Identification Of Heavy Hitters For Network Data Streams With Probabilistic Sketch
2018 IEEE 3RD INTERNATIONAL CONFERENCE ON CLOUD COMPUTING AND BIG DATA ANALYSIS (ICCCBDA)(2018)
摘要
Traffic measurement is critical for many network applications, such as traffic accounting, anomaly detection. One central problem is to identify heavy hitters, flow size of which is more than the given threshold or the certain percentage of total network traffic in the measurement interval. According to low accuracy and memory utilization for traditional methods, we propose an identification algorithm of heavy hitters with probabilistic sketch in high-speed networks (for short HHS) Since the probabilistic sketch consists of a few bit vectors, data updating only needs a small amount of calculation. It can estimate flow size by probabilistic counting approach approximately. Moreover, in order to improve the identification accuracy of heavy hitters, we introduce confidence interval to estimate flow size. We use real network traffic to evaluate the performance of the proposed method. The experimental results illustrate that our method can estimate flow size accurately and identify heavy hitters effectively.
更多查看译文
关键词
heavy hitter identificaation, network data stream, probablistic sketch
AI 理解论文
溯源树
样例
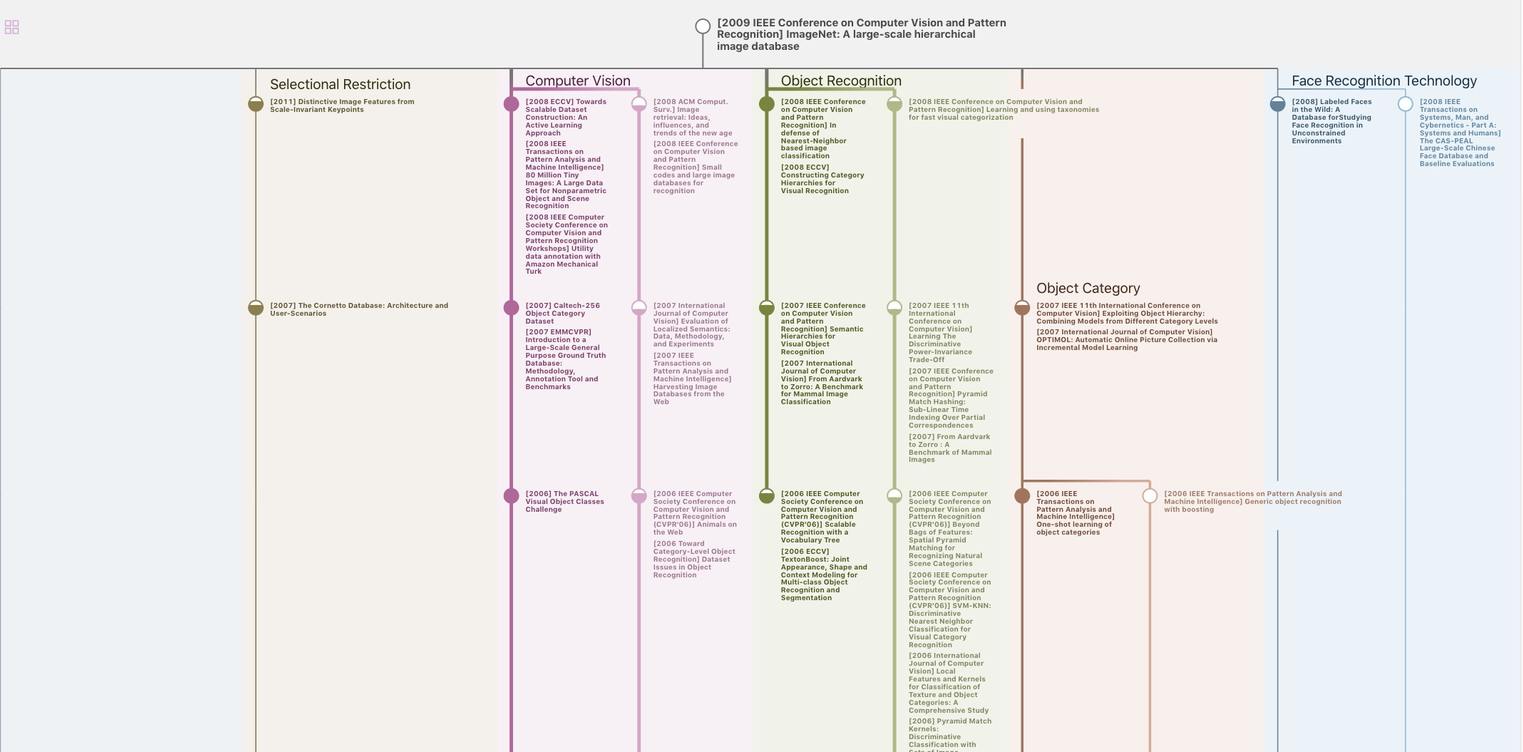
生成溯源树,研究论文发展脉络
Chat Paper
正在生成论文摘要