Prognostic models for Ebola virus disease derived from data collected at five treatment units in Sierra Leone and Liberia: performance, external validation, and risk visualization
bioRxiv(2019)
摘要
Background: The 2014-2016 Ebola Virus Disease (EVD) outbreak highlighted the need for rigorous, rapid, and field-deployable tools to enable case management. We previously introduced an approach for EVD prognosis prediction, using models that can be implemented in the field and updated in light of new data. Here we enhance and validate our methods with the largest published EVD dataset to date. We also present a proof-of-concept medical app that summarizes patient information and offers tailored treatment options using an interactive risk visualization for quick interpretation and decision-making. Methods and Findings: We derived prognosis prediction models for EVD using data from 470 patients admitted to five Ebola treatment units (ETUs) operated by International Medical Corps (IMC) in Liberia and Sierra Leone. We fitted logistic regression models, handled missing data by multiple imputation, and conducted internal validation with bootstrap sampling. We also validated our models with independent datasets from two treatment centers in Sierra Leone comprising 106 patients at Kenema Government Hospital and 158 patients at the GOAL-Mathaska ETU in Port Loko district. We corroborated earlier reports on the importance of viral load and age as mortality predictors and identified jaundice and bleeding to be features with highest predictive value at presentation. Additional clinical symptoms at presentation, although individually weakly correlated with outcome, help broaden sensitivity and refine discrimination of the models. The app provides a visual representation of the predictive outcome as well as attributing clinical protocols adjusted by demographic parameters and prioritized to target the largest contributing factor to overall risk. Conclusions: We derived and validated high performance models of EVD prognosis prediction from the largest and most geographically diverse EVD patients available to date. The performance was maintained during external validations on two independent datasets representing different treatment settings and mortality rates, which suggests that the models could be generalized to new populations. This tool may better inform treatment choices in future EVD outbreaks. It also provides a template to validate additional datasets used in developing novel clinical-decision support systems for EVD and other emerging infectious diseases.
更多查看译文
关键词
Ebola Virus Disease,Prognostic Models,Machine Learning,Data Visualization,Severity Score,mHealth,Supportive Care Guidelines,Clinical Intuition
AI 理解论文
溯源树
样例
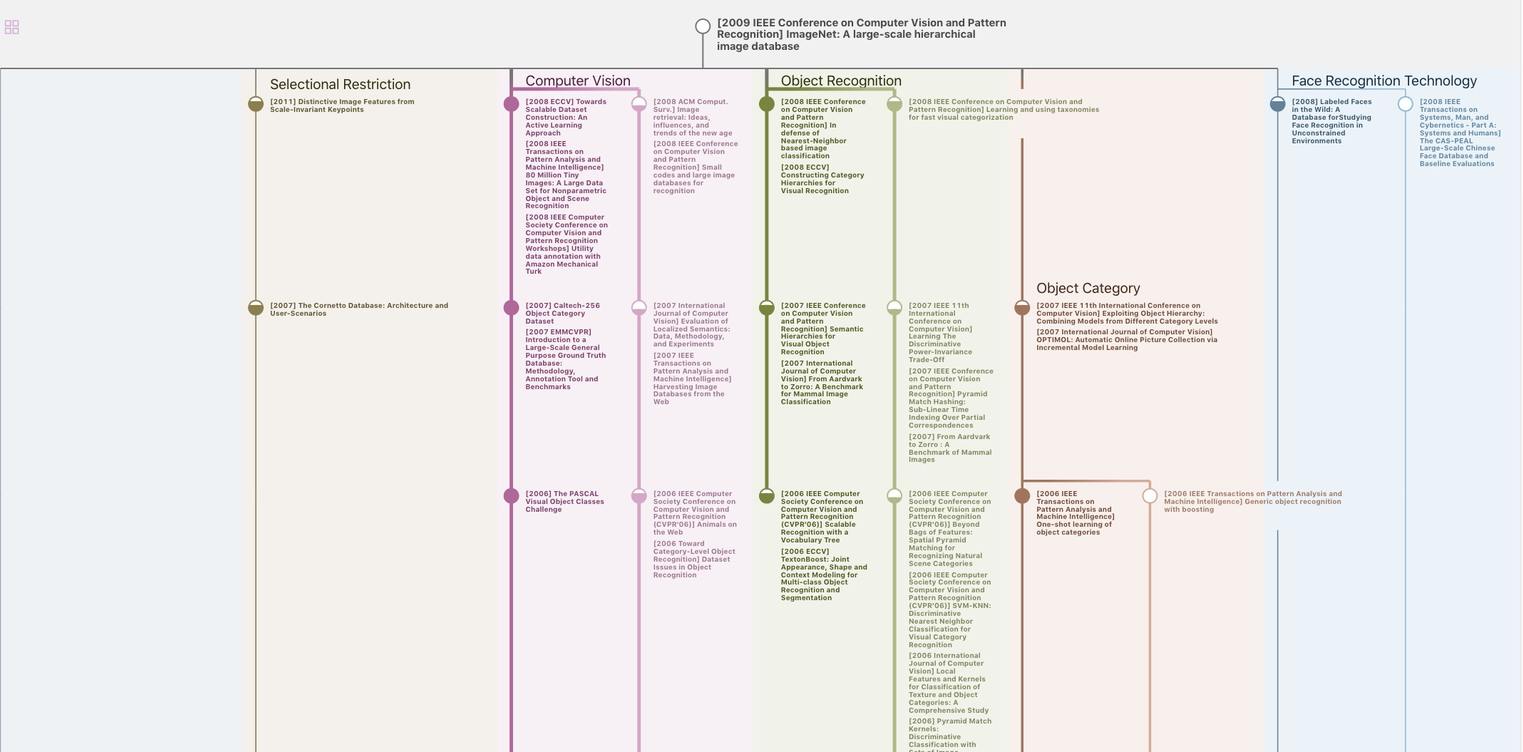
生成溯源树,研究论文发展脉络
Chat Paper
正在生成论文摘要