Effects of Training Samples and Classifiers on Classification of Landsat-8 Imagery
Journal of The Indian Society of Remote Sensing(2018)
Abstract
In this study, we used Landsat-8 imagery to test object- and pixel-based image classification approaches in an urban fringe area. For object-based classification, we applied four machine learning classifiers: decision tree (DT), naive Bayes (NB), random trees (RT), and support vector machine (SVM). For pixel-based classification, we utilized the maximum likelihood classifier (MLC). Specifically, we explored the influence of repeated sampling on classification results with different training sample sizes. We found that (1) except the overall accuracy of NB, those of the other four classifiers increased as the training sample size increased; (2) repeated sampling had a significant effect on classification accuracy, especially for the DT and NB classifiers; and (3) SVM achieved the best classification accuracy. In addition, the performance of the object-based classifiers was superior to that of the pixel-based classifier. The results of this study can provide guidance on the training sample size and classifier selection.
MoreTranslated text
Key words
Object-based classifiers, Pixel-based classifiers, Landsat-8 imagery, Training sample size
AI Read Science
Must-Reading Tree
Example
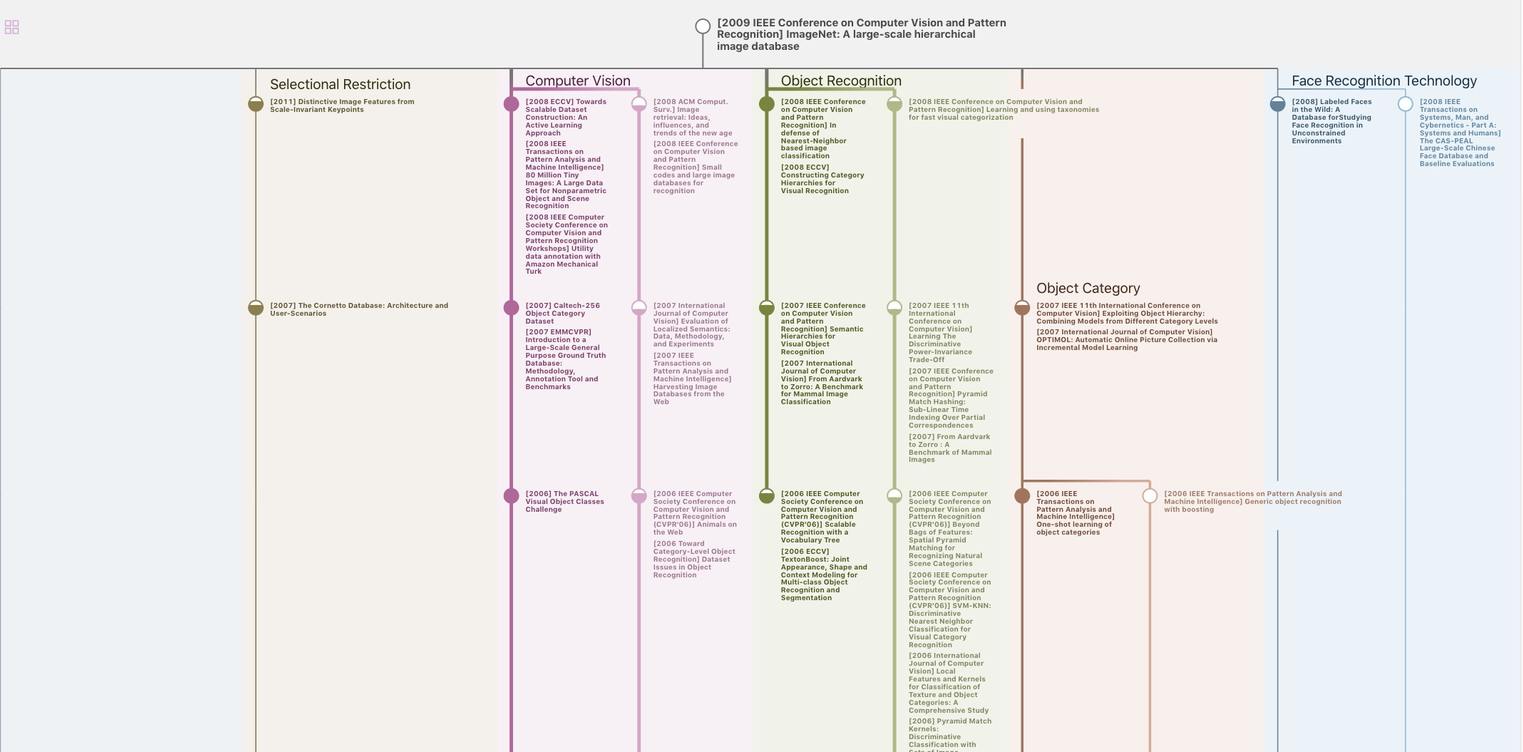
Generate MRT to find the research sequence of this paper
Chat Paper
Summary is being generated by the instructions you defined