Neighborhood attribute reduction for imbalanced data
granular computing(2018)
摘要
From the viewpoint of rough granular computing, neighborhood decision error rate-based attribute reduction aims to improve the classification performance of the neighborhood classifier. Nevertheless, for imbalanced data which can be seen everywhere in real-world applications, such reduction does not pay much attention to the classification results of samples in minority class. Therefore, a new strategy to attribute reduction is proposed, which is embedded with preprocessing of the imbalanced data. First, the widely accepted SMOTE algorithm and K-means algorithm are used for oversampling and undersampling, respectively. Second, the neighborhood decision error rate-based attribute reduction is designed for those updated data. Finally, the neighborhood classifier can be tested with the attributes in reducts. The experimental results on some UCI and PROMISE data sets show that our approach is superior to the traditional attribute reduction based on the evaluations of F-measure and G-mean. Therefore, the contribution of this paper is to construct the attribute reduction strategy for imbalanced data, which can select useful attributes for improving the classification performance in such data.
更多查看译文
关键词
Attribute reduction,Granular computing,K-means,Neighborhood decision error rate,Neighborhood classifier,SMOTE
AI 理解论文
溯源树
样例
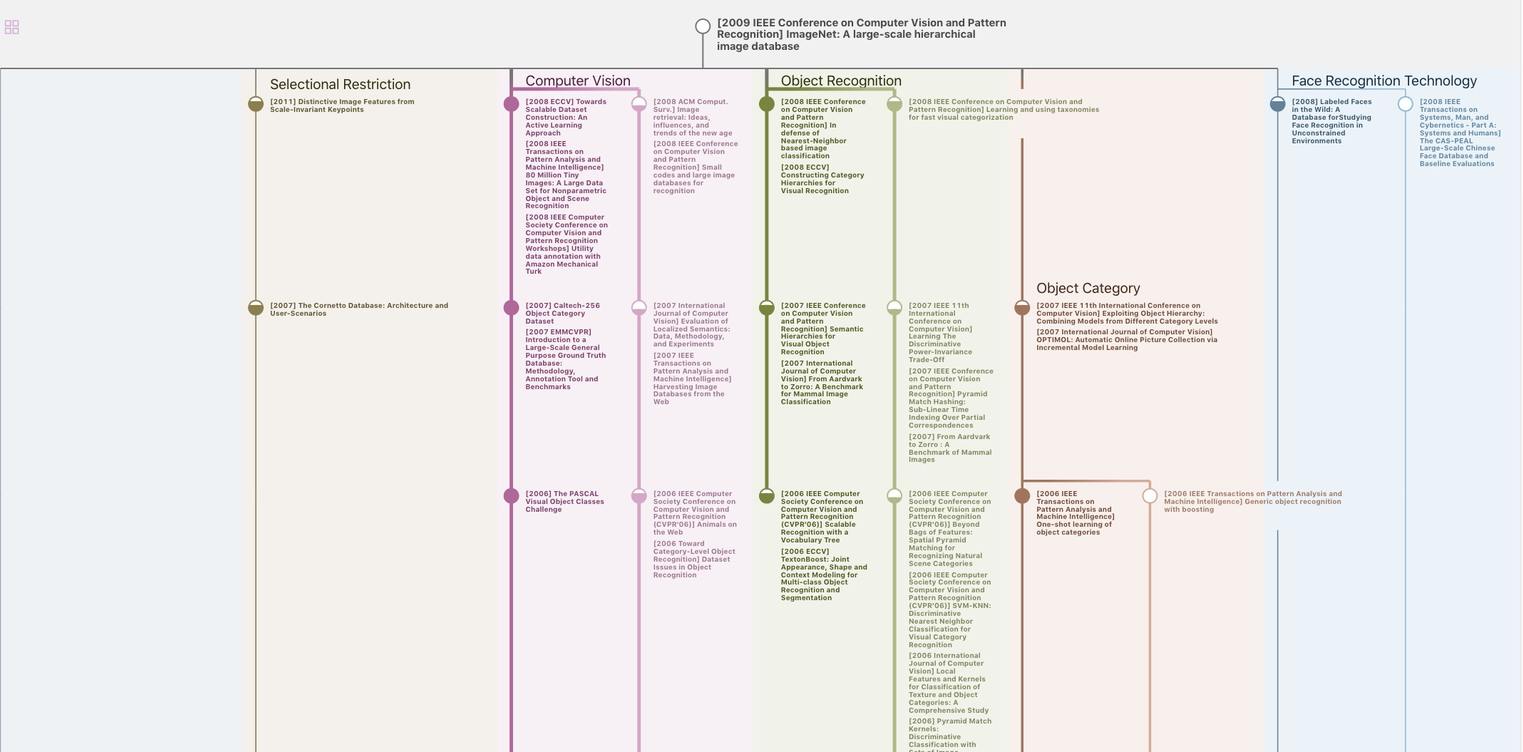
生成溯源树,研究论文发展脉络
Chat Paper
正在生成论文摘要