Gfrp Cutting Parameters Optimization Using Different Moeas
2017 INTERNATIONAL CONFERENCE ON INTELLIGENT COMPUTING, INSTRUMENTATION AND CONTROL TECHNOLOGIES (ICICICT)(2017)
摘要
In last few decades, optimization of multi-objective problems, especially with composite constraints, has become an important research area. Many evolutionary algorithms have been used in the literature to solve multi-objective problems. In this article, the cutting parameters optimization of Glass Fiber Reinforced Plastic (GFRP) machining has been studied as a multi-objective optimization problem. Here, the objective is to minimize the roughness of the surface and tool flank wear, and maximize the material removal rate. For this purpose, we have applied five different Multi-objective Evolutionary Algorithms (MOEAs): (i) Non-dominated Sorting Genetic AlgorithmII (NSGA-II) and (ii) Archive-based Steady-state Micro Genetic Algorithm (ASMiGA) based on GA, (iii) Archive based hybrid Scatter Search (AbYSS) based on scatter search, (iv) Generalized Differential Evolution 3 (GDE3) based on Differential Evolution (DE) and (v) Multi-objective Particle Swarm Optimization (MOPSO) based on Particle Swarm Optimization (PSO) to solve the cutting parameter optimization problem of GFRP. Performances of these algorithms have been evaluated and compared based on three performance metrics: (i) Hyper volume (HV), (ii) Inverted generalized distance (IGD) and (iii) Generalized spread (GS). Simulation results demonstrate the superiority of ASMiGA compared to other four standard evolutionary algorithms for cutting parameters optimization of GFRP.
更多查看译文
关键词
AbYSS, ASMiGA, GDE3, GFRP, MOPSO, multi-objective optimization, NSGA-II
AI 理解论文
溯源树
样例
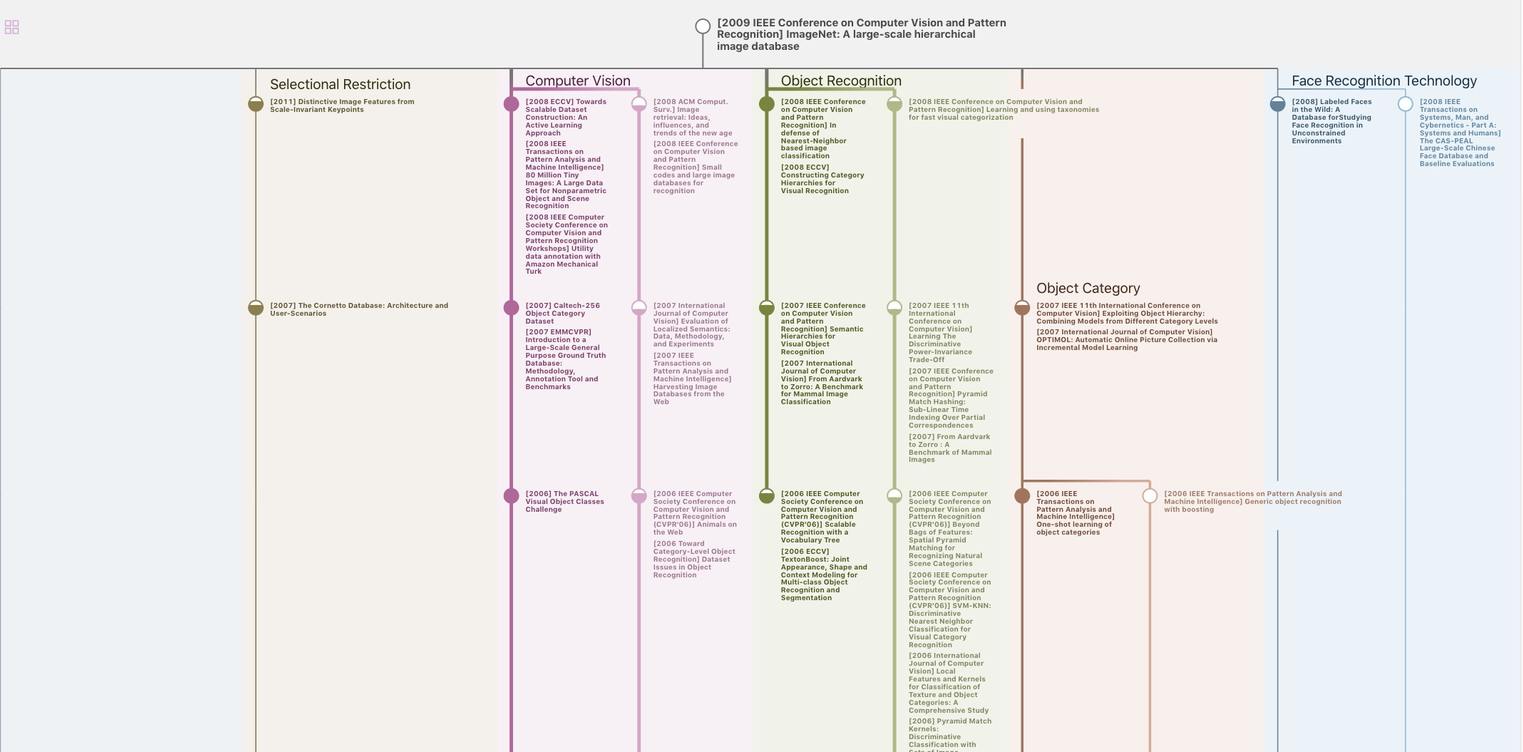
生成溯源树,研究论文发展脉络
Chat Paper
正在生成论文摘要