COLLABORATIVE RECOMMENDERATION BASED ON STATISTICAL IMPLICATION RULES
Journal of Computer Science and Cybernetics(2018)
Abstract
In recent research, many approaches based on association rules have been proposed to improve the accuracy of recommender systems. These approaches are primarily based on Apriori data mining algorithm in order to generate the association rules and apply them to improving the recommendation results. However, these approaches also reveal some disadvantages of the system, such as taking a longer time for generating association rules; applying the Apriori algorithm on rating sparse matrix resulting in irrelevant information and causing poor recommendation results to target users and association rules generated primarily relying on given threshold of Support and Confidence measures leading to the focus on the majority of rules and ignoring the astonishment of rules to affect the recommendation results. In this study, we propose a new model for collaborative filtering recommender systems: The collaborative recommendation is based on statistical implication rules (IIR); Different from collaborative recommendation based on association rules (AR), the IIR predicts the items for users based on statistical implication rules generated from rating matrix and Implication intensity measures measuring the surprisingness of rules. To evaluate the effectiveness of the model, the experimental section is implemented on three real datasets to compare the results with some different effective models. The results show that the IIR has higher precision on the experimental datasets.
MoreTranslated text
Key words
recommenderation statistical implication rules
AI Read Science
Must-Reading Tree
Example
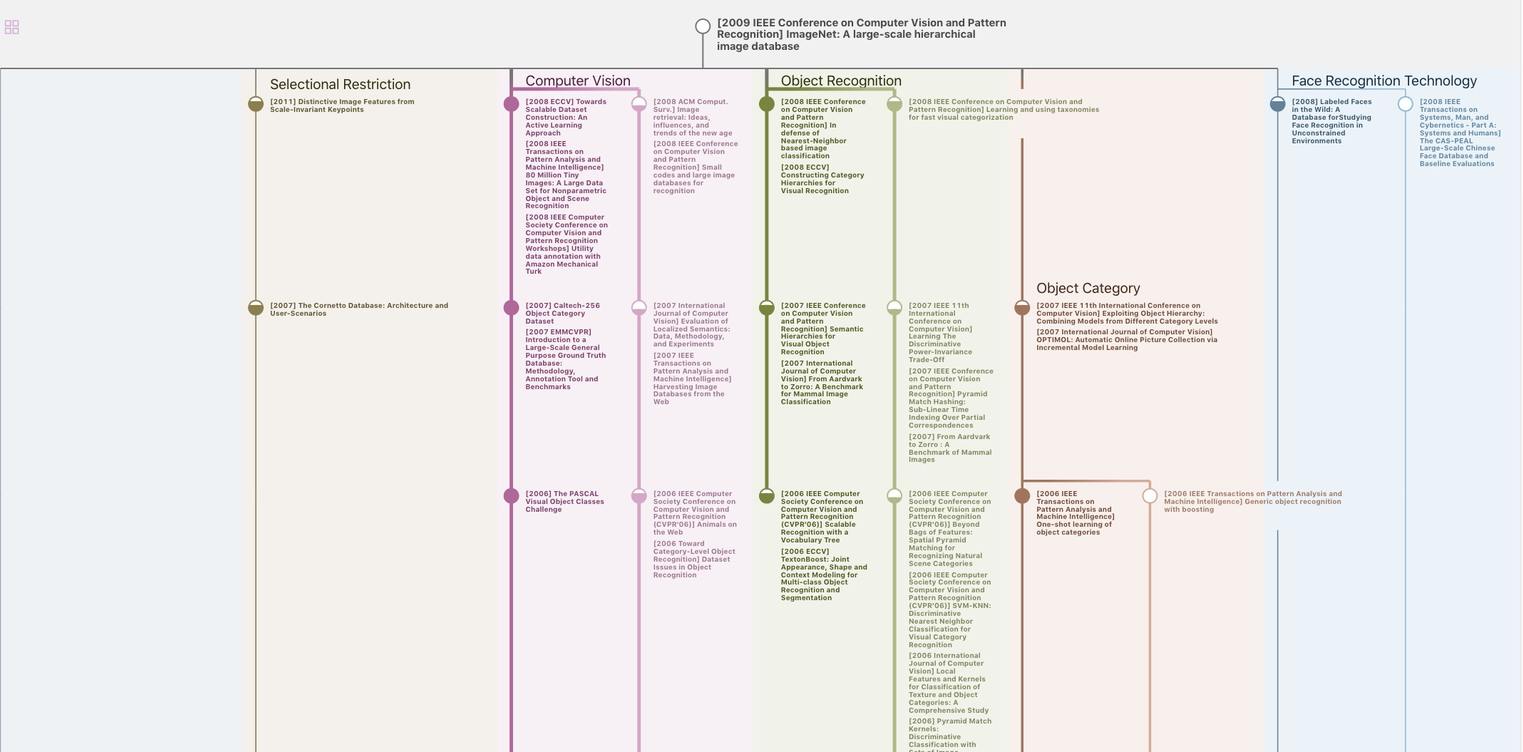
Generate MRT to find the research sequence of this paper
Chat Paper
Summary is being generated by the instructions you defined