Tree species classification in Norway from airborne hyperspectral and airborne laser scanning data
EUROPEAN JOURNAL OF REMOTE SENSING(2018)
Abstract
This article compares four new automatic methods to discriminate between spruce, pine and birch, which are the dominating tree species in Norwegian forests. Airborne laser scanning and hyperspectral data were used. The laser scanning data was used to mask pixels with low or no vegetation in the hyperspectral data. A green-blue ratio was used to remove shadow areas from tree canopies, and the normalized difference vegetation index to remove dead vegetation and non-vegetation. The best method was hyperspectral pixel classification with 160 spectral channels in the visible and near-infrared spectrum, using a deep neural network. This method achieved 87% correct classification rate. Partial least squares regression for hyperspectral pixel classification achieved 78%. Deep neural network image classification using canopy height blended with three hyperspectral channels achieved 74%. A simple pixel classification method based on two spectral indices resulted in 67% correct classification. A possible future improvement is to find a better way to combine hyperspectral data with canopy height data in a deep neural network.
MoreTranslated text
Key words
Lidar,imaging spectroscopy,canopy height model,automatic processing,forestry,deep learning
AI Read Science
Must-Reading Tree
Example
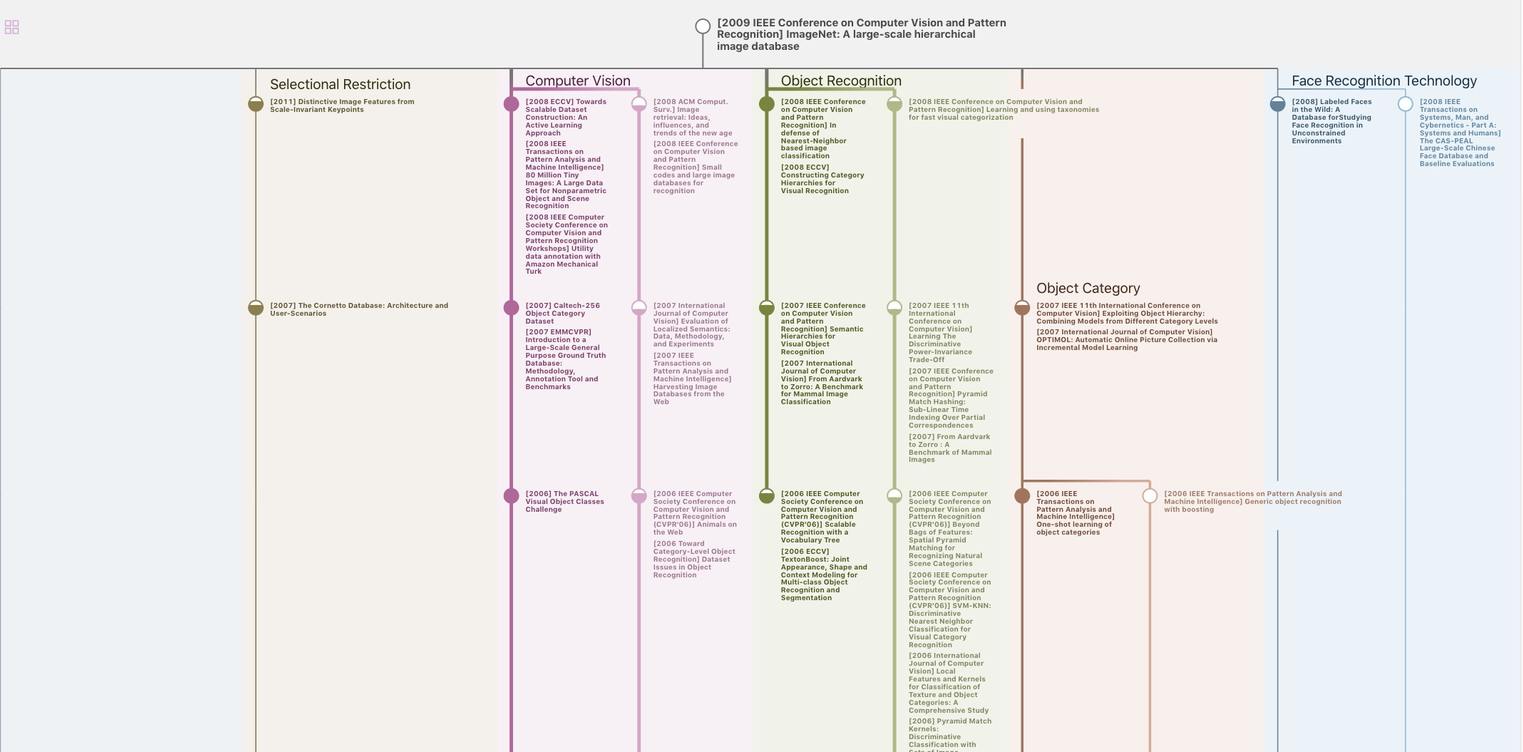
Generate MRT to find the research sequence of this paper
Chat Paper
Summary is being generated by the instructions you defined