Atrial fibrillation classification using step-by-step machine learning
BIOMEDICAL PHYSICS & ENGINEERING EXPRESS(2018)
摘要
This paper presents a detailed overview of our submission to the 2017 Physionet Challenge where competitors were asked to build a model to classify a single lead ECG waveform as either normal sinus rhythm, atrial fibrillation, other rhythm, or noisy. A step-by-step machine learning pipeline was assembled, which included signal conditioning, R-peak detection and filtering, and feature extraction. Asuite of over 300 features, falling into one of three main feature groups; template features, RRI features, and full waveform features, were extracted from each waveform and an XGBoost, tree-based, gradient boosting classifier was used as the machine learning algorithm. The model produced a cross-validation F-1 score of 0.8245, a hidden sub-test score of 0.82, and a hidden test score of 0.8125. The score breakdown for each class (normal sinus rhythm, atrial fibrillation, other rhythm, and noisy) was as follows: F-1,F- NRS = 0.9024, F-1,F- AF = 0.8156, F-1,F- OR = 0.7194, F-1,F- Noise =. 0.5705.
更多查看译文
关键词
machine learning,signal processing,ECG Waveforms,physionet challenge
AI 理解论文
溯源树
样例
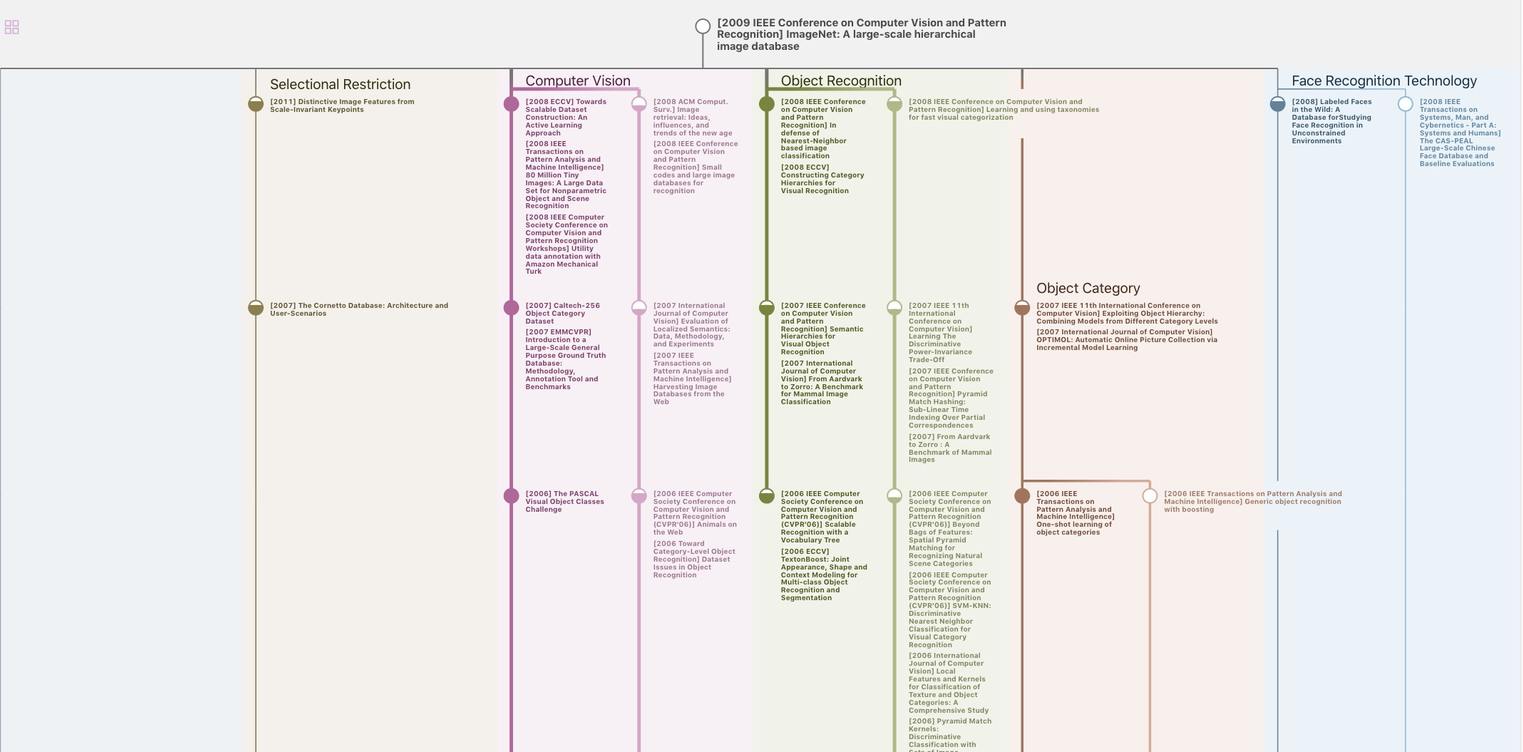
生成溯源树,研究论文发展脉络
Chat Paper
正在生成论文摘要