Estimating Propensity Scores for the Receipt of Allogeneic Hematopoietic Cell Transplantation (AlloHCT) in Outcomes Research Using Claims Data: A Machine Learning Approach
BIOLOGY OF BLOOD AND MARROW TRANSPLANTATION(2018)
摘要
Background: Careful analysis of claims data can yield policy-relevant evidence about the real-world cost and outcomes of AlloHCT. Accounting for treatment self-selection is critical to obtain unbiased comparisons between AlloHCT and other treatments. Propensity scores can be used to adjust for observed confounders that affect both treatment selection and outcomes. While logistic regression (logit) is the standard method for estimating propensity scores, machine learning may predict treatment selection more accurately, particularly when there are many possible predictors relative to observations or when interactions of predictors may be important but would be difficult to pre-specify. Methods: We defined a cohort of patients aged 18-74 years with acute myelogenous leukemia (AML) who received AlloHCT (N = 278) or chemotherapy only (N = 570), using Optum Clinformatics data (2004-2014). Both logit and stochastic tree-based extreme gradient boosting (R package xgboost) were used to predict receipt of AlloHCT conditional on age, gender, diagnosis year, region, time from diagnosis to chemotherapy initiation, insurer (commercial vs. Medicare), insurance plan type (e.g., HMO, PPO, etc.), Elixhauser Comorbidity Index (ECI) and comorbidity indicators used to construct the ECI that were present in ≥3% of patients observed in the 2 months before diagnosis (hypertension, diabetes, coagulopathy, electrolyte imbalance, and anemia). Learning parameters were tuned using 20-fold cross-validation to control over-fitting. We compared prediction residuals and balancing properties of standardized inverse propensity weights derived using both approaches. Results: The logit model found that patients who were older, had Medicare, or had a shorter time from diagnosis to chemotherapy initiation were less likely to receive AlloHCT (P < .05). With boosting, the same predictors were among the top four in improving prediction accuracy; however, diagnosis year produced larger accuracy gains than having Medicare. Boosting produced a lower mean-squared error (.164 vs. .200) and prediction residuals with higher density near zero, indicating more accurate propensity scores than logit (Figure 1). Standardized inverse propensity weights constructed using both approaches achieved covariate balance, with no variable exceeding Cohen's .2 threshold for a “small” absolute standardized difference (Figure 2).Figure 2Standardized differences in predictors (AlloHCT - Chemotherapy Only)View Large Image Figure ViewerDownload Hi-res image Download (PPT) Conclusion: Propensity scores constructed with boosting more accurately predicted receipt of AlloHCT than those derived from logit while producing comparably-balanced weighted datasets. While neither approach accounts for unobserved confounders, machine-learning can better predict treatment choice by modeling complex patterns of predictor variables, and consequently may improve inference about the comparative effectiveness and cost of AlloHCT.
更多查看译文
关键词
allogeneic hematopoietic cell transplantation,propensity scores,outcomes
AI 理解论文
溯源树
样例
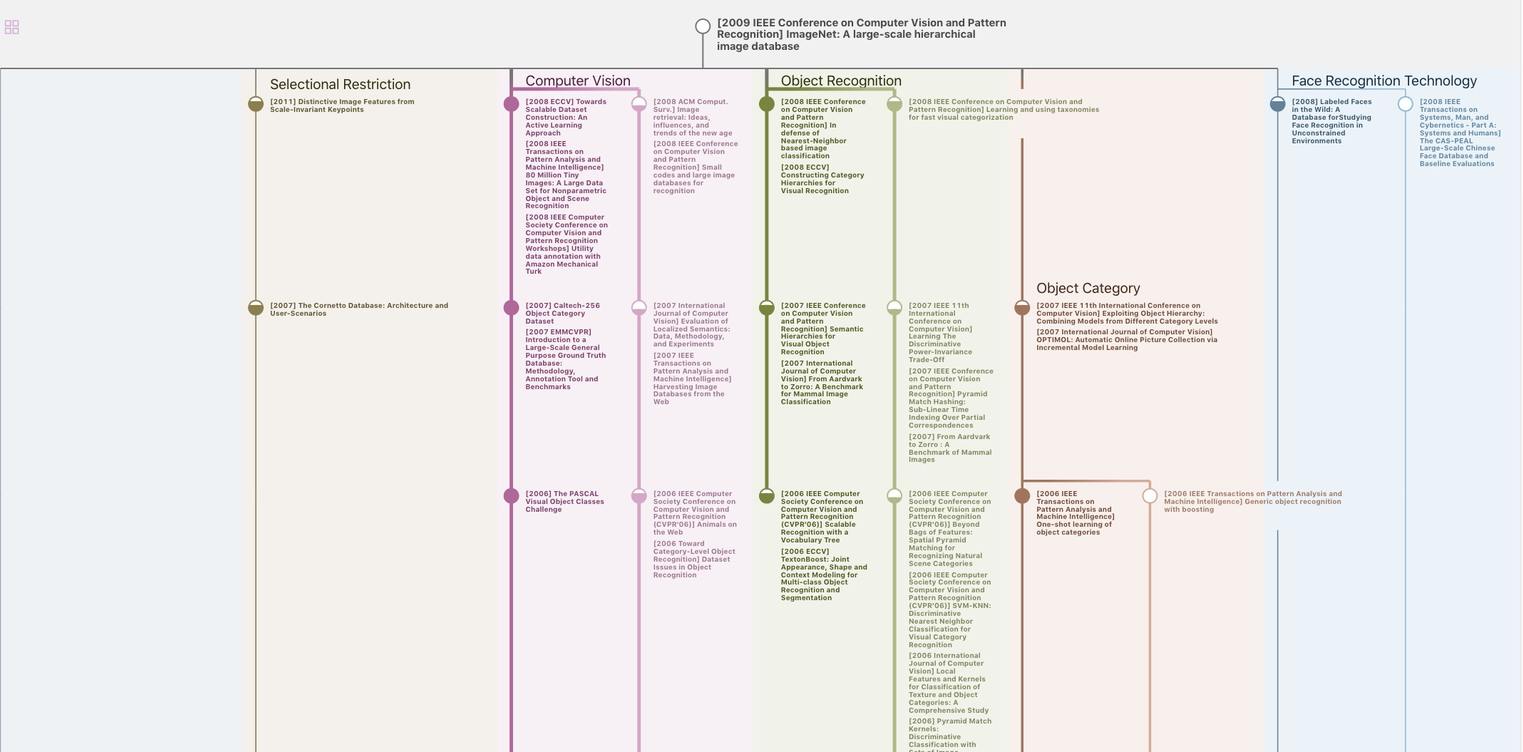
生成溯源树,研究论文发展脉络
Chat Paper
正在生成论文摘要