A machine learning approach for pixel wise classification of residue and vegetation cover under field conditions
Biosystems Engineering(2018)
摘要
Soil cover is a crucial factor for sustainable cultivation of arable land. A certain degree of residue and vegetation cover reduces erosion significantly and has positive effects on plant development. In order to accomplish these positive effects, it is necessary to measure and control the amount of soil cover on fields. Manual measurement methods are time consuming and/or subjective. Available image analysis methods often lack of generalisation and accuracy. Many approaches only focus on residue or on vegetation cover and do not consider different camera hardware. Recent advancements in machine learning techniques are promising to overcome these issues. The proposed method, the entangled random forest, a variant of a random decision forest, classifies individual pixels into soil, residue, living plants and stones. Simple and efficient pixel-wise comparisons to neighbouring pixels are integrated as decision-features into the random forest. To validate our method, the result of the automatic classification was compared with results of manual classifications from evaluators on image grid points. The classification of soil results in a regression equation between the results of the new introduced method and a manual image classification of y = 0.99x + 2.02 (R-2 = 0.93). Living plant classification results in a regression between both methods in y = 0.94x 0.70 with (R-2 = 0.98) and for dead residues in y = 1.04x 0.64 (R-2 = 0.84). It is possible to access a demo of the algorithm by using a web and a mobile application on https://soilcover.josephinum.at. (C) 2018 IAgrE. Published by Elsevier Ltd. All rights reserved.
更多查看译文
关键词
2D image classification,Random forest,Soil cover estimation
AI 理解论文
溯源树
样例
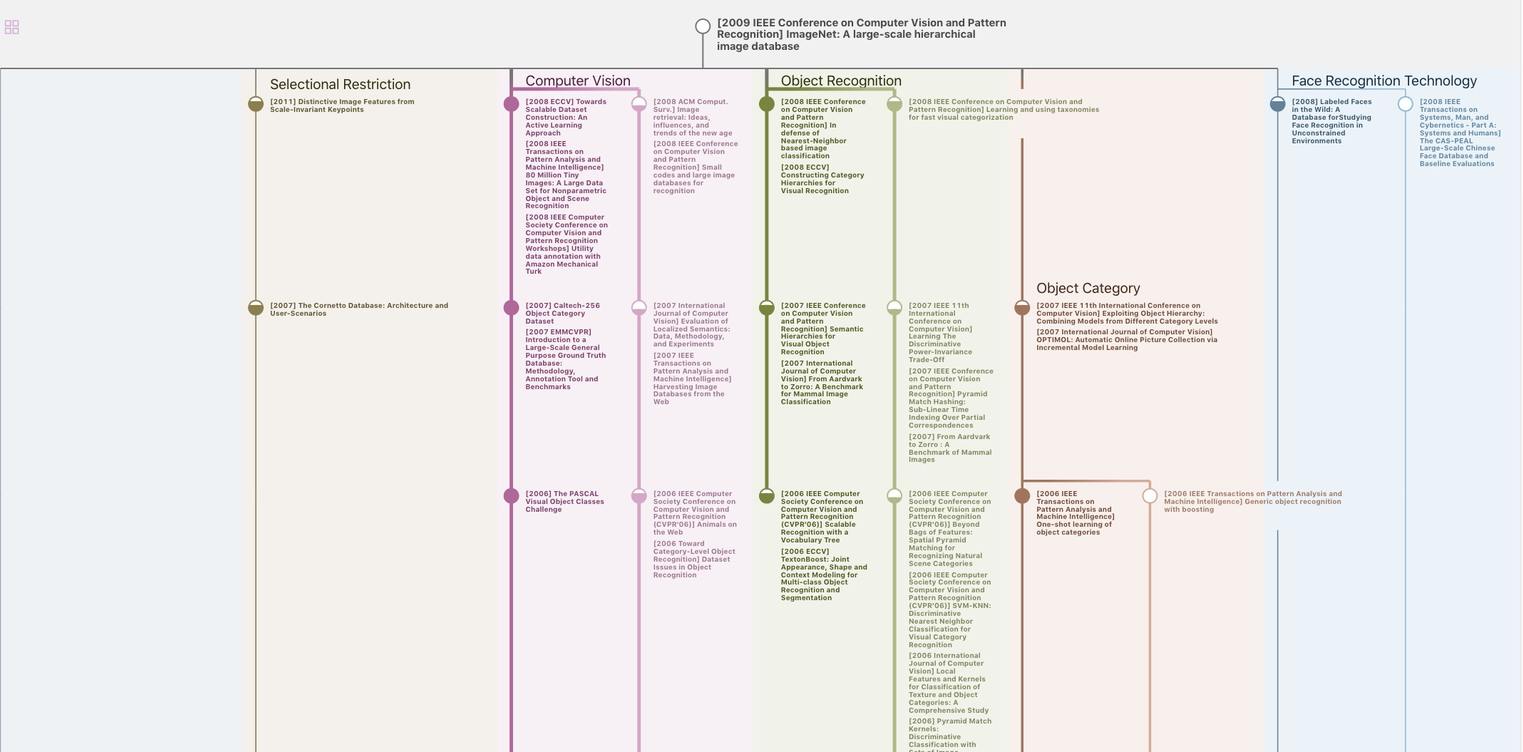
生成溯源树,研究论文发展脉络
Chat Paper
正在生成论文摘要