003 A markov/multi-state model of progression and dynamics of seizure freedom in in large cohort of patients with epilepsy
JOURNAL OF NEUROLOGY NEUROSURGERY AND PSYCHIATRY(2018)
摘要
Introduction Epilepsy is a common and often highly morbid disease, associated with a not insignificant mortality. Some aspects of epilepsy have been well studied, for example the genetic basis and phenomenology of certain epilepsy syndromes, while other aspects such as long-term outcomes in large cohorts of epilepsy patients are less well studied. Mathematical modelling can assist in the analysis and interpretation of patterns and trends in long-term cohort data, that might not otherwise be obvious intuitively or using standard statistical methods. Methods Markov chain and state based modelling have been successfully used in analysing the progression of stages of disease in other specialties, such as chronic kidney disease and diabetic retinopathy. We applied this methodology to disease progression in epilepsy, by applying it to long-term cohort data. We used data from a longitudinal observational cohort study of 1795 patients with newly diagnosed epilepsy, for up to 30 years. The patients were managed in typical, real world clinical practice. Results We created a framework for conceptualising the natural history of epilepsy as a step-wise progression of disease through various stages, determined by seizure freedom and antiepileptic drug schedule. We adapted mathematical frameworks based on Markov chain and multi-state models to this model. We generated transition probabilities representing the dynamics of moving between stages of disease. This allowed us to analyse the dynamics of progression, and hence prognosis, in one of the largest and longest followed epilepsy cohorts in the world. Conclusion Epilepsy can be conceptualised as multi-stage, stepwise disease process. Markov and multistate models can be used to model the natural history of epilepsy, and inform us of the likely outcomes of epilepsy at the various stages in its progression. This quantification of outcomes can help us better inform patients of their prognosis at various stages in their illness.
更多查看译文
AI 理解论文
溯源树
样例
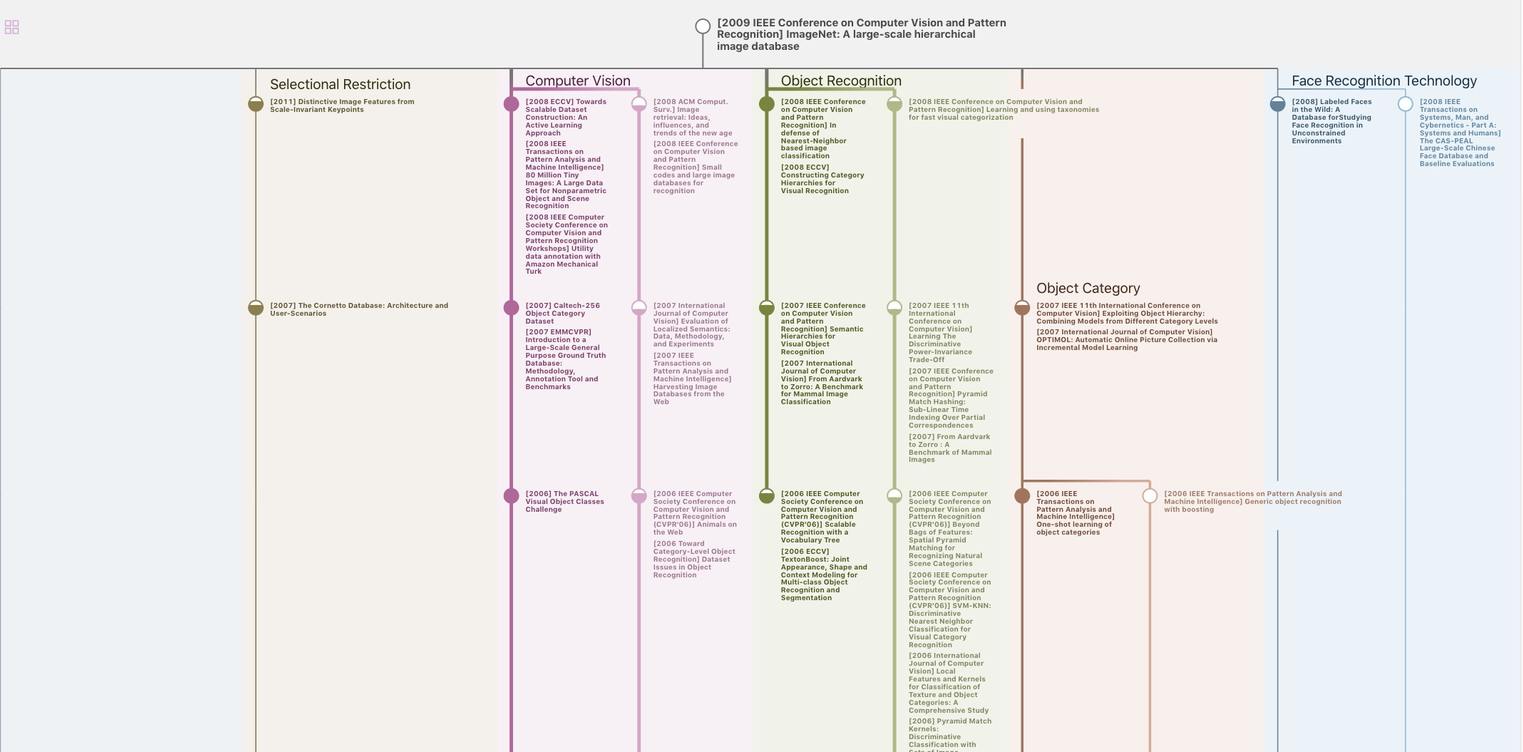
生成溯源树,研究论文发展脉络
Chat Paper
正在生成论文摘要