Nonlinear Tikhonov Regularization In Hilbert Scales With Balancing Principle Tuning Parameter In Statistical Inverse Problems
INVERSE PROBLEMS IN SCIENCE AND ENGINEERING(2019)
摘要
In this article, we study the balancing principle for Tikhonov regularization in Hilbert scales for deterministic and statistical nonlinear inverse problems. While the rates of convergence in deterministic setting is order optimal, they prove to be order optimal up to a logarithmic term in the stochastic framework. The two-step approach allows us to consider a data-driven algorithm in a general error model for which an exponential behaviour of the tail of the estimator chosen in the first step is valid. Finally, we compute the overall rate of convergence for a Hammerstein operator equation and for a parameter identification problem. Moreover, we illustrate these rates for the last application after we study some large sample properties of the local polynomial estimator in a general stochastic framework.
更多查看译文
关键词
Statistical inverse problem, nonlinear Tikhonov regularization, Hilbert scales, balancing principle, Primary: 65J20, Secondary: 47N20
AI 理解论文
溯源树
样例
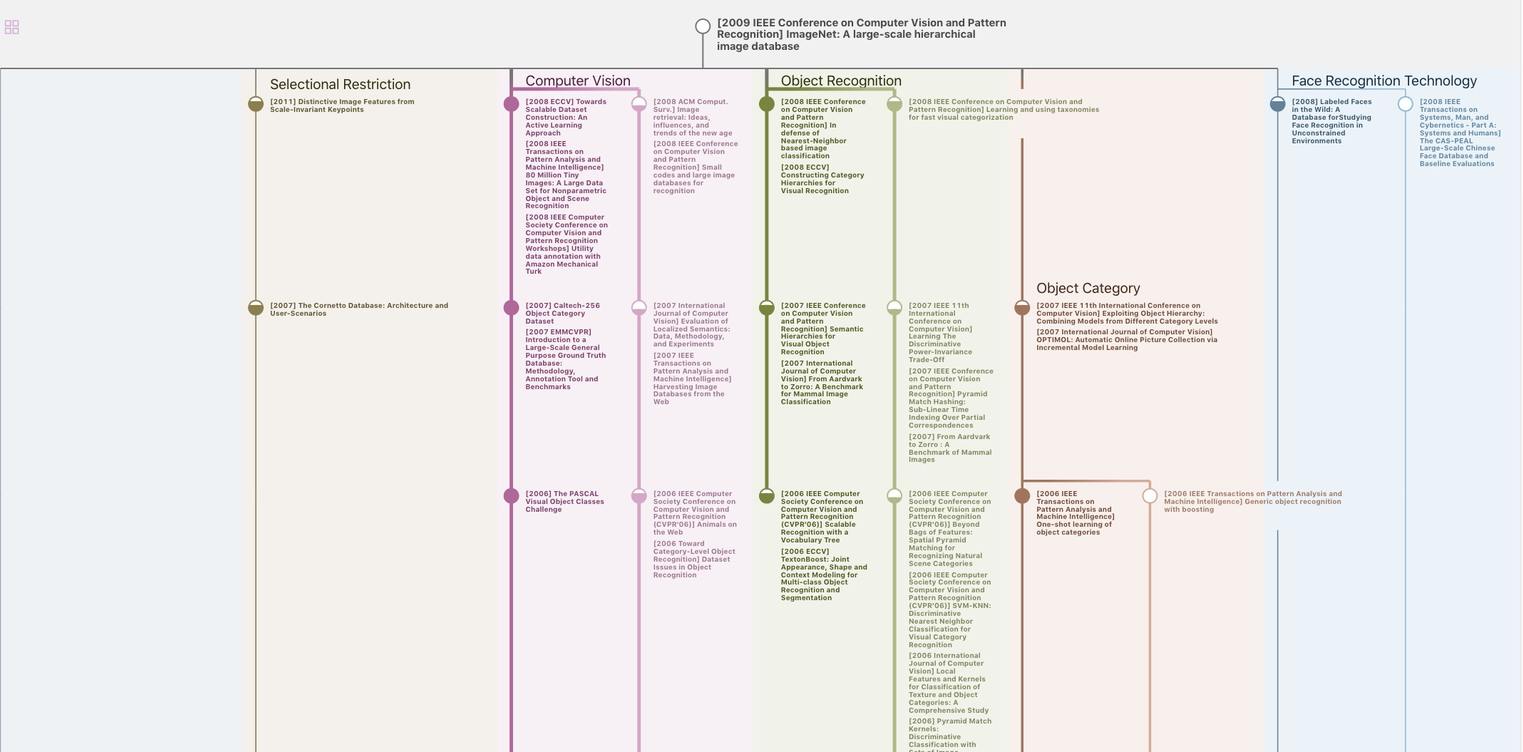
生成溯源树,研究论文发展脉络
Chat Paper
正在生成论文摘要