Robust Object Tracking Based On Collaborative Sparse Representation Of Multifeature
PROCEEDINGS OF 2017 INTERNATIONAL CONFERENCE ON ROBOTICS AND ARTIFICIAL INTELLIGENCE (ICRAI 2017)(2015)
摘要
It is inefficient when the target changes in pose variation, scale and partial occlusion. In this paper, a new target tracking algorithm with collaborative sparse representation of multifeature is proposed, which is based on the particle filter framework and sparse representation. Firstly, in the construction of the target template, both the fusion feature and HAAR features are used to describe the target, the appearance model of the tracked target is modeled by instantaneous and stable appearance features simultaneously. Then, a two-stage sparse-coded method which takes the spatial neighborhood information of the image patch and the computation burden into consideration is used to construct the tracking likelihood function of transient and stable appearance. Finally, the reliability of each tracker is measured by the tracking likelihood function, and the most reliable tracker is obtained by a well established particle filter framework. The templates library are incrementally updated based on the current tracking results. Experimental results show that the proposed method is robust to pose variation, scale change and partial occlusion.
更多查看译文
关键词
sparse representation, multifeature fusion, target tracking
AI 理解论文
溯源树
样例
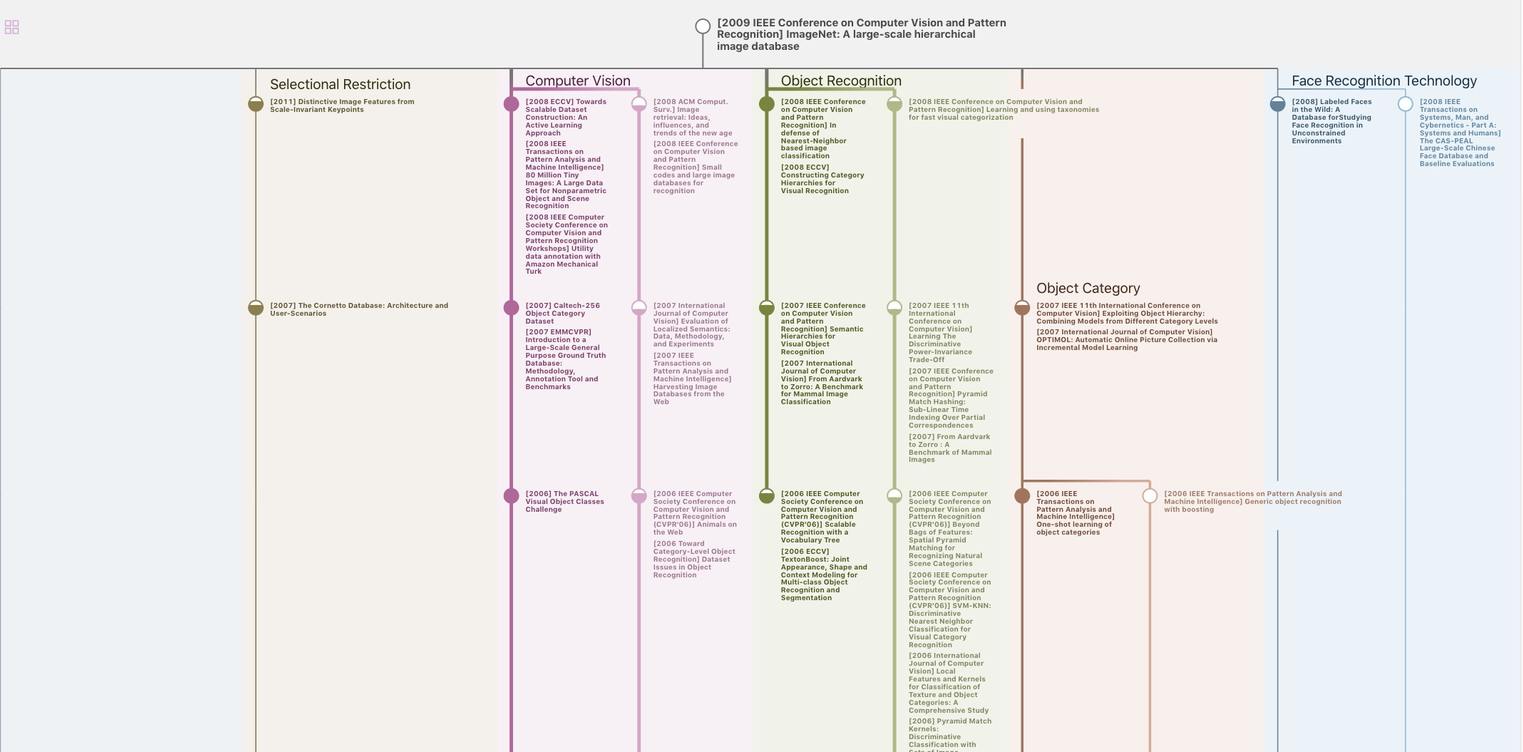
生成溯源树,研究论文发展脉络
Chat Paper
正在生成论文摘要