Comprehensive Evaluation of Machine Learning Techniques for Estimating the Responses of Carbon Fluxes to Climatic Forces in Different Terrestrial Ecosystems
ATMOSPHERE(2018)
摘要
Accurately estimating the carbon budgets in terrestrial ecosystems ranging from flux towers to regional or global scales is particularly crucial for diagnosing past and future climate change. This research investigated the feasibility of two comparatively advanced machine learning approaches, namely adaptive neuro-fuzzy inference system (ANFIS) and extreme learning machine (ELM), for reproducing terrestrial carbon fluxes in five different types of ecosystems. Traditional artificial neural network (ANN) and support vector machine (SVM) models were also utilized as reliable benchmarks to measure the generalization ability of these models according to the following statistical metrics: coefficient of determination (R-2), index of agreement (IA), root mean square error (RMSE), and mean absolute error (MAE). In addition, we attempted to explore the responses of all methods to their corresponding intrinsic parameters in terms of the generalization performance. It was found that both the newly proposed ELM and ANFIS models achieved highly satisfactory estimates and were comparable to the ANN and SVM models. The modeling ability of each approach depended upon their respective internal parameters. For example, the SVM model with the radial basis kernel function produced the most accurate estimates and performed substantially better than the SVM models with the polynomial and sigmoid functions. Furthermore, a remarkable difference was found in the estimated accuracy among different carbon fluxes. Specifically, in the forest ecosystem (CA-Obs site), the optimal ANN model obtained slightly higher performance for gross primary productivity, with R-2 = 0.9622, IA = 0.9836, RMSE = 0.6548 g C m(-2) day(-1), and MAE = 0.4220 g C m(-2) day(-1), compared with, respectively, 0.9554, 0.9845, 0.4280 g C m(-2) day(-1), and 0.2944 g C m(-2) day(-1) for ecosystem respiration and 0.8292, 0.9306, 0.6165 g C m(-2) day(-1), and 0.4407 g C m(-2) day(-1) for net ecosystem exchange. According to the findings in this study, we concluded that the proposed ELM and ANFIS models can be effectively employed for estimating terrestrial carbon fluxes.
更多查看译文
关键词
terrestrial ecosystems,climate change,carbon fluxes,flux towers,machine learning techniques
AI 理解论文
溯源树
样例
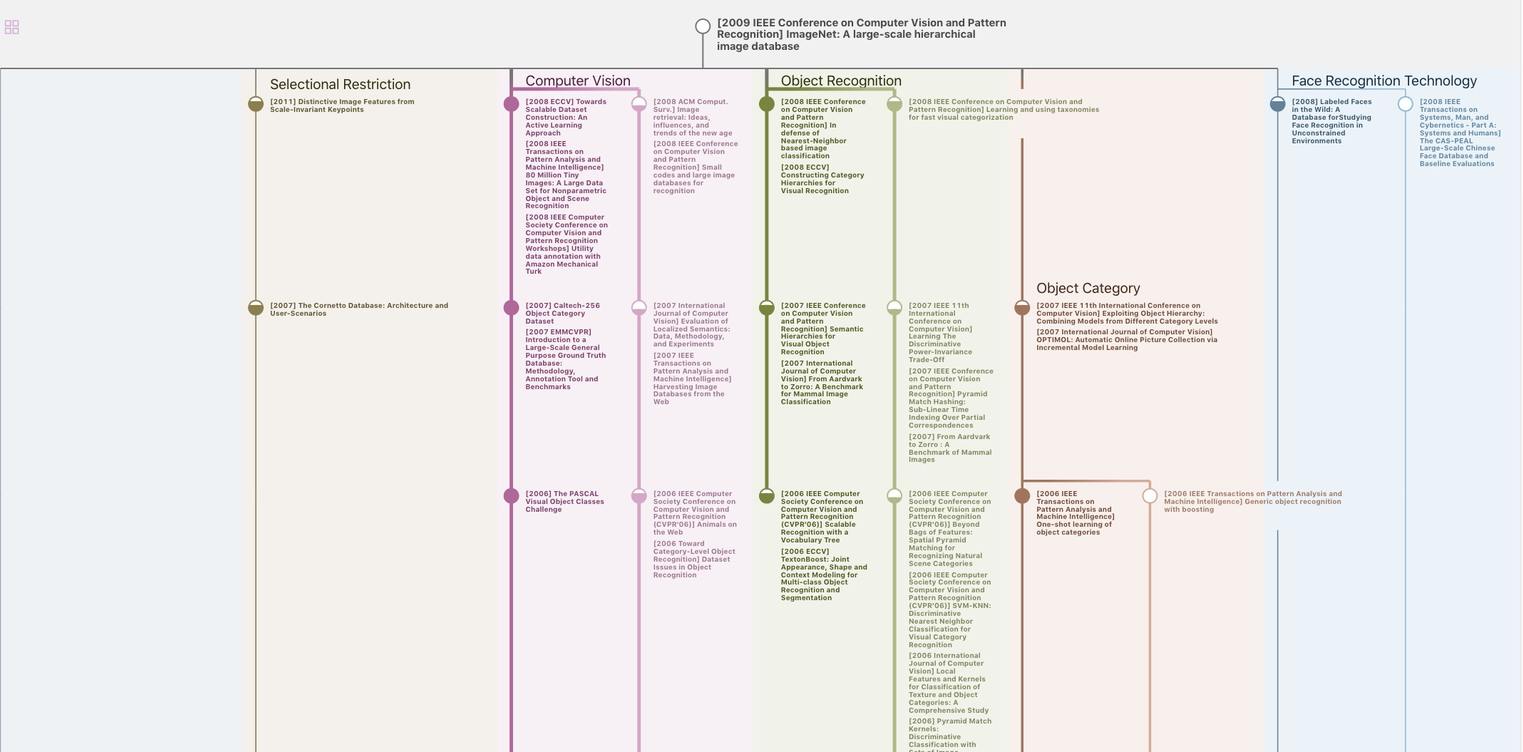
生成溯源树,研究论文发展脉络
Chat Paper
正在生成论文摘要