Pass-Efficient Randomized Algorithms for Low-Rank Matrix Approximation Using Any Number of Views
SIAM JOURNAL ON SCIENTIFIC COMPUTING(2019)
摘要
This paper describes practical randomized algorithms for low-rank matrix approximation that accommodate any budget for the number of views of the matrix. The presented algorithms, which are intended to be as pass efficient as needed, expand and improve on popular randomized algorithms targeting efficient low-rank reconstructions. First, a more flexible subspace iteration algorithm is presented that works for any views v >= 2, instead of only allowing an even v. Second, we propose more general and more accurate single-pass algorithms. In particular, we propose a more accurate memory efficient single-pass method and a more general single-pass algorithm which, unlike previous methods, does not require prior information to ensure near peak performance. Third, combining ideas from subspace and single-pass algorithms, we present a more pass-efficient randomized block Krylov algorithm, which can achieve a desired accuracy using considerably fewer views than that needed by a subspace or previously studied block Krylov methods. However, the proposed accuracy enhanced block Krylov method is restricted to large matrices that are accessed either a few columns or a few rows at a time. Recommendations are also given on how to apply the subspace and block Krylov algorithms either when estimating the dominant left or right singular subspace of a matrix or when estimating a normal matrix, such as those appearing in inverse problems. Computational experiments are carried out that demonstrate the applicability and effectiveness of the presented algorithms.
更多查看译文
关键词
singular value decomposition,random,low rank,subspace iteration,single pass,block Krylov
AI 理解论文
溯源树
样例
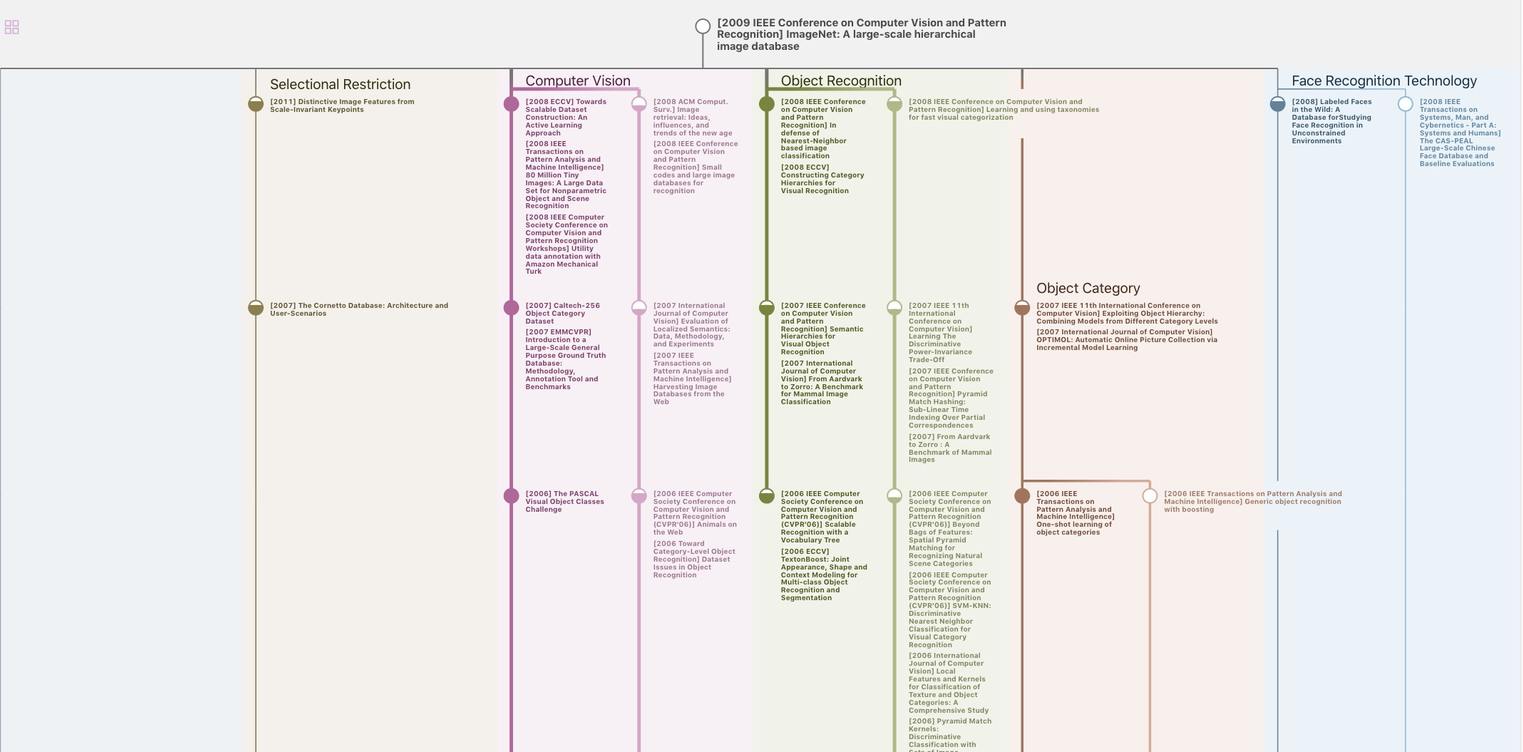
生成溯源树,研究论文发展脉络
Chat Paper
正在生成论文摘要