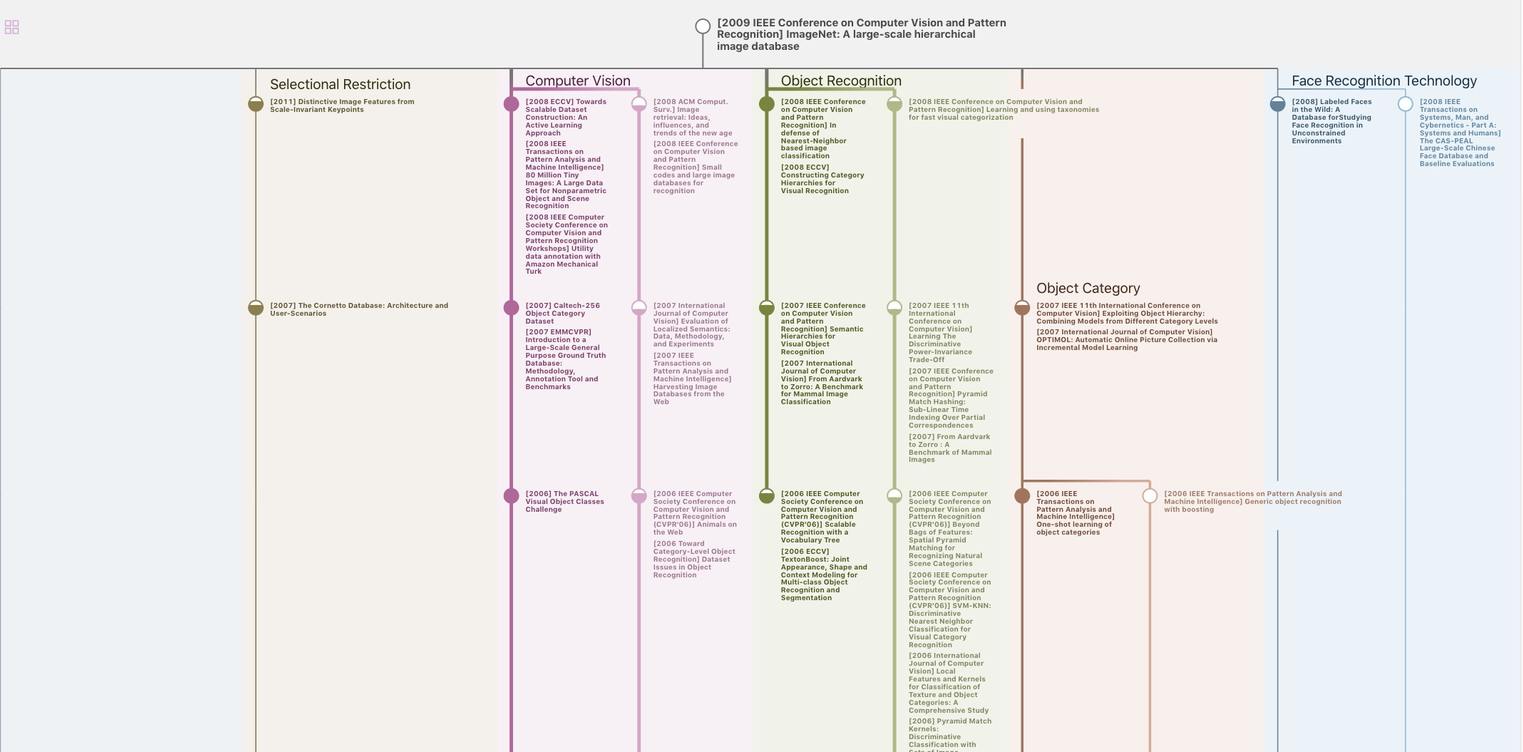
生成溯源树,研究论文发展脉络
Chat Paper
正在生成论文摘要