MP01-07 USE OF COMPUTER VISION MOTION ANALYSIS TO AID IN SURGICAL SKILL ASSESSMENT OF SUTURING TASKS
The Journal of Urology(2018)
Abstract
You have accessJournal of UrologySurgical Technology & Simulation: Training & Skills Assessment I1 Apr 2018MP01-07 USE OF COMPUTER VISION MOTION ANALYSIS TO AID IN SURGICAL SKILL ASSESSMENT OF SUTURING TASKS Brady Miller, David Azari, Robert Radwin, and Brian Le Brady MillerBrady Miller More articles by this author , David AzariDavid Azari More articles by this author , Robert RadwinRobert Radwin More articles by this author , and Brian LeBrian Le More articles by this author View All Author Informationhttps://doi.org/10.1016/j.juro.2018.02.113AboutPDF ToolsAdd to favoritesDownload CitationsTrack CitationsPermissionsReprints ShareFacebookTwitterLinked InEmail INTRODUCTION AND OBJECTIVES Marker-less video motion analysis can quantify kinematic properties of simulated operative tasks without need for expert review which can be time-consuming and subjective. Here, we assess the feasibility of this technology in the surgical simulation setting and compare hand summary kinematics with a standardized self-assessment using two simulated tasks. METHODS Medical students, residents, attending and retired surgeons completed simulated simple interrupted and running subcuticular suturing tasks. Performance was self-rated using previously tested visual analog motion scales. Digital cameras were positioned to record hand motions at 30 frames/sec without markers. Video analysis utilized a semi-supervised cross correlation template matching algorithm, producing an x-y pixel location of the participant’s dominant hand across successive video frames, enabling the kinematic parameters speed, acceleration and jerk to be calculated. RESULTS Participants (n=35) were recorded performing both tasks (n=70 observations), totaling 5.2 hours (avg 4:45 min/video) video time. The two most common sources of video artifact requiring analyst adjustment were (1) drift of hands outside the frame and (2) transient obscuring of the frame by participant's head. The time required to supervise the tracking algorithm varied greatly, ranging from 3 to 10 times the original segment length. Despite some tracking artifact, kinematic parameters were calculated for all (100%) observations. Mean acceleration was greater for residents (631.1 mm/sec^2) than attendings (577.8), students (563.9) and retirees (471.2), though was not statistically different (p=0.32). Mean speed and jerk index were also greater for residents than others but also not statistically different (p=0.27). Self-coordination rating was weakly correlated with speed (r=0.24, p=0.04), acceleration (r=0.31, p=0.01) and jerk (r=0.31, p=0.01). CONCLUSIONS Marker-less video motion analysis successfully tracked hand movement and represents a potentially high-throughput tool with on-demand availability to provide objective feedback. Some difference by experience level was suggested that may be accentuated with increasingly difficult tasks. Future work should focus reducing need for manual adjustment. © 2018FiguresReferencesRelatedDetails Volume 199Issue 4SApril 2018Page: e4 Advertisement Copyright & Permissions© 2018MetricsAuthor Information Brady Miller More articles by this author David Azari More articles by this author Robert Radwin More articles by this author Brian Le More articles by this author Expand All Advertisement Advertisement PDF downloadLoading ...
MoreTranslated text
Key words
surgical skill assessment,computer vision motion analysis,tasks
AI Read Science
Must-Reading Tree
Example
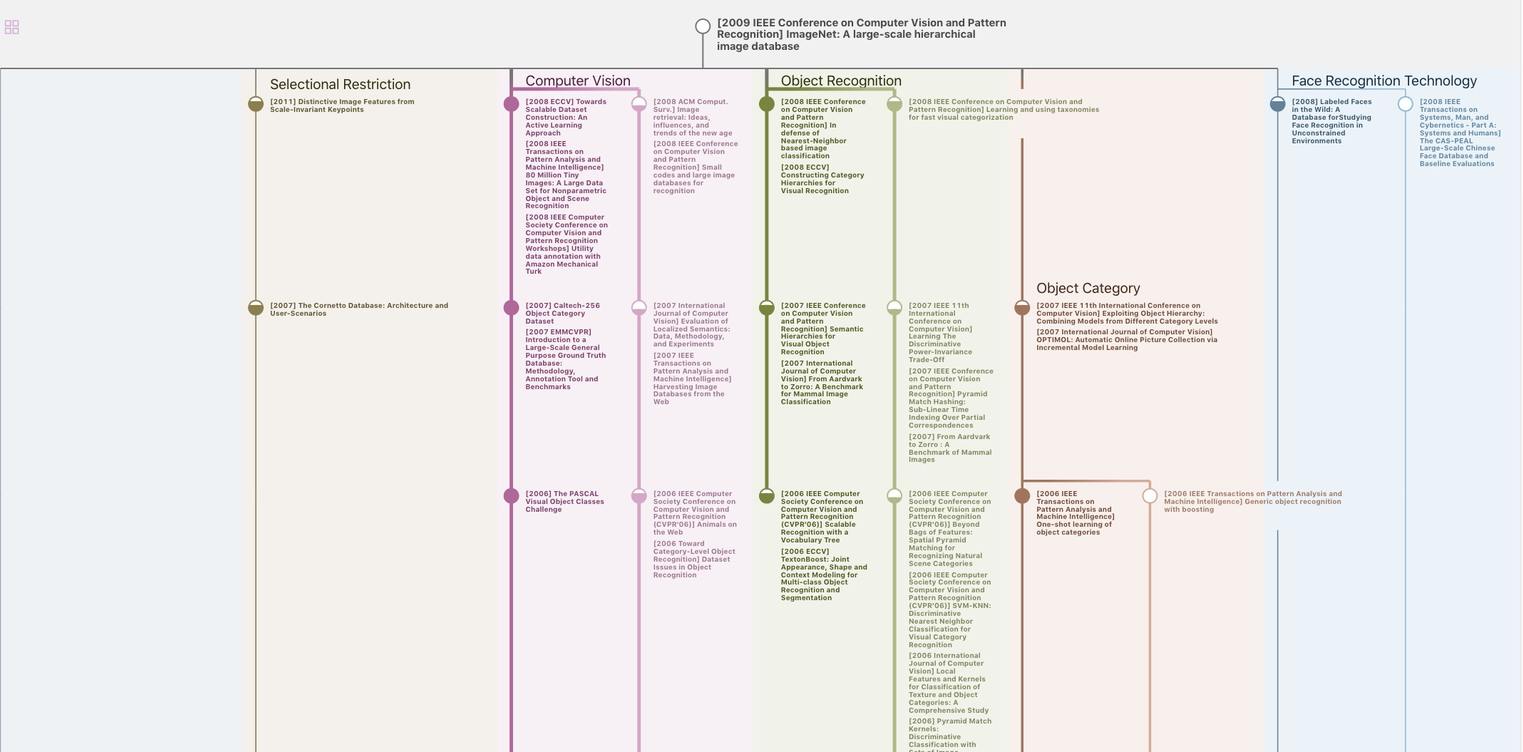
Generate MRT to find the research sequence of this paper
Chat Paper
Summary is being generated by the instructions you defined