A hidden-Gamma model-based filtering and prediction approach for monotonic health factors in manufacturing
Control Engineering Practice(2018)
摘要
In the context of Smart Monitoring and Fault Detection and Isolation in industrial systems, the aim of Predictive Maintenance technologies is to predict the happening of process or equipment faults. In order for a Predictive Maintenance technology to be effective, its predictions have to be both accurate and timely for taking strategic decisions on maintenance scheduling, in a cost-minimization perspective. A number of Predictive Maintenance technologies are based on the use of “health factors”, quantitative indicators associated with the equipment wear that exhibit a monotone evolution. In real industrial environment, such indicators are usually affected by measurement noise and non-uniform sampling time. In this work we present a methodology, formulated as a stochastic filtering problem, to optimally predict the evolution of the aforementioned health factors based on noisy and irregularly sampled observations. In particular, a hidden Gamma process model is proposed to capture the nonnegativity and nonnegativity of the derivative of the health factor. As such filtering problem is not amenable to a closed form solution, a numerical Monte Carlo approach based on particle filtering is here employed. An adaptive parameter identification procedure is proposed to achieve the best trade-off between promptness and low noise sensitivity. Furthermore, a methodology to identify the risk function associated to the observed equipment based on previous maintenance data is proposed. The present study is motivated and tested on a real industrial Predictive Maintenance problem in semiconductor manufacturing, with reference to a dry etching equipment.
更多查看译文
关键词
Decision support system,Gamma distribution,Industry 4.0,Monte Carlo methods,Particle filters,Predictive maintenance,Prognostic and health management,Semiconductor manufacturing
AI 理解论文
溯源树
样例
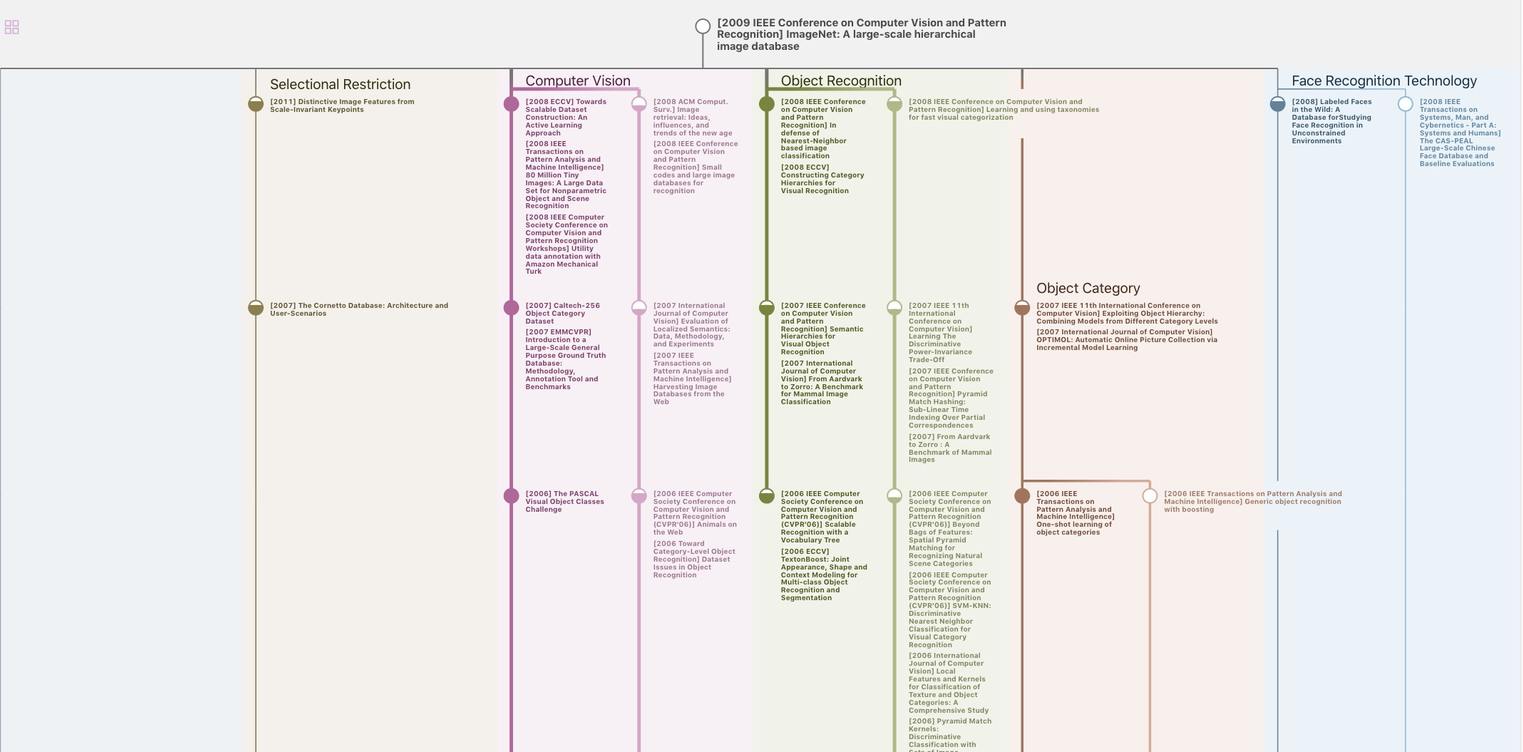
生成溯源树,研究论文发展脉络
Chat Paper
正在生成论文摘要