Adaptive Human-In-The-Loop Multi-Target Recognition Improved By Learning
INTERNATIONAL JOURNAL OF ADVANCED ROBOTIC SYSTEMS(2018)
摘要
Machine learning algorithms have been designed to address the challenge of multi-target recognition in dynamic and complex environments. However, sufficient high-quality samples are not always available for training an accurate multi-target recognition classifier. In this article, we propose a generic human-in-the-loop multi-target recognition framework that has four collaborative autonomy levels, and it allows adaptive autonomy level adjustment based on the recognition task complexity as well as the human operator's performance. The human operator can intervene to relabel the collected data and guarantee the recognition accuracy when the trained classifier is not good enough. Meanwhile, the relabeled data are used for online learning which further improves the performance of the classifier. Experiments have been carried out to validate the proposed approach.
更多查看译文
关键词
Multi-target recognition, human-in-the-loop surveillance, online learning, autonomy level adjustment
AI 理解论文
溯源树
样例
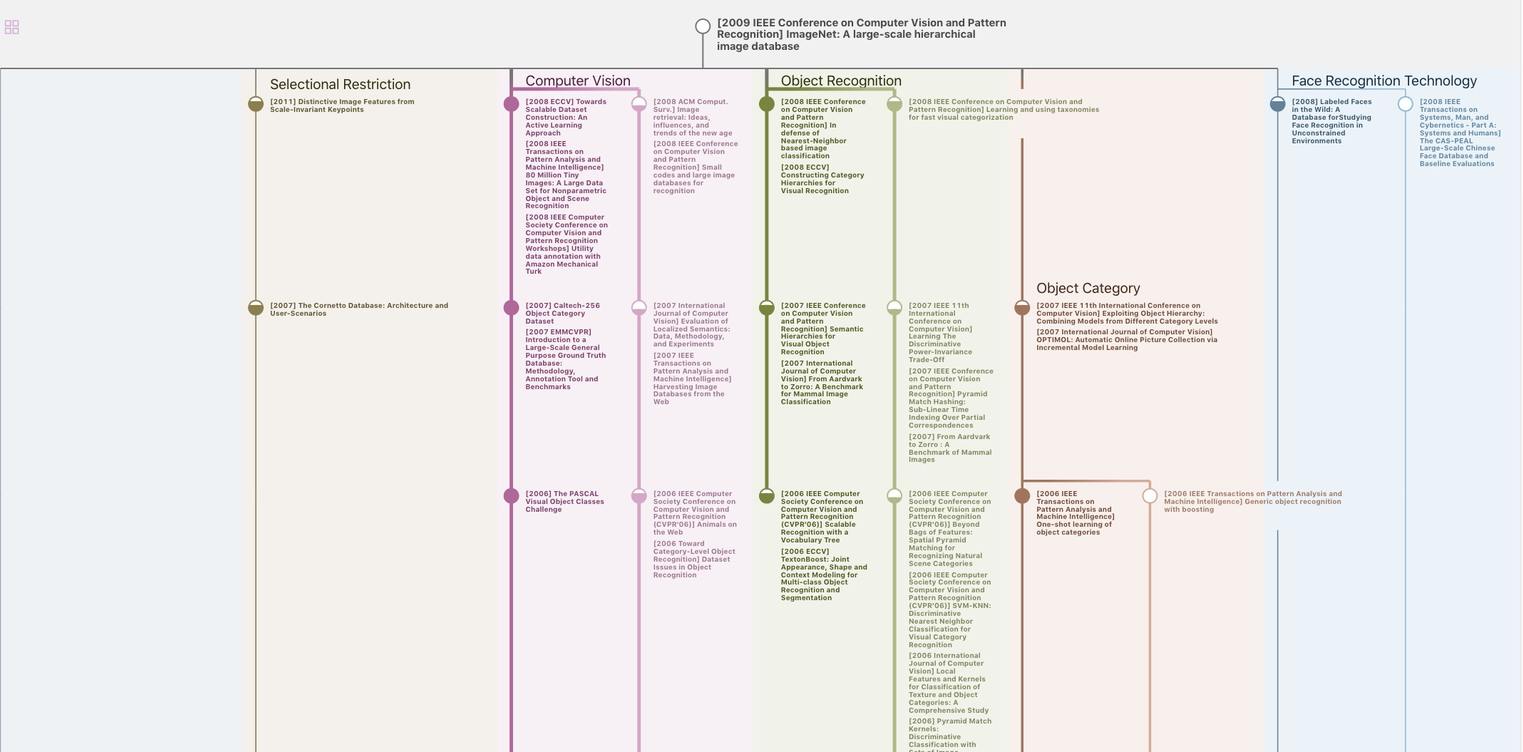
生成溯源树,研究论文发展脉络
Chat Paper
正在生成论文摘要