DensityPath: a level-set algorithm to visualize and reconstruct cell developmental trajectories for large-scale single-cell RNAseq data
bioRxiv(2018)
摘要
Cell fates are determined by transition-states which occur during complex biological processes such as proliferation and differentiation. The advance in single-cell RNA sequencing (scRNAseq) provides the snapshots of single cell transcriptomes, thus offering an essential opportunity to study such complex biological processes. Here, we introduce a novel algorithm, DensityPath, which visualizes and reconstructs the underlying cell developmental trajectories for large-scale scRNAseq data. DensityPath has three merits. Firstly, by adopting the nonlinear dimension reduction algorithm elastic embedding, DensityPath reveals the intrinsic structures of the data. Secondly, by applying the powerful level set clustering method, DensityPath extracts the separate high density clusters of representative cell states (RCSs) from the single cell multimodal density landscape of gene expression space, enabling it to handle the heterogeneous scRNAseq data elegantly and accurately. Thirdly, DensityPath constructs cell state-transition path by finding the geodesic minimum spanning tree of the RCSs on the surface of the density landscape, making it more computationally efficient and accurate for large-scale dataset. The cell state-transition path constructed by DensityPath has the physical interpretation as the minimum-transition-energy (least-cost) path. We demonstrate that DensityPath is capable of identifying complex cell development trajectories with bifurcating and trifurcating branches on the human preimplantation embryos. We demonstrate that DensityPath is robust and has high accuracy of pseudotime calculation and branch assignment on the real scRNAseq as well as simulated datasets.
更多查看译文
AI 理解论文
溯源树
样例
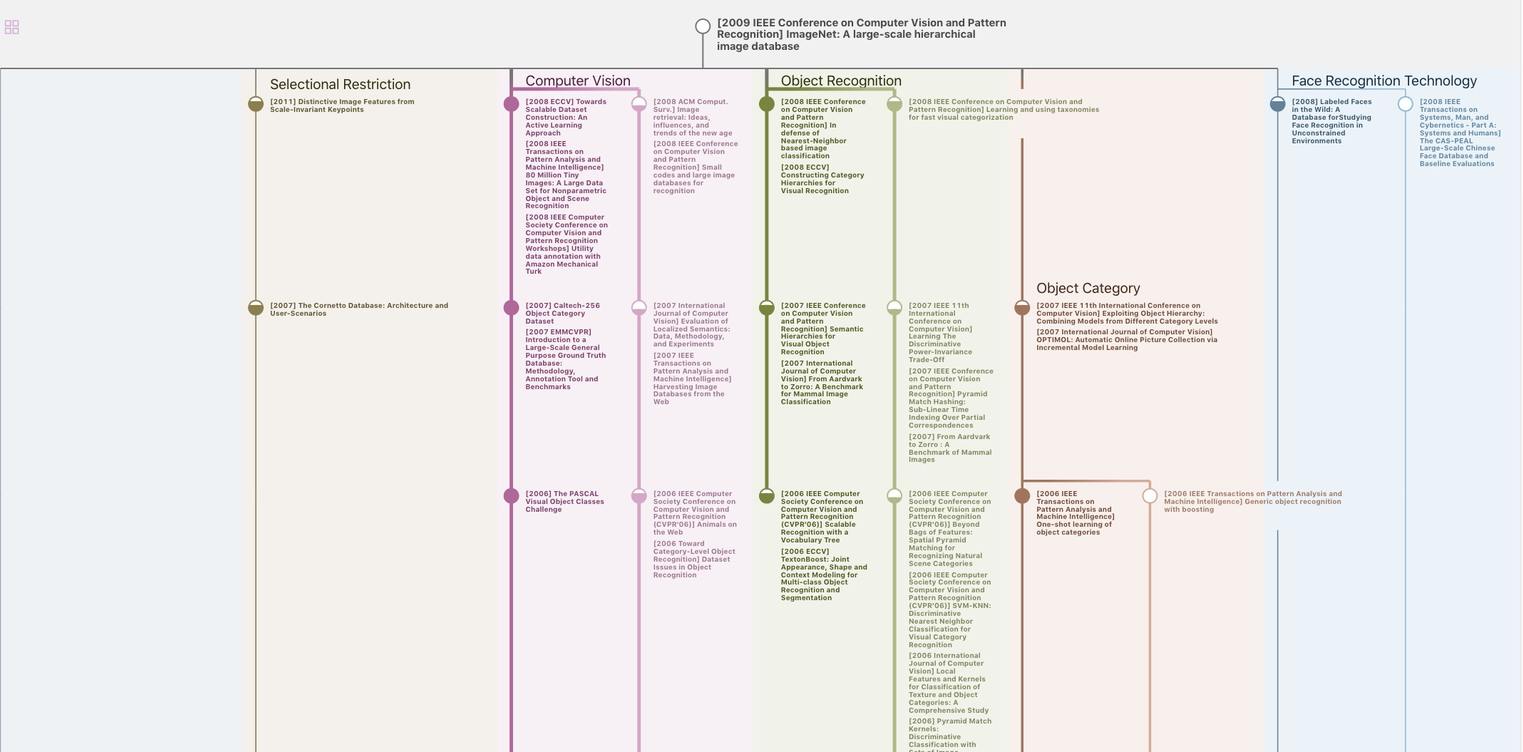
生成溯源树,研究论文发展脉络
Chat Paper
正在生成论文摘要