FedGR: Federated Learning with Gravitation Regulation for Double Imbalance Distribution.
DASFAA (1)(2023)
Abstract
Federated Learning (FL) is a well-known framework for distributed machine learning that enables mobile phones and IoT devices to build a shared machine learning model via only transmitting model parameters to preserve sensitive data. However, existing Non-IID FL methods always assume data distribution of clients are under a single imbalance scenario, which is nearly impossible in the real world. In this work, we first investigate the performance of the existing FL methods under double imbalance distribution. Then, we present a novel FL framework, called Federated Learning with Gravitation Regulation (FedGR), that can efficiently deal with the double imbalance distribution scenario. Specifically, we design an unbalanced softmax to deal with the quantity imbalance in a client by adjusting the forces of positive and negative features adaptively. Furthermore, we propose a gravitation regularizer to effectively tackle the label imbalance among clients by facilitating collaborations between clients. At the last, extensive experimental results show that FedGR outperforms state-of-the-art methods on CIFAR-10, CIFAR-100, and Fashion-MNIST real-world datasets. Our code is available at https://github.com/Guosy-wxy/FedGR .
MoreTranslated text
Key words
federated learning,double imbalance distribution,gravitation regulation,fedgr
AI Read Science
Must-Reading Tree
Example
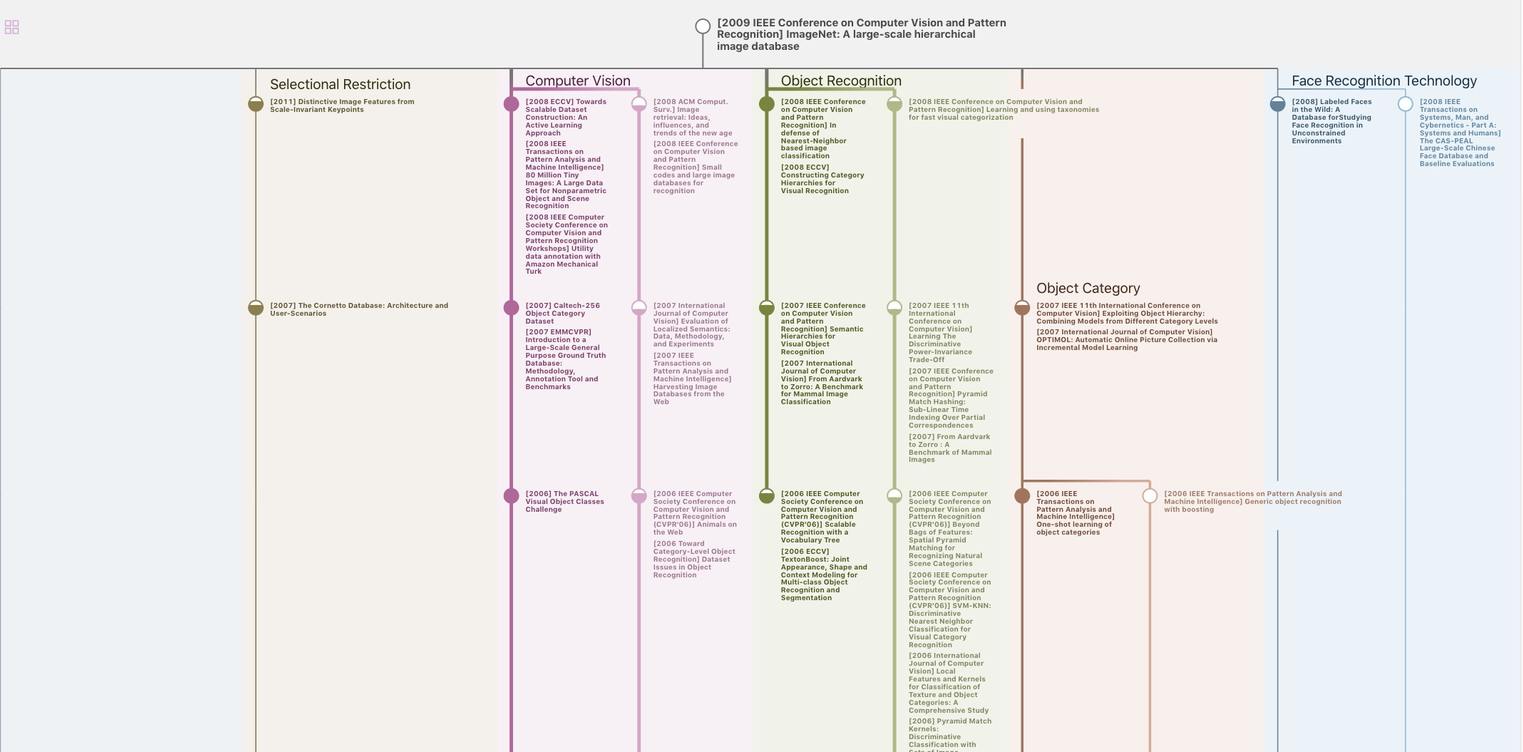
Generate MRT to find the research sequence of this paper
Chat Paper
Summary is being generated by the instructions you defined